[Update 9 December 2014]
Summaries online
The summaries of the Climate Dialogue on Climate Sensitivity and Transient Climate response are now online (see links below). We have made two versions: an extended and a shorter version.
Both versions can be downloaded as pdf documents:
Summary of the climate dialogue on climate sensitivity
Extended summary of the climate dialogue on climate sensitivity
[End update]
Climate sensitivity is at the heart of the scientific debate on anthropogenic climate change. In the fifth assessment report of the IPCC (AR5) the different lines of evidence were combined to conclude that the Equilibrium Climate Sensitivity (ECS) is likely in the range from 1.5°C to 4.5°C. Unfortunately this range has not narrowed since the first assessment report in 1990.
An important discussion is what the pros and cons are of the various methods and studies and how these should be weighed to arrive at a particular range and a ‘best estimate’. The latter was not given in AR5 because of “a lack of agreement on values across assessed lines of evidence”. Studies based on observations from the instrumental period (1850-2014) generally arrive at moderate values for ECS (and that led to a decrease of the lower bound for the likely range of climate sensitivity from 2°C in AR4 to 1.5°C in AR5). Climate models, climate change in the distant past (palaeo records) and climatological constraints generally result in (much) higher estimates for ECS.
A similar discussion applies to the Transient Climate Response (TCR) which is thought to be more policy relevant than ECS.
We are very pleased that the following three well-known contributors to the general debate on climate sensitivity have agreed to participate in this Climate Dialogue: James Annan, John Fasullo and Nic Lewis.
The introduction and guest posts can be read online below. For convenience we also provide pdf’s:
Introduction climate sensitivity and transient climate response
Guest blog James Annan
Guest blog John Fasullo
Guest blog Nic Lewis
To view the dialogue of James Annan, John Fasullo, and Nic Lewis following these blogs click here.
Climate Dialogue editorial staff
Bart Strengers, PBL
Marcel Crok, science writer
First comments on the guest blog of James Annan:
I enjoyed reading James Annan’s guest blog on climate sensitivity. There is much in his post that I agree with and I found his discussion of nonlinearities in the paleoclimate record to be particularly interesting. I also agree with his characterization of our recent work (Fasullo and Trenberth 2012) as being primarily qualitative in nature. Given the likelihood that the CMIP archives do not span the full range of parametric and structural uncertainties, it seems unlikely that a more quantitative assessment would have been justified. It is clear however, from both our work and the work of others, that various GCMs have particular difficulty in simulating even the basic features of observed variability in both clouds and radiation. Given the importance of related processes in driving the inter-model spread in sensitivity we viewed this as a sound basis for discounting such models, which as it turns out were the only models in CMIP3 with ECS below 2.7. These models were also amongst the oldest in the archive and had been shown in other work to be lacking in key respects. As discussed below, this may present an opportunity for narrowing the GCM-based range of sensitivity.
I also agree with James’ point that an adequate estimation of uncertainty has been lacking generally, though I tend to view the problem of underestimation to be more common than that of overestimation. I think this challenge speaks directly to the question posed by the editors in their introduction as to why AR5 did not choose between the different lines of evidence in forming a single “best estimate”. Doing so would have required a firmer understanding of the uncertainties inherent to each approach than is presently available. Improved assessment of these uncertainties exists as a high priority in my view and one that is achievable in the not-so-distant future.
Lastly, while James makes a good point that there is not necessarily a contradiction or tension between the various approaches if different lines of evidence provide different ranges, it is here that I have reservations. Does this necessarily mean that the likely value in nature lies at the intersection of available ranges and does this also mean that the approaches should be given equal weight? In my view, given the issues regarding uncertainty mentioned above, the answer to both of these questions is likely to be “no”. Potential improvements in so-called “20th Century” approaches include a more thorough consideration of the adequacy of any “prior”, given the rich internal variability of the climate system, and the uncertainty in both forcings and their efficacy. There is also a need to more fully consider the sensitivity of any method to observations, particularly when using ocean heat content. As we show in a paper earlier this year, the choice of an ocean heat content dataset can change the conclusions of such an analysis from being a critique of the IPCC range to being consistent with it.
For paleoclimate-based estimates, as James points out, sensitivity to nonlinearities, data problems, and uncertainty in forcing undermine any strong constraint on ECS and it is unclear (to me at least) whether progress on these fronts presents an immediate opportunity for reducing uncertainty in ECS in the near future. Lastly, I view estimates involving GCMs to be somewhat of a mixed bag. Clearly, some GCMs can be discounted based on their inability to simulate key aspects of observed climate, as discussed above. One would be hard-pressed to argue that the NCAR PCM1 and NCAR CESM1-CAM5 should be given equal weighting in estimating sensitivity. Weighting or culling model archives based on various physically-based rationales is likely to play a key role in constraining GCM estimates of sensitivity in the near future. A major, apparently unavoidable, question for this approach however is whether existing model archives sample the full range of parametric and structural uncertainty in the processes that determine sensitivity.
First comments on the guest blog of James Annan:
May I start by thanking James Annan for taking part in this discussion of climate sensitivity at Climate Dialogue. I am sure that this will be an interesting debate.
I largely agree with most of what James says about PDFs for climate sensitivity, although we have somewhat different approaches to Bayesian methodology. One point I would make is that where the PDFs have different shapes and not merely different widths, one may be more influential at low sensitivities and the other at high sensitivities. Estimated PDFs for ECS from instrumental period studies are generally both narrower and much more skewed (with long upper tails) than those from paleoclimate studies. When the evidence represented in these two types of PDFs is combined, in general the instrumental period PDF will largely determine the lower bound of the resulting uncertainty range but the paleoclimate PDF may have a significant influence on its upper bound. That is because, in general, whichever of the PDFs is varying more rapidly at a particular ECS level will have more influence on the combined studies’ PDF at that point. Instrumental study PDFs for ECS generally have a sharp decline at low ECS values but, with their long upper tails, a very slow decline at high ECS values.
I think that the reasons AR5 downweights various approaches differs between them. For paleoclimate studies, on my reading the AR5 scientists took the view that the uncertainties were generally underestimated, not only because of the difficulty in estimating changes in forcing and temperature but also, importantly, because climate sensitivity in the current state of the climate system might be significantly different from that when it was in substantially different states. There, widening the uncertainty range (effectively flattening the PDF) seems reasonable. For studies involving short term changes, some of which have non-overlapping uncertainty ranges, the concern seems to me more that it is unclear whether the estimates they arrive at really represent ECS, or something different. The case for simply disregarding all such estimates as unreliable is stronger there. The concern is more with the merits of such approaches than with individual studies.
I agree with James’ observation that the transient pattern of warming is likely to be a little different from the equilibrium result, which may result in the ECS estimates from instrumental period warming studies involving only global or hemispherically-resolving models (which usually represent effective climate sensitivity) differing a little from equilibrium climate sensitivity. However, the Armour et al (2013) paper that James cited in that connection was based on a particular GCM that has a latitudinal pattern of climate feedbacks very different from that of most GCMs.
James and I seem to have similar views as to studies based on ensembles of simulations involving varying the parameters of a GCM being of little use. And whilst it is interesting that climate models with higher sensitivities may be better at simulating certain aspects of the climate system than others, it does not follow that their sensitivities must be realistic.
Regarding paleoclimate study ECS estimates, I concur with the conclusions reached in AR5. So, overall, this line of evidence indicates that there is only about a 10% probability of ECS being below 1°C and a 10% chance of it being above 6°C. I think the uncertainties are simply too great to support the narrower ~2–4.5°C range mentioned by James, and I wouldn’t support using that narrower range as a prior in a Bayesian analysis.
Reference
Armour, K. C., Bitz, C. M., & Roe, G. H. (2013). Time-Varying Climate Sensitivity from Regional Feedbacks. Journal of Climate, 26, 4518–4534. doi:10.1175/JCLI-D-12-00544.1
First comments on the guest blog of John Fasullo:
John Fasullo focusses on the change lower limit of the IPCC AR5 “likely” range from 2 (in AR4) to 1.5 (in AR5), arguing that although it was understandable, it was wrong on the basis that models can reproduce periods of little warming. While I don’t presume to know what was going on in the IPCC authors’ esteemed minds, I believe it’s far preferable to consider the climate sensitivity estimation on the merits of the available literature rather than considering the previous IPCC AR4 estimate (and/or the GCM model range) as some sort of prior or null hypothesis to only be changed if and when the observational data become overwhelming. Whether we can still argue that the recent observed global mean temperature time series is consistent with the GCM ensemble (at some arbitrary level of confidence) is rather beside the point. The observed time series is indisputably close to the lower end of the range, and any reasonable estimate had better take that into account.
Some recent estimates, like Stott et al (2013), look beyond the global or hemispheric mean temperature change, and consider the full spatial pattern of response to different forcings. Fasullo’s arguments don’t appear to apply to this sort of detection and attribution approach at all.
Reference
Stott, P., Good, P., Jones, G., Gillett, N., & Hawkins, E. (2013). The upper end of climate model temperature projections is inconsistent with past warming. Environmental Research Letters, 8(1), 014024. doi:10.1088/1748-9326/8/1/014024
First comments on the guest blog of John Fasullo:
May I start by thanking John Fasullo for taking part in this discussion of climate sensitivity at Climate Dialogue. I can see from the title of his guest blog that we are in for an interesting debate.
I have just a few comments on John’s opening section The Challenge. In relation to climatological constraint approaches, my analysis – summarised in my guest blog – of the Sexton et al (2011) and Harris et al (2013) studies featured in AR5 (only the TCR estimate from the latter being shown) establishes that perturbing GCM parameters does not provide a valid way to estimate ECS, at least for the HadCM3/SM3 model that has been widely used for this purpose. I note that James Annan no longer considers such methods to be of much use.
Whether use of CMIP ensembles and ‘emergent constraints’ will provide much of a constraint on climate sensitivity is an open question. At present, supposed ‘emergent constraints’ seem primarily to tell one which models are good or bad at various things. For instance, Cai et al (2014) showed that 20 out of 40 CMIP3 and CMIP5 models are able to reproduce the high rainfall skewness and high rainfall over the Nino3 region, whilst Sherwood et al (2014) shows that 7 CMIP3 and CMIP5 models (5 of which were included in Cai’s analysis) have a lower-tropospheric mixing index falling within the observational (primarily model reanalyses, in fact) uncertainty ranges – and that those models have high climate sensitivities. Unfortunately, no model satisfies both Cai’s and Sherwood’s tests. A logical conclusion is that at present models are not good enough to rely on the climate sensitivities of any of them.
John says that to some extent the distinctions between ECS estimation methods are artificial. But although there are elements in common, there are fundamental differences. As he says, all GCMs have used the instrumental record to select model parameter values that produce plausible climates. However, as an experienced team of climate modellers has written (Forest, Stone & Sokolov, 2008), many combinations of model parameters can produce good simulations of the current climate but substantially different climate sensitivities. Whilst observations inform model development, the resulting model ECS values are only weakly constrained by those observations.
By contrast, in a properly designed observationally-based study, the best estimate for ECS is completely determined by the actual observations, as is normal in scientific experiments. To the extent that the model, simple or complex, used to relate those observations to ECS is inaccurate, or the observations themselves are, then so will the ECS estimate be. But, in any event, the ECS estimate will be far more closely related to observations than are GCM ECS values.
Moving on to the need for physical understanding, I certainly agree about the desirability of a physically-based perspective. However, I fear that the climate system may be too complex and current understanding of it too incomplete for strong constraints on ECS or TCR to be achieved in the near future from just narrowing constraints on individual feedbacks. Certainly, I doubt that we are close to that point yet. Attempts have been made to constrain cloud feedback, where uncertainties are greatest, from observable aspects of present-day clouds. But AR5 (Section 7.2.5.7) judges these a failure to date, concluding that “there is no evidence of a robust link between any of the noted observables and the global feedback”.
At present, there seems little doubt that energy-budget based approaches are the most robust way of estimating ECS and TCR. They involve a very simple physical model – directly based on conservation of energy – with relatively few assumptions, and they in effect measure the overall feedback of the climate system using the longest and least uncertain observational records available, of surface temperatures. Their main drawback is the large uncertainty as to changes in total radiative forcing, resulting principally from uncertainty in aerosol forcing.
Better constraining aerosol forcing is the key to narrowing uncertainty in all ECS and TCR estimates based on observed multidecadal warming during the instrumental period, not only energy budget estimates. But it is encouraging that all instrumental period warming based observational studies that have no evident serious flaws now arrive at much the same ECS estimates, in the 1.5–2.0°C range. As well as simple energy budget approaches using the AR5 best estimates for aerosol and other forcings, that includes several studies which form their own estimates of aerosol forcing using suitable data – more than just global temperature – and relatively simple (but hemispherically-resolving) or intermediate complexity models.
My readings of the conclusions in Chapters 10 and 12 of AR5 WG1 is that the scientists involved shared my view that higher confidence should be placed on studies based on warming over the instrumental period than on other observational approaches.
John raises the issue of varying definitions of ECS. IPCC assessment reports treat equilibrium climate sensitivity as relating to the response of global mean surface temperature (GMST) to a doubling of atmospheric CO₂ concentration once the atmosphere and ocean have reached equilibrium, but without allowing for slow adjustments by such components as ice sheets and vegetation. The term Earth system sensitivity (ESS) is used for the equilibrium response taking into account such adjustments.
In practice, what many observationally-based studies estimate is Effective climate sensitivity, a measure of the strengths of climate feedbacks at a particular time, evaluated from model output or observations for evolving non-equilibrium conditions. Effective climate sensitivity does take changes in both the upper and the deep ocean into account, as well as changes in the cryosphere other than ice sheets, but in some GCMs it is a bit lower than equilibrium climate sensitivity. AR5 concludes (Section 12.5.3) that the climate sensitivity measuring the climate feedbacks of the Earth system today “may be slightly different from the sensitivity of the Earth in a much warmer state on time scales of millennia”. But the terms effective climate sensitivity and equilibrium climate sensitivity are largely used synonymously in AR5. From a practical point of view, changes over the next century will in any event be more closely related to TCR than to either variant of ECS, let alone to ESS.
John raises the difficulty of finding the appropriate statistical “prior” for the free parameters of a model. That is far more of a problem with a GCM than with a simple model, because of the much higher dimensionality of the parameter space – a GCM has hundreds of parameters. Even assuming some combinations of parameter values will produce a realistic simulation of the climate, that may be a tiny and almost impossible-to-find subset of possible parameter combinations. The number of degrees of freedom available in relevant observations is limited, bearing in mind the high spatiotemporal correlations in the climate system and the large uncertainty in most observations (from internal variability as well as measurement error). It is therefore more practicable to constrain a smaller number of parameters using observations.
Where the intent is to allow the observations alone to inform parameter estimation (objective estimation, as is usual for scientific experiments), there are well established methods of finding the appropriate statistical prior. See Jewson, Rowlands and Allen (2009) for how to apply these in the context of a climate model. Or non-Bayesian methods such as modified or simple profile likelihood, which do not involve explicitly selecting a prior, can be used. Incorporating subjective beliefs or other non-observational information about parameter values is more complex. Doing so may also not be wise. A parameter value thought to be physically unlikely may be necessary in order to compensate for an erroneous or incomplete representation of the climate process(es) involved.
Hiatus
I believe John’s views of the impact of “hiatus” in global surface warming over the last circa 15 years on estimation of ECS are seriously mistaken. He starts by claiming that, based on simple models, the hypothesis that the hiatus argues for a reduction in the lower bound of the range for ECS was found sufficiently compelling that IPCC AR5 reduced the lower bound of its likely range for ECS. John cites Chapter 12 in that regard. But Box 12.2, which covers equilibrium climate sensitivity and transient climate response, does not even mention the slowdown in warming this century. It says (my emphasis):
Based on the combined evidence from observed climate change including the observed 20th century warming, climate models, feed¬back analysis and paleoclimate, ECS is likely in the range 1.5°C to 4.5°C with high confidence.
and goes on to say:
“The lower limit of the likely range of 1.5°C is less than the lower limit of 2°C in AR4. This change reflects the evidence from new studies of observed temperature change, using the extended records in atmosphere and ocean. These studies suggest a best fit to the observed surface and ocean warming for ECS values in the lower part of the likely range.”
John’s argument that observationally-based estimates pointing to ECS being lower than previous consensus estimates are strongly influenced by the hiatus seems quite widespread. A recent peer-reviewed paper (Rogelj et al, 2014) cites in that connection four studies, including the only three instrumental-period warming based observational ECS estimates featured in Figure 1 of Box 12.2 that I conclude are sound. It first discusses the old AR4 2–4.5°C likely range for ECS, saying:
“Some newer studies have confirmed that range (Andrews et al 2012, Rohling et al 2012), but others have raised the possibility that ECS may be either lower (Schmittner et al 2011, Aldrin et al 2012, Lewis 2013, Otto et al 2013) or higher (Fasullo and Trenberth 2012, Sherwood et al 2014) than previously thought.”
Rogelj et al then conclude (my emphasis):
“A critical look at the various lines of evidence shows that those pointing to the lower end are sensitive to the particular realization of natural climate variability (Huber et al 2014). As a consequence, their results are strongly influenced by the low increase in observed warming during the past decade (about 0.05 C/decade in the 1998–2012 period compared to about 0.12 C/decade from 1951 to 2012, see IPCC 2013)… ”
It is clear from the context that the claim that “their results are strongly influenced by the low increase in observed warming during the past decade” refers back to the results of the Schmittner et al 2011, Aldrin et al 2012, Lewis 2013 and Otto et al 2013 studies. But the claim is completely incorrect in relation to all four studies:
• Schmittner et al 2011 estimated ECS from temperature reconstructions of the Last Glacial Maximum.
• Aldrin et al 2012 used data ending in 2007 for its main results ECS estimate but also presented an alternative estimate based on data ending in 2000. The median ECS estimate using data only up to 2000 was lower, not higher, than the main one using data to 2007. Moreover, their updated ECS estimate using data up to 2010, published in Figure 10.20b of AR5, had a higher median than that using data to 2007.
• The Otto et al 2013 median ECS estimate using 2000s data was the highest of all its ECS estimates; the ECS estimates using data from the 1970s, 1980s or 1990s were all lower.
• Lewis 2013 used data ending in August 2001.
Evidence for climate sensitivity being lower than previous consensus views has indeed been piling up, but that is not because of the hiatus. On the other hand, I agree that any claim that global warming has stopped is nonsense. As John says, a planetary radiative imbalance persists, as shown by ocean heat uptake data. However, the level of imbalance appears to be only about 0.5 W/m², and if anything to have declined slightly since the turn of the century.
I believe that the suggestion John refers to (in Schmidt et al, 2014), that reductions in total forcing (ERF) are driving the hiatus, is wide of the mark. That paper claims CMIP5 forcings, based on the historical estimates to 2000 or 2005 and representative concentration pathway (RCP) scenarios thereafter, have been biased high since 1998. The largest claimed bias is in volcanic forcing, which Schmidt et al say averaged -0.3 W/m² from over 2006–11, almost treble AR5’s best estimate, and nearly twice what their cited source seems to indicate. Their assumption that CMIP5 models all had zero volcanic forcing post 2000 is also dubious; the RCP forcings dataset has volcanic forcing averaging -0.13 /m² over that period. Their assumption that increases in nitrate aerosols affected aerosol forcing by -0.1 W/m² since the late 1990s has little support in the cited source. Their application of a multiplier of two to differences in estimated solar forcing has no support in AR5. My conclusion that the Schmidt et al study is biased and almost certainly wrong is supported by statements in Box 9.2 of AR5. It says there that over 1998–2011 the CMIP5 ensemble-mean ERF trend is actually slightly lower than the AR5 best-estimate ERF trend, and that “there are no apparent incorrect or missing global mean forcings in the CMIP5 models over the last 15 years that could explain the model–observations difference during the warming hiatus”.
I concur with John’s view that natural internal climate system variability has probably made a substantial contribution to the hiatus. But it probably made a significant contribution in the opposite direction to the fast warming over the previous quarter century, due principally to the Atlantic Multidecadal Oscillation then being in its warming phase.
CAM5 model
I am not surprised that the NCAR CESM1-CAM5 model matched global actual warming reasonably well from the 1920s until the early 2000s despite having a high ECS of 4.1°C and (according to AR5) a TCR of 2.3°C. The CESM1-CAM5.1 model’s aerosol forcing was diagnosed (Shindell et al, 2013) as strengthening by -0.7 W/m² more from 1850 to 2000 than per AR5’s best estimate. If the model’s other forcings were in line with AR5’s estimates, its increase in total ERF over 1850–2000 would have been only 64% of the AR5 best estimate. That much ERF change and a TCR of 2.3°C would have produced the same warming as a model with a TCR of 1.48°C in which ERF had changed in line with AR5’s best estimate.
As John says, the ensemble mean in his Figure 2 suggests that, due to forcing, certain decades are predisposed to a reduced rate of surface warming. But that is hardly surprising: decades having a major volcanic eruption near their start or end will tend to have respectively high or low trends, whereas others will tend to be in between. So, due to the 1991 Mount Pinatubo eruption, decades ending in the early 1990s show low trends whilst those ending around 2000 show high trends. Ensemble mean trends for decades ending in the last few years, whilst therefore lower than those for decades ending around 2000, are higher than for almost any other decades. I would challenge John’s view that 2010–2012 represented exceptional La Niña conditions. According to the MEI Index, it had only the 10th lowest 3-year index average since 1952. As 2012 had a positive index value, the average for 2010-11 is perhaps a fairer test. That had the 7th lowest 2-year average since 1951, still hardly exceptional: it was under half as negative as for 1955–56.
I endorse John’s call for well-understood, well-calibrated, global-scale observations of the energy and water cycles, but would emphasise the need for better observations of clouds and their interactions with aerosols. In my view, too much of the available resources were put into model development in the past and not enough into observations. Unfortunately, for many variables only a long record without gaps is adequate. The ARGO network has indeed greatly improved estimates of ocean heat content (OHC), but what a shame it has only been operating for a decade. Modern ocean “reanalysis” methods are no substitute for good observations. The modern ORAS4 reanalysis is clearly model-dominated in the pre-Argo period: the huge declines in 0-300 m and 0-700 m OHC shown in Balmaseda et al (2013) after the 1991 Mount Pinatubo eruption are absent in the observational datasets.
References
Balmaseda, M, K Trenberth and E Kallen, Distinctive climate signals in reanalysis of global ocean heat content. Geophys Res Lett, 40, 1–6, doi:10.1002/grl.50382
Cai, W et al, 2014. Increasing frequency of extreme El Niño events due to greenhouse warming. Nature Climate Change 4, 111–116
Forest, C.E., P.H. Stone, and A.P. Sokolov, 2008. Constraining climate model parameters fromobserved 20th century changes. Tellus, 60A, 911–920
Jewson, S., D. Rowlands and M. Allen, 2009: A new method for making objective probabilistic climate forecasts from numerical climate models based on Jeffreys’ Prior. arXiv:0908.4207v1 [physics.ao-ph].
Rogelj, Meinshausen, Sedlacek and Knutti, 2014. Implications of potentially lower climate sensitivity on climate projections and policy. Environ Res Lett 9 031003 (7pp)
Sherwood, SC, S Bony & J-L Dufresne, 2014. Spread in model climate sensitivity traced to atmospheric convective mixing. Nature 505, 37–42
Shindell, D. T. et al, 2013. Radiative forcing in the ACCMIP historical and future climate simulations. Atmos. Chem. Phys. 13, 2939–2974
First comments on the guest blog of Nic Lewis:
Nic Lewis appears to be arguing primarily on the basis that all work on climate sensitivity is wrong, except his own, and one other team who gets similar results. In reality, all research has limitations, uncertainties and assumptions built in. I certainly agree that estimates based primarily on energy balance considerations (as his are) are important and it’s a useful approach to take, but these estimates are not as unimpeachable or model-free as he claims. Rather, they are based on a highly simplified model that imperfectly represents the climate system.
For instance, one well-known limitation of such models that effective climate sensitivity is not truly a constant parameter of the earth system, but changes through time depending on the transient response to radiative forcing. This introduces an extra source of uncertainty (which is probably a negative bias) into estimates based on this approach.
I’m disappointed in this response. Lewis addressed your objections, particularly with regard to effective vs equilibrium climate sensitivity.
First comments on the guest blog of Nic Lewis:
I find the statistical approach promoted by Nic Lewis (and others preceding him) to be a compelling and potentially promising contribution in the effort to better understand and constrain climate sensitivity. The approach provides an elegant and powerful means for understanding the collective, gross-scale behavior of the climate system using a simple statistical framework, if implemented appropriately. However I also have reservations regarding the method in its current form. It has yet to be widely scrutinized in a physically realistic framework, has multiple untested assumptions, and is likely to have considerable sensitivity to a various details surrounding its implementation.
While I am optimistic that many of these issues can be addressed in future work, my confidence in the robustness of the sensitivity estimates and associated bounds of uncertainty currently promoted by Nic is low, given these issues. From my point of view, some of the key questions remaining to be addressed include:
• What is the method’s sensitivity to internal variability and uncertain forcings (and their combined direct / indirect effects and efficacy), particularly in situations in which their variability is not orthogonal?
• How long of a record is required to obtain a robust estimate of sensitivity? Is it asking too much of a purely statistical approach to distill the combined effects of uncertain and variable forcings from internal variability using a finite data record?
• In what contexts can instrumental estimates be viewed as more reliable than other estimates and in what situations are they particularly vulnerable to error?
• How can a more process-relevant statistical approach be developed that takes better advantage of the available data record? How do the various trade-offs between dataset uncertainty and relevance to the planetary imbalance, climate change, and feedbacks play out in such an effort?
While I could go into details addressing the many points made and studies cited by Nic in his post, in order to avoid repeating the points made in my original post and to promote a broader discussion without getting lost in the weeds, I think it might be useful to focus on a few key overarching issues on which there seems to be fundamental disagreement. From my perspective:
1) All estimates of climate sensitivity require a model. It is the complexity of the underlying model that varies across methods. Attempts to isolate the effects of CO2 on the temperature record are inherently an exercise in attribution and the use of a model is therefore unavoidable.
2) Given (1), it is a misnomer to present 20th Century instrumental approaches as being “observational estimates”. It is therefore also inappropriate to present them as being superior to other approaches based on such an assertion. Moreover, as discussed in my original post, the distinction between the approaches is somewhat contrived. In fact, GCM’s incorporate several orders of magnitude more observational information in their development and testing than do the typical “instrumental” approaches described by the editors (more on this below).
3) All methods have their weaknesses. While Nic has done a good job pointing out issues with other methods, he underestimates those in his own and in doing so is at odds with the originators of such techniques (e.g. Forster et al. 2013). Without a physical understanding of the climate system, based on robust observations of key processes, which can likely be promoted in instances by statistical approaches, there cannot be high confidence in climate projections. Statistical techniques, particularly when trained over a finite, complex, and uncertain data record in which forcings are also considerably uncertain, are no panacea to the fundamental challenge of physical uncertainty.
The good news, in my view, is that at least some of the questions I’ve posed above are readily testable and our understanding of a range of statistical approaches can be significantly improved in the near future. For instance, the NCAR Large Ensemble now provides the opportunity to apply assessments using Bayesian priors to a physical framework that has been demonstrated to be quite skillful in reproducing many of the observed modes of low frequency variability. The capability of such methods to estimate the known climate sensitivity of the CESM-CAM5 in the midst of realistic internal variability and temporally finite records is quantifiable. In fact, colleagues and I at NCAR are currently collaborating in an effort to do just this. Our initial perspective is that such methods are likely to be first-order sensitive to these effects and that uncertainty assessments such as that provided by Schwartz (2012) are probably much more reasonable than others claiming to provide a strong constraint on models. Our work is ongoing, and as such any definitive conclusion would be premature, but please, stay tuned.
In closing, it is only reasonable to welcome a broad array of approaches in assessing climate sensitivity. Yet, it is also clear that not all approaches have received equal scrutiny and that some perspectives on them have received even less scrutiny. Ultimately it is the thorough scrutiny of all models, whether complex or simple, and methods that will be instrumental in reducing uncertainty. The lure of doing so using purely statistical approaches is appealing, but in my view, is fool’s gold. In the early days of modeling, a time at which global observations of key fields were lacking, I would have advocated for the supremacy of such an approach over poorly constrained GCMs. Yet as I write this commentary, and as I work on a parallel effort to assess decadal variability in GCMs, I cannot help but be struck by a clear irony. The dataset I am using is the pioneering NOAA AVHRR OLR dataset, which completes its fourth decade of reporting next month, beginning in June of 1974. Despite its various blemishes, the achievement in constructing this record is both remarkable and unprecedented, and lessons learned have contributed to numerous follow-on efforts (e.g. CALIPSO, CERES, CLOUDSAT, ERBE, GPCP, GRACE, ISCCP, QUIKSCAT, SSM/I, TOPEX, TRMM, …). Given this era of such remarkable observations, accompanied by similar achievements across a realm of disciplines (e.g. ocean and atmospheric observations, operational models, reanalysis methods, supercomputing, …), I cannot help but be struck by the fact that there are those advocating for assessing climate solely with statistical approaches using simple models that capture little of the climate system’s physical complexity, trained on a limited subset of questionably relevant surface observations, and based on largely untested physical assumptions. It is an argument for which I find little support.
References:
Forster, P. M., T. Andrews, P. Good, J. M. Gregory, L. S. Jackson, and M. Zelinka (2013), Evaluating adjusted forcing and model spread for historical and future scenarios in the CMIP5 generation of climate models, J. Geophys. Res. Atmos., 118, 1139–1150, doi:10.1002/jgrd.50174.
Schwartz, S. E. (2012). Determination of Earth’s transient and equilibrium climate sensitivities from observations over the twentieth century: strong dependence on assumed forcing. Surveys in Geophysics, 33(3-4), 745-777.
Dear John, Nic and James,
I propose to start the discussion with the first question raised in our introduction:
What are the pros and cons of the different lines of evidence?
After studying your guest blogs and the first responses above I conclude there is a major difference in opinion on the pros and cons (and thus the importance or weight) of the first line of evidence, i.e. studies based on observations from the instrumental period that generally arrive at lower values of ECS.
Below I tried to summarize the pros and cons that I found in your contributions so far on this first line of evidence (the references can be found in the guest blogs). Mainly based on his pros, and the rejection of other lines of evidence, Nic arrives at a likely range for ECS that is much lower than reported by the IPCC: 1.2 – 3.0 and a best estimate of 1.7. According to James, the paleoclimate evidence provides ‘reasonable grounds for expecting a figure around to the IPCC canonical range’, which is 1.5 – 4.5, but he adds that ’the recent transient warming (combined with ocean heat uptake and our knowledge of climate forcings) points towards a “moderate” value for the ECS’ between 2.0 to 3.0. John made the point that ’the evidence accumulated in recent years’ justifies a lower bound of the likely range as in AR4, i.e. 2.0 instead of 1.5 in AR5. He did not provide a likely upper bound or a beste estimate yet.
Nic Lewis on observations from the instrumental period:
Pros
1. Anthropogenic signal has risen clear of the noise arising from internal variability and measurement/forcing uncertainty and therefore provide narrower ranges than those from other studies.
2. In a properly designed observationally-based study, the best estimate for ECS is completely determined by the actual observations, as is normal in scientific experiments. In any event, the ECS estimate will be far more closely related to observations than are GCM ECS values.
3. The only studies on observations from the instrumental period that should be regarded as both reliable and able to usefully constrain ECS are Aldrin (2012), Ring (2012), Lewis (2013) and Otto (2013), in accordance with the conclusions of AR5.
4. The robust ‘energy budget’ method of estimating ECS (and TCR) gives results in line with these studies.
5. Finding the appropriate “prior” is far more of a problem with a GCM than with a simple model, because of the much higher dimensionality of the parameter space.
6. Chapters 10 and 12 of AR5 WG1 share my view that higher confidence should be placed on studies based on warming over the instrumental period than on other observational approaches.
7. Annan is right that effective CS is slightly different from ECS, but these terms are largely used synonymously in AR5; Annan cites Armour (2013) but that is based on a GCM that has a latitudinal pattern of climate feedbacks very different from that of most GCMs.
8. Observational evidence is preferable to that from models, as understanding of various important climate processes and the ability to model them properly is currently limited.
Cons
1. Large uncertainty as to changes in total radiative forcing, resulting principally from uncertainty in aerosol forcing.
2. Lindzen & Choi (2011) and Murphy (2009) depend on short-term changes and are deprecated by AR5.
3. Studies using global mean temperature data to estimate aerosol forcing and ECS together are useless. Northern Hemisphere and Southern Hemisphere must be separated.
4. Observational studies with uniform priors greatly inflate the upper uncertainty bounds for ECS.
5. Observational studies using expert priors produce ECS estimates that reflect the prior, with the observational data having limited influence.
James Annan on observations from the instrumental period:
Pros
1. Global warming points to an ECS at the low end of the IPCC range due to better quality and quantity of data and better understanding of aerosol effects (Aldrin et al 2012, Ring et al 2012, Otto et al 2013).
2. Lewis’ estimates based primarily on energy balance considerations is a useful approach to take.
Cons
1. These studies assume an idealised low-dimensional and linear system in which the surface temperature can be adequately represented by global or perhaps hemispheric averages. In reality the transient pattern of warming (or the effective CS) is different from the equilibrium result, which complicates the relationship between observed and future (equilibrium) warming (Armour, 2014).
2. Lewis’ four preferred observational studies are not as unimpeachable or model-free as he claims but based on a highly simplified model that imperfectly represents the climate system.
3. Effective CS is not a constant parameter of the earth system, but changes through time depending on the transient response to radiative forcing. This introduces an extra source of uncertainty (which is probably a negative bias) into estimates based on Lewis’ approach.
John Fasullo on observations from the instrumental period:
No Pros given yet.
Cons
1. These studies are severely limited by the assumptions on which they’re based, the absence of a unique “correct” prior, and the sensitivity to uncertainties in observations and forcing (Trenberth 2013).
2. Uncertainty in observations and the need to disentangle the response of the system to CO2 from the convoluting influences of internal variability and responses to other forcings (aerosols, solar, etc) entails considerable uncertainty in ECS (Schwartz, 2012) and thus: 1) the use of a model is unavoidable, 2) it is a misnomer to present 20th Century instrumental approaches as being “observational estimates”.
3. Limited warming during the hiatus does not point at a low ECS but has been driven by the vertical redistribution of heat in the ocean, confirmed by persistence in the rate of thermal expansion since 1993 (Cazenave et al 2014).
4. Recent observations have reinforced the likelihood that the current hiatus is consistent with such simulated periods.
5. Attempts to isolate the effects of CO2 on the temperature record are inherently an exercise in attribution and the use of a model is therefore unavoidable.
6. Lewis underestimates the weaknesses and in doing so is at odds with the originators of this method (e.g. Forster et al. 2013).
7. Statistical techniques, particularly when trained over a finite, complex, and uncertain data record in which forcings are also considerably uncertain, are no panacea to the fundamental challenge of physical uncertainty.
8. Assessing ECS solely with statistical approaches using simple models that capture little of the climate system’s physical complexity, trained on a limited subset of questionably relevant surface observations, and based on largely untested physical assumptions is impossible.
I consider it very interesting to focus first on the second con of James and the related second con of John:
Lewis’ four preferred observationally-based studies are not as unimpeachable or model-free as he claims but based on a highly simplified model that imperfectly represents the climate system.
And:
Uncertainty in observations and the need to disentangle the response of the system to CO2 from the convoluting influences of internal variability and responses to other forcings (aerosols, solar, etc) entails considerable uncertainty in ECS (Schwartz, 2012) and thus: 1) the use of a model is unavoidable, 2) it is a misnomer to present 20th Century instrumental approaches as being “observational estimates”.
Lewis fully disagrees since he claims that:
In a properly designed observationally-based study, the best estimate for ECS is completely determined by the actual observations, as is normal in scientific experiments. In any event, the ECS estimate will be far more closely related to observations than are GCM ECS values.
I think it would be valuable to discuss this difference in opinion in more detail.
Bart has done an excellent job in summarising the issues, and in fact I’m not sure that I have a lot to add to my previous comments. I do think Nic Lewis over-states the case for the so-called “observational estimates” in a number of ways. Clearly, even these estimates rely on models of the climate system, which are so simple and linear (and thus certainly imperfect) that they may not be recognised as such.
Further issues arise with his methods, though in my opinion these are mostly issues of semantics and interpretation that do not substantially affect the numerical results. (For those who are interested in the details, his use of automatic approach based on Jeffreys prior has substantial problems at least in principle, though any reasonable subjective approach will generate similar answers in this case.) The claim that “observations alone” can ever be used to generate a useful probabilistic estimate is obviously seductive, but sadly incorrect. Thus, his results are not the peerless answer that he claims.
Nevertheless, they are a useful indication of the value of the equilibrium sensitivity, and I would agree that these approaches tend to be the most reliable in that the underlying assumptions (and input data) are generally quite good. A caveat arising from very recent research is the matter of forcing efficacy raised by Shindell and explored by Kummer and Dessler. I would like to see this new literature reconciled with previous research, especially that relating to detection and attribution, which already implicitly includes an (a priori unknown) efficacy factor in its estimation methods – and which, I believe, generally reaches contrary conclusions.
A quick response to James Annan’s recent comment.
First, I agree that observationally-based climate sensitivity estimates also involve use of climate models. I said so in my guest blog. I did not claim that observations alone can be used to generate a useful estimate of ECS. But, unlike estimates based directly on GCMs, or on constraining GCMs, observationally-based ECS estimates do not generally depend to first order on the ECS values of the climate models involved.
Second, just to clarify, my point summarised by Bart as “In a properly designed observationally-based study, the best estimate for ECS is completely determined by the actual observations” relates to the ECS estimation once the details of the method and the model used have been fixed.
I’m not sure exactly what James refers to when he writes “his results are not the peerless answer that he claims”, but if it is to the results in my objective Bayesian 2013 Journal of Climate paper (available here then I do not claim that they are perfect. (They are in a sense peerless, but only in that everyone else carrying out explicitly Bayesian multidimensional climate sensitivity studies seems to have used a subjective approach.)
I agree that Bart’s summary of the issues is excellent and am glad that we have broad agreement that the use of some model is intrinsic to all approaches to estimate ECS. The quality of the estimate thus hinges critically on the quality of the model. Like Bart, I also find this perspective to be at odds with the statement that observational-based estimates are “completely determined by the actual observations”.
I would also like to add that I do find “pros” for approaches attempting to estimate ECS from the observational record, per my comments on Nic’s piece. I genuinely do think that they have the potential to play an important role in constraining ECS once their strengths and weaknesses are broadly understood. I also suggest a means for doing so – namely exploring such methods in a framework that is tightly constrained. As mentioned, using a model whose sensitivity is known and whose variability is thoroughly vetted provides such an opportunity. A model ensemble can be generated to encompass the full range of uncertainty arising from forcing (including a consideration of direct/indirect effects and efficacy) and internal variability, and these methods can be applied over records of varying length and phases of internal modes to evaluate their robustness. To my knowledge, such an examination has yet to be done. Am I perhaps overlooking one? As such, I see no solid basis for rejecting an approximate range for ECS of 2.0 to 4.5 with a best estimate of about 3.4. It is noteworthy as well that an additional “pro” of these methods, once they are understood, is that they hold the promise of saving the countless CPU-hours of computation involved in estimating ECS from a fully coupled simulation (as a complement to the Gregory method).
Lastly, I would like to reiterate my position that I do not believe any method for estimating ECS should be rejected outright. The challenge as I see it is how to understand the apparent divergence in results provided by each in terms of their respective strengths and weaknesses. From my point of view, Shindell (2014) and Kummer and Dessler (2014) provide a viable rationale for reconciling such disagreements. Is there any basis for rejecting them outright?
Hello everybody. My usual nick is: Antonio (AKA “Un físico”) but from now on and in here I will use the nick “Antonio AKA Un fisico”. Well, going to the point of this post. After my analysis in: https://docs.google.com/file/d/0B4r_7eooq1u2TWRnRVhwSnNLc0k
(see subsection 3.1, pgs. 6&7) anyone can easily conclude that IPCC’s ECS is an invented value: that it is science fiction.
Nic Lewis is wrong when he says: “Regarding paleoclimate study ECS estimates, I concur with the conclusions reached in AR5. So, overall, this line of evidence indicates that there is only about a 10% probability of ECS being below 1°C and a 10% chance of it being above 6°C”.
So let’s dialogue about IPCC’s paleoclimate stimations of ECS. Please Nic, read my pg.7. Spetially the paragraph: “Error bars (see, for example, WGI AR5 Figure 5.2 {p.395 (411/1552)}) tend to grow as we move to the past; spanning not only in the vertical axis (in CO2 RF or GST), but in the time axis. Thus, reconstructing CO2 RF, or GST, vs. time: becomes a highly inaccurate issue”.
So Nic, now that you understand my view, please demonstrate to all of us why you agree with IPCC: why is there only about a 10% probability of ECS being below 1°C?. [you cannot expect Lewis to go through your document now; make your comment more on topic]
This is a very interesting discussion. Here’s how I think about the low end of the climate sensitivity range. Doubling carbon dioxide by itself gives you about 1.2°C of warming. Add in the water vapor and lapse-rate feedbacks, which we have pretty high confidence in, and you get close to 2°C. Then add in the ice-albedo feedback and you get into the low 2s. To get back down to 1.5-ish, the cloud feedback needs to be large and negative. Is that possible? Yes, but essentially none of the evidence supports that. Instead, most evidence suggests a small positive cloud feedback, which would push the ECS to closer to 3°C. There are nuances to how to interpret this, of course, (e.g., these were derived from inter annual variations, not long-term climate change) but I find these estimates, all based on observations, to be pretty convincing.
As far as the ECS calculations based on the 20th-century observational record go, I think they’re useful and interesting, but I have less confidence in them. What’s particularly troubling to me is that we have no observations of forcing — it is an entirely model-generated parameter. If there is a single most troubling weakness in any of the calculations, to me that is it. Thus, I put most of my confidence in the bottom-up estimate described in the last paragraph and conclude that the climate sensitivity is going to be above 2°C.
If you ask what evidence would convince me that the ECS was 1.5°C, it would be evidence of a negative feedback that could cancel the known positive ones.
FYI, I make this argument in this YouTube video: http://www.youtube.com/watch?v=mdoln7hGZYk
Thanks, Andy Dessler
Dear James, John and Nic,
Thanks for your last comments.
@James: You indicate Nic seriously underestimates the uncertainties in observational based studies (i.e. they use a far too simple ‘model’), but at the same time you say ’these approaches tend to be the most reliable’. I interpret this as you partly agree with Nic that these approaches should be weighted stronger than studies based on other lines of evidence. I guess that is also why you arrive at a range for ECS (i.e. 2.0 – 3.0) in the lower part of the IPCC range. Am I right? And if so do you consider this range of 2.0 – 3.0 as a likely range or a very likely range?
@Nic: you write ‘I did not claim that observations alone can be used to generate a useful estimate of ECS.’ To be honest, as far as I have read your contributions I do think you did such a claim, but maybe I have interpreted them incorrectly. Could you explain what else is needed to generate an estimate for ECS?
Regarding your second point, what does it exactly imply? It is obvious to me that if a model and a method have been fixed, than ECS is completely determined. But the same holds for the other models and methods used in the other lines of evidence. Does it not?
Finally, Andy Dessler indicates that particulary troubling is the fact that there are no observations of forcing (and thus introducing a large uncertainty in the ‘energy budget’ method) especially due to the uncertainties in aerosols. What is your reply on that, Nic?
@John: you indicate that observational based studies could have an important role in constraining ECS if observations would be used in combination with GCMs. Then you come up with a range for ECS of 2.0 to 4.5 and a best estimate of about 3.4. Could you say a bit more how you arrive at these numbers? (Especially the relatively high best estimate).
You mention Shindell(2014) and Kummer and Dessler (2014) as a possible explaination for the difference between studies based on the instrumental period and the ones based on other lines of evidence. Could you indicate in a few sentences how these studies close the gap? (And in what direction?)
Finally, in a public comment, Andy Dessler adds that a (strong) negative cloud feedback is needed to get an ECS as low as suggested by Nic. However, current studies suggest the opposite, he says. However, in his guest blog Nic writes that: ‘observational evidence for cloud feedback being positive rather than negative is lacking’. This is a remarkable contradiction that needs some clarification, I would say. Especially because Nic also writes that Global Circulation Models (GCMs) have too high ECS values (i.e. over 2°C) due to positive cloud feedbacks and adjustments.
Looking forward to your responses.
In reply to Andrew Dessler:
I think that your argument regarding sensitivity is broadly reasonably and indeed we used it as a basis for a vague prior in our 2006 paper, but I don’t think such an argument from ignorance (ie, we don’t know much cloud feedback) can really be used as a confident estimate. Assuming the comment about forcing being model-generated refers primarily to anthropogenic aerosols, I’d be interested to hear how your calculations work out when applied to the last 30 years when by common consent, the change in aerosol forcing has been fairly modest.
I agree that Bart has made a good summary. I will attempt here to address his second question.
Let me start by saying that I reciprocate Johns views: I do find “pros” for approaches attempting to estimate ECS from the complex numerical climate models and studies of feedbacks represented in them as well as from the observational record. I think that such approaches may offer the most accurate way of constraining ECS once they are known to represent all significant climate system processes sufficiently accurately. In the meantime, they complex climate models play many other important roles – not least in helping gain a better physically-based understanding of the workings of the climate system. I agree that very simple statistical models cannot provide much help gaining such understanding, even if they currently offer the most robust way of estimating ECS.
In his piece, John discussed simulations by the NCAR CCSM4 model in some detail. One of my well informed contacts in the UK climate modelling community told me that the NCAR model was one of only three CMIP5 models in the world that they considered to be good. But, as Figure 5 in my guest blog shows, over the 25 year period 1988-2012 it simulated four times faster warming in the important tropical troposphere than the average of the two satellite-observation based datasets (UAH and RSS), and five times faster than the ERA-Interim reanalysis (which I understand is thought to be the best of the reanalysis datasets).
As shown in my Figure 4, CCSM4 also simulated global surface warming over the 35 year period 1979-2013 more than 50% higher than HadCRUT4 – and than either of the other two main observational datasets. Moreover, 1979–2013 is a period in which natural internal variability seems to have had a positive influence on global temperatures. That is due to the Atlantic Multidecadal Oscillation (AMO) having moved from near the bottom to near the top of its range over that period, according to NOAA’s AMO index (a slightly smoothed version of which is available here : the black line in panel a). Over the 64 year period 1950-2013, which started and ended with the AMO index at much the same level, CCSM4’s trend in simulated global surface temperature was nearly 85% higher than per HadCRUT4.
IMO, no sensible scientist would place his faith in the sensitivity of a model that has performed like this being anywhere near correct, or indeed view the model itself as satisfactorily representing the real climate system.
I agree with John’s comment that the quality of an ECS estimate depends on the quality of the model (as well as of the observations). But quality, in this context, means how accurately the model translates the observations into an estimate that correctly reflects the information the observation provides about ECS. A simple statistical model may in this context be much higher quality than a sophisticated method based on a state-of-the-art coupled GCM. This perspective is not at odds with my statement that sound observationally-based estimates are “completely determined by the actual observations”. My next sentence read: “To the extent that the model, simple or complex, used to relate those observations to ECS is inaccurate, or the observations themselves are, then so will the ECS estimate be.”
Consider estimating a distance on the ground by measuring on a map. The estimate will entirely depend on the measurement made, but will be inaccurate if the map is poor and/or if the wrong scale factor is used. The point I was making is that ECS values of GCM are not completely determined by the observations, even though model development is informed by observations. Nor are ECS values so determined where they are estimated by methods that are unable – typically because of climate model limitations or use of expert priors – properly to sample the entire range of values of ECS and other parameters being estimated alongside it, ignoring any part ruled out by the observations.
I would like to respond to John’s suggestion of exploring methods to estimate ECS from the observational record in a framework that is tightly constrained, using a model whose sensitivity is known and whose variability is thoroughly vetted. I concur, although depending on the approach used the realism of variability in the model with known sensitivity may not matter.
One approach is to use a detection and attribution method, comparing model simulations and observations for some “fingerprint” of the forcing of interest and using regression to find the best scaling factor. This provides estimates of TCR more readily than of ECS. If the scaling factor is for the response to greenhouse gases (GHG) over the last 60 or more years of the instrumental period, multiplying the scaling factor by the model’s TCR provides an observationally-based estimate of TCR. Figure 10.4 of AR5, panel (b), shows the GHG scaling factors (green bars) estimated by three such studies. The corresponding observationally-based TCR estimates for the nine CMIP5 GCMs studied in Gillett et al (2013), which uses the longest data time series, have a median of 1.45°C, close to median estimates of ~1.35°C that I have derived using simple energy balance approaches. A problem with such studies is difficulty in obtaining a complete separation of responses to different forcings. Incomplete separation between responses to GHG and aerosol forcing may lead to overestimation of the GHG scaling coefficient and hence of TCR.
An approach that avoids the separation problem is to systematically vary parameters of a climate model, varying the model physics so as to achieve a large number of different combinations of ECS, ocean vertical diffusivity (or other measure of ocean heat uptake efficiency), aerosol forcing and any other key climate system properties, and performing simulations for each – so-called PPE studies. Obviously, those properties must be calibrated in relation to the model parameters. The simulation results are then compared with observations and the best fit found. The fidelity of model variability is normally not critical since its effects are suppressed by using averages over ensembles of simulations. Real-world variability and covariability is then estimated from one or more separate much longer simulation runs, not necessarily by the same model, and appropriately allowed for.
This PPE approach can be used with full scale coupled GCMs, but the extensive supercomputer time required is very expensive. More seriously, it may prove impracticable to explore all combinations of climate system properties that are compatible with the observations. That was the problem with the Sexton et al (2012) and Harris et al (2013) PPE studies, as explained in my guest blog. often used In the idea is to minimise the effects of model variability by using ensembles of simulations, real-world variability then being allowed for using simulations by a more complex model.
It is more usual to use a PPE approach with models that are simpler than a GCM, typically resolving the globe horizontally only by hemisphere and land vs ocean, and maybe having a single layer atmosphere. However, several such studies have been carried out using the MIT climate model, which is in effect a 2D GCM, key parameter settings of which have been calibrated against 3D coupled GCMs. Longitude, which is not resolved, is generally far less important than latitude. Such studies include Forest et al (2002 and 2006), Lewis (2013) and Libardoni & Forest (2011, corrigendum 2013). Internal covariability was allowed for by the use of long control run simulations from full coupled GCMs, and multiple observations were used to constrain ECS, aerosol forcing and ocean effective diffusivity. Are such studies in principle more acceptable to John than observationally-based estimates using simpler numerical climate models or simple mathematical/statistical models?
Bart,
OK, you’ve put me on the spot. I was deliberately a little vague in my initial estimate, because I had not done any detailed calculations recently and there’s been a lot of new literature in the last couple of years. I do think that my range of 2-3C could be considered “likely”, bearing in mind that this still leaves a substantial probability (33%) of a value outside that range.
I don’t really like the term “weighting” as it might be interpreted as taking some sort of weighted average, which I don’t think is really appropriate. But yes, I do consider the transient 20th century warming-based estimates more trustworthy than other approaches, as they are more-or-less directly based on the long-term (albeit transient) response of the climate system to anthropogenic forcing, which is after all what we are interested in here!
Hi Bart,
Thanks for the additional questions. Firstly to clarify my position, I have indicated that so-called instrumental-record studies could play an important role in the discussion if they were more thoroughly vetted and understood. One need not use a GCM at all, though that approach could provide a useful well-constrained framework for such a vetting. The fact that this has not yet been done in any thorough sense to me is startling, given the sweeping statements that have been made based on such techniques and given how widely scrutinized other approaches (e.g. GCMs) have been.
My basis for the lower part of my estimated range is very much in line with Andy’s comments based on feedbacks – an approach I focus on in my original post. I know of no valid studies supporting the strong negative cloud feedback needed to arrive at a sensitivity well below 2C. I know of several claiming to show such a negative feedback that have been revealed (by myself and others) to clearly be wrong (Lindzen and Choi, Spencer and Braswell, among others). Lindzen himself has admitted to major errors in this work (http://dotearth.blogs.nytimes.com/2010/01/08/a-rebuttal-to-a-cool-climate-paper/?pagemode=print). From other recent work, (multiple works each by Soden, Webb, Romanski/Rossow, Sherwood, Brient/Bony, Gregory, Gettelman, Dessler, Jonko, Norris, Sanderson, Shell, Bender, Vechhi, Lauer …) that examine the issue across observations, cloud resolving models, and GCM archives of various sorts, there is persuasive evidence that the feedback is not strongly negative but rather is likely to be positive, perhaps strongly so. Clearly there remains a considerable range of uncertainty on the exact value of the feedback but in my view the evidence does not allow for a strong negative feedback. And so how does one construct a physical basis for a value well below 2?
My upper end of the range is based on my evaluation of models and related work in the literature (e.g. by many of the above mentioned authors). For instance, in my view, the CESM1-CAM5 ensemble that I present in my Fig. 2 shows no obvious bias in its reproduction of the surface temperature record yet its sensitivity is 4.1! Again, the main disparity between the observed record and the ensemble mean occurs during the hiatus, yet this does not accompany any reduction in the planetary imbalance (in nature or comparable model ensemble members) and therefore is not evidence for a strong negative feedback. It is therefore also not an indication of biases in model feedbacks and is not a basis for revising our sensitivity estimates downward. Moreover, the key processes that drive sensitivity are actually better represented in many of the high sensitivity models (Fasullo and Trenberth 2012, Sherwood et al. 2014) and the sensitivities of the poorest performing models in CMIP3 (e.g. 2.1 of NCAR PCM1 which we know has major problems) have not been reproduced by models in CMIP5, as a broader improvement (though not perfection) of key processes has been realized.
Regarding the work on efficacy, I’ll let texts from the abstracts do the talking, paraphrasing where useful.
Shindell: …transient climate sensitivity to historical aerosols and ozone is substantially greater than the transient climate sensitivity to CO2. This enhanced sensitivity is primarily caused by more of the forcing being located at Northern Hemisphere middle to high latitudes where it triggers more rapid land responses and stronger feedbacks. I find that accounting for this enhancement largely reconciles the {instrumental and GCM ranges}.
Kummer and Dessler: Previous estimates of ECS based on 20th-century observations have assumed that the efficacy is unity, which in our study yields an ECS of 2.3 K (5%-95%- confidence range of 1.6-4.1 K), near the bottom of the IPCC’s likely range of 1.5- 4.5 K. Increasing the aerosol and ozone efficacy to 1.33 increases the ECS to 3.0 K (1.9-6.8 K), a value in excellent agreement with other estimates. Forcing efficacy therefore provides a way to bridge the gap between the different estimates of ECS.
John comments that from his point of view, Shindell (2014) and Kummer and Dessler (2014) provide a viable rationale for reconciling disagreements between different methods of estimating ECS, and asks if there is any basis for rejecting them outright. The answer to that question is yes in relation to Kummer & Dessler (2014), and to a very large extent in relation to Shindell 2014.
To avoid a very lengthy comment, I will just address Kummer & Dessler (2014) here. It is titled “The impact of forcing efficacy on the equilibrium climate sensitivity” and states that ‘Recently, Shindell [2014] analyzed transient model simulations to show that the combined ozone and aerosol efficacy is about 1.5.’ Kummer & Dessler estimate ECS using an energy balance method, as per Equation (1) in my blog, based on a forcing estimate with ozone and aerosol forcing either unscaled (giving an ECS best estimate of 2.3°C) or, following Shindell (2014) scaled up by an efficacy of 1.5 or 1.33 (giving best estimates for ECS of respectively 3.0°C or 3.5°C). I am afraid that there are several problems with their paper.
First, what Shindell actually discusses is transient sensitivity to inhomogeneous aerosol and ozone forcings being higher than to homogeneous CO₂ forcing. He never claims that these inhomogeneous forcings have a efficacy of greater than one. He never refers to efficacy at all in his paper or its Supplementary Information.
The efficacy of a forcing agent is the surface temperature response to radiative forcing from that agent relative to the response from carbon dioxide forcing. Studies of the efficacy of aerosol forcing (including by Hansen and by Shindell) have typically found a value close to one. As AR5 says, by including many of the rapid adjustments that differ across forcing agents, the effective radiative forcing (ERF) concept it uses – which is generally also used in energy budget ECS estimates – in any case includes much of their relative efficacy. Shindell’s claim isn’t that inhomogeneous forcings (mainly aerosol) have a high efficacy, but that they are concentrated in regions of high transient sensitivity, thereby having more effect on global surface temperature than if they were uniformly distributed.
Presumably as a result of Kummer & Dessler confusing forcing efficacy with transient climate sensitivity, their calculations make no physical sense. Their method appears to hugely over-adjust for the effects on ECS estimation of the higher transient sensitivity to aerosol and ozone forcings that Shindell (2014) estimates. Troy Masters has an excellent blog explaining this problem here.
Secondly, Kummer & Dessler state that their forcing time series is referenced to the late 19th century and accordingly use a reference (base) period to measure changes in global surface temperature from of 1880-1900. That would be fine were it true, but it is not. Their forcing time series actually come from AR5 and are referenced to 1750. The mean total forcing during 1880-1900 was substantially negative relative to 1750 due to high volcanic activity. Referencing the forcing change to a base period of 1880-1900, as necessary to match their temperature change, reduces their non-efficacy-adjusted ECS estimate to 1.5°C. And their headline 3.0°C best ECS estimate, based on an aerosol and ozone ‘efficacy’ of 1.33 and their faulty adjustment method, become 1.7°C.
There are other issues with the paper, but I will leave it at that. I’ve probably already upset Andrew Dessler quite enough!
To James Annan:
Overall, I think I agree with James’ comments — I wish my argument were stronger. However, to be fair, it’s important to realize that there are no really strong arguments for any particular climate sensitivity range — if there were, we wouldn’t be having this argument. Rather, any argument about climate sensitivity requires you to evaluate conflicting arguments and decide one is right and the other isn’t. So while I think that ECS > 2°C, I understand the IPCC authors who decided the ECS > 1.5°C.
To Nic Lewis:
I appreciate your comments. Your statement about the referencing period of the forcing is correct and that will be corrected in the galleys. Assuming that the climate in the late 19th century is warmer than that in the mid 18th century (probable since radiative forcing is +0.3 W/m2 in the late 19th century), then referencing both time series to 1750 will increase the calculated climate sensitivity (I can explain why if it’s not clear). Thus, it does not affect our conclusion that incorporating efficacy has a significant effect on the inferred climate sensitivity.
I also agree that there is a useful clarification to be made between Shindell’s analysis and ours. The efficacy in Shindell’s analysis is a combination of a heat-capacity effect and an effect from differing climate sensitivities to aerosols/ozone and greenhouse gases. The effect due to differing heat capacities is not relevant for the ECS, but the other one is. Given the weaker radiative restoring force at high latitudes, I find it perfectly reasonable that there is a significant difference in sensitivity to these different forcers — and if there is, it resolves an otherwise confusing situation. As we say in the paper, determining this is should be a priority.
To John Fasullo:
I agree with just about everything you say!
Nic Lewis here exhibits a decidedly un-self-critical attitude in his comments here – I don’t think this reflects well on his arguments, or for the likelihood of any resolution of this “dialogue”. To move forward it is essential to recognize merits in opposing views, in fact to try to acknowledge the best arguments the “other side” may have. Both John Fasullo and James Annan do this in their comments, describing Lewis’ and similar instrumental-based approaches in very fair terms, with considerable praise for their good points. But Lewis insists on an extremely biased presentation. As just one very clear example to me, he seems to acknowledge none of the previous debate that occurred in this forum on the tropical hot spot – citing conflict between models and “observations” on mid-troposphere warming as an indictment of the models, when in fact the measured trends are clearly still very uncertain. Lewis asserts several similar claims that fall down if proper uncertainty measures are applied.
A little more honest self-criticism would be a huge help here. And addressing what seem to be contradictions in what’s been raised already, for example the one Bart Strengers pointed out, is also important.
Bart queries my comment that ‘I did not claim that observations alone can be used to generate a useful estimate of ECS.’ and asks what else is needed to generate a estimate for ECS. I think this is an issue of terminology. When I refer to observational estimates, I do not imply that a sound ECS estimate can be derived from observations alone. As I wrote in my guest blog, ‘Whichever method is employed, GCMs or similar models have to be used to help estimate most radiative forcings and their efficacy, the characteristics of internal climate variability and maybe other ancillary items.’
Let me take the example of an energy budget estimate of ECS, using Equation (1) in my guest blog. The change in global surface temperature is typically taken from a dataset that involves multiple measurement time series at different locations and a more or less sophisticated mathematical method of averaging the measurement, adjusting for inhomogeneities, etc. That method could be regarded as a model, but not in the normal sense of the word. Most people would view HadCRUT and other global temperature estimates as observational data, not model outputs. Planetary heat uptake / radiative imbalance in the final period can be calculated in similar ways. These may involving rather more processing and adjustments, but the outcome is still generally regarded as observational data.
On the other hand, it is generally necessary to rely on coupled GCM simulations to derive heat uptake in the base period, since that is typically in the second half of the nineteenth century, before proper observations of ocean temperatures at depth started. That estimate will have a first order dependence on the GCM’s ECS value. However, the absolute value of heat uptake in the nineteenth century is small, so it has only modest effect on ECS estimation, and an approximate adjustment can be made to the GCM’s simulated value by reference to the relationship of the GCM’s ECS to the energy budget ECS estimate.
The remaining term involves radiative forcings. Although the most important radiative forcings (those from greenhouse gases) can be estimated without use of GCMs, some radiative forcings cannot ,and nor can effective radiative forcing (ERF) – which is more appropriate for energy budget estimates – be estimated without use of GCMs. However, ERF estimates do not rely on the ECS values of the models involved. There is, for instance, a negligible correlation between ECS and F_2x (the ERF from a doubling of CO2 concentration) in the CMIP5 models included in Table 5, which have ECS values ranging from 2.1°C to 4.7°C, is negligible. And in any event, for most forcings (aerosol forcing being an exception) ERF is not estimated to be significantly different from plain radiative forcing.
So, to summarise, GCMs or similar climate models are needed for observationally-based climate sensitivity estimates, but their ECS values have very little effect on those estimates.
Bart’s summary is very good and I believe so far the most insightful observations is this one from James Annan:
1. These studies assume an idealised low-dimensional and linear system in which the surface temperature can be adequately represented by global or perhaps hemispheric averages. In reality the transient pattern of warming (or the effective CS) is different from the equilibrium result, which complicates the relationship between observed and future (equilibrium) warming (Armour, 2014).
and all main points from John Fasullo:
1. These studies are severely limited by the assumptions on which they’re based, the absence of a unique “correct” prior, and the sensitivity to uncertainties in observations and forcing (Trenberth 2013).
2. Uncertainty in observations and the need to disentangle the response of the system to CO2 from the convoluting influences of internal variability and responses to other forcings (aerosols, solar, etc) entails considerable uncertainty in ECS (Schwartz, 2012) and thus: 1) the use of a model is unavoidable, 2) it is a misnomer to present 20th Century instrumental approaches as being “observational estimates”.
3. Limited warming during the hiatus does not point at a low ECS but has been driven by the vertical redistribution of heat in the ocean, confirmed by persistence in the rate of thermal expansion since 1993 (Cazenave et al 2014).
4. Recent observations have reinforced the likelihood that the current hiatus is consistent with such simulated periods.
5. Attempts to isolate the effects of CO2 on the temperature record are inherently an exercise in attribution and the use of a model is therefore unavoidable.
6. Lewis underestimates the weaknesses and in doing so is at odds with the originators of this method (e.g. Forster et al. 2013).
7. Statistical techniques, particularly when trained over a finite, complex, and uncertain data record in which forcings are also considerably uncertain, are no panacea to the fundamental challenge of physical uncertainty.
8. Assessing ECS solely with statistical approaches using simple models that capture little of the climate system’s physical complexity, trained on a limited subset of questionably relevant surface observations, and based on largely untested physical assumptions is impossible.
What I find most relevant and yet surprisingly not mentioned yet is the kind of limitations in the current models raised by papers like England 2014, which IMHO suggest that ECS might be underestimated and TCR might be slightly overestimated by current models. Having models that are able to reflect that kind of evidence would be a major step forward, would certainly close the gap between various lines of ECS estimates and might also provide insight into scenarios where the radiative imbalance (between a fast-increasing forcing and a very slow-increasing ocean surface) goes faster to much higher levels than the current generation of models suggest.
Bart also queries my implicit claim that even when a model and a method have been fixed, then in unsound studies ECS may not be completely determined by the observations. I should say that I regard a case where the model and method result in the best estimate for ECS potentially being substantially different from the value at which the model indicates a best fit to the observations as not completely determining ECS from the observations.
As I pointed out in a previous comment, there are two obvious ways that this situation can arise. One is where, a study is unable because of climate model limitations – properly to sample the entire space of values for ECS and other parameters being estimated alongside it that is compatible with the observations. This is a major problem with GCM-based PPE studies: varying the GCM’s parameters may not achieve even a moderately low ECS. I think James found this problem with a Japanese GCM.
Even if reasonably low ECS values can be achieved, they may always be accompanied by values for other climate system properties that make the simulated climate unrealistic. As I have shown, that is the problem with studies based on the UK HadCM3 model, which has been used a lot for such studies. HadCM3 seems unable to exhibit ECS values below 2°C whatever its parameter settings, although somewhat lower ECS values have been extrapolated by statistical emulation. But even with ECS reduced just to 2°C, the parameter setting required produce much more highly negative aerosol forcing, resulting in an unrealistically cool climate. This is probably because both low ECS values and high aerosol forcing result from low clouds being increased in extent and maybe having different properties. HadCM3 studies therefore simply cannot explore the combination of low-to-moderate ECS and moderate aerosol forcing that the observations point to.
The other obvious case is where the statistical method uses a highly informative prior. An obvious example is an expert prior for ECS. If one uses, as Tomassini et al (2007) did, a sharply peaked expert prior that falls to one-fifteenth of its maximum (achieved at an ECS of 2.5°C) at ECS values of 1°C and 6°C, then the best estimate – taken in AR5, correctly, as the median of the estimated (posterior) PDF for ECS – is obviously going to be pushed towards values somewhere in the middle of the 1°C and 6°C range.
But a uniform prior for ECS is also highly informative. The observable variables have a much more linear relationship to the reciprocal of ECS, the climate feedback parameter (lambda), than to ECS itself. It follows that a uniform prior in lambda is fairly uninformative. But if a uniform prior in lambda is uninformative for estimating lambda, it follows mathematically that for a prior in ECS to be uninformative it must have the form 1/ECS^2. That is, the prior should quarter each time the ECS value doubles. Even with a fairly well-constrained observational likelihood (the model–observation fit being good only over a limited range), the use of a uniform prior in ECS has a major distorting effect.
Compare the two ECS ranges shown (purple lines 3rd and 4th up from the bottom of the Instrumental section) in AR5 Box 12.2 Figure 1 for the Forster & Gregory (2006) study. The solid line, showing the study’s regression-derived original results, has a 5–95% range of 0.9–3.5°C and a best (median) estimate of 1.5°C. The standard regression method implicitly, and correctly, reflected a uniform-in-lambda prior. The dashed line, showing the estimate reported in AR4 – which had, for no valid reason, been transformed onto a uniform-in-ECS prior, has a 5–95% range of 1.2–7.9°C and a best estimate of 2.4°C. The best estimate is increased by 50%+ and the top of the uncertainty range is more than doubled!
In his final question to me, Bart asks for my view on Andy Dessler’s comment that there are no observations of forcing (and thus introducing a large uncertainty in the ‘energy budget’ method) especially due to the uncertainties in aerosols.
Well, there are solid line-by-line radiative transfer calculations of forcing by greenhouse gases, based on solid physics. As I’ve explained, some other forcings have to be derived with the help of GCMs, as do conversion factors from plain radiative forcings to ERFs (all near unity in fact), but are to first order at least independent of the GCM ECS values. Chapter 8 of AR5 spells out the basis for the estimates of the various forcings and their uncertainties. Forcing estimates diagnosed from GCMs are on average similar to AR5’s best estimates apart from in respect of aerosol and volcanic forcing. If Andrew Dessler wants to reject AR5’s forcing estimates, that’s up to him. But GCM-based projections of future warming depend on their estimation of forcing, so if he rejects those then he must also discard GCM projections of future warming.
As AR5 states, the most important uncertainties by far are in aerosol forcing. (There is also significant uncertainty in the ERF of CO₂, but when estimating ECS this largely cancels out with the corresponding uncertainty in F₂ₓ.) If aerosol was known to have a current ERF of -0.9 W/m², in line with AR5’s best estimate, then energy budget estimates of ECS using data from AR5 would be quite narrowly constrained around a best estimate in the 1.5–2.0°C range.
There are observationally-based aerosol forcing estimates, derived from satellite instrumentation, although they do involve a number of assumptions. The mean estimate of total aerosol ERF from all satellite studies used in forming AR5’s expert best estimate was -0.78 W/m². That best estimate was also informed by model-based aerosol forcing estimates, averaging -1.28 W/m². Hence the wide and asymmetrical 5-95% uncertainty range in AR5 of -1.9 to -0.1 W/m².
If aerosol forcing is in line with or smaller (less negative) than AR5’s best estimate, then there can be little doubt that most of the CMIP5 models are oversensitive. Narrowing the uncertainty range for aerosol forcing is key to obtaining narrowly constrained estimates for ECS and TCR, and hence for projecting future warming.
The importance of aerosol forcing uncertainty was the main message from the Schwartz (2012) paper ‘Determination of Earth’s Transient and Equilibrium Climate Sensitivities from Observations Over the Twentieth Century: Strong Dependence on Assumed Forcing’ the uncertainty assessment of which John cited approvingly. In Schwartz’s conclusions, he wrote: ’the forcing due to anthropogenic aerosols is the source of the greatest uncertainty, and it this uncertainty that is mainly responsible for the differences in forcings over the twentieth century.’ Yet John implicitly rejects Schwartz’s finding that, notwithstanding high aerosol forcing uncertainty, a 95% upper bound of 1.9°C could be put on TCR (his best estimate being 1.3°C) , which is below the TCRs of almost half of the CMIP5 models.
I was not familiar with Nic Lewis’ 2013 paper, which figures strongly in his view of a low climate sensitivity, so I had a look at it. He uses the well-known method of Chris Forest to simultaneously estimate aerosol forcing, ocean heat uptake efficiency and equilibrium climate sensitivity from historical data, but extends it using more recent data and makes a few other changes to the method. Historical data is one of three constraints on climate sensitivity, the other two being information on prehistoric climate change (paleoclimate) and climate sensitivity calculated from first principles (climate models). Of the three, the estimates from historical data have generally been lower than those from paleo data or from models. Recently Otto et al. 2013 showed that the estimate drops still furhter when the most recent data are used, and Lewis shows an even larger drop, to a climate sensitivity likely near or below 2C.
The problem with estimating climate sensitivity from recent historical data is that the answer is very sensitive to aerosol forcing, which is poorly known, and (despite what Lewis says) such estimates also depend on models. The Forest/Lewis method assumes that aerosol forcing is in the northern hemisphere (establishing the “fingerprint”), so in effect uses the interhemispheric temperature difference to constrain the aerosol forcing.
In the last couple of decades, northern high latitudes have warmed dramatically while the southern high latitudes have warmed very little if any. Forest’s approach will implicitly attribute this to a positive aerosol forcing over that period, in contrast to the negative forcing that would be expected given the increase in aerosol precursor emissions over that time. This leads to a very small estimate of the climate sensitivity, since if I understand correctly, the method will believe that aerosols were adding to CO2 forcing rather than opposing it as we would normally think based on independent evidence including satellite observations of aerosol forcing. Such a large forcing, with less than 1C warming, would if it were true imply a low sensitivity.
The problem is that this interhemispheric warming difference since the 1980’s is almost certainly not aerosol-driven as the Forest/Lewis approach assumes. It is not fully understood but probably results from circulation changes in the deep ocean, unexpectedly strong ice and cloud feedbacks in the Arctic, meltwater effects around Antarctica, and/or the cooling effect of the ozone hole over Antarctica. Most of these things are poorly or un-represented in climate models, especially the MIT GCM used by Forest and Lewis, and these models display too little natural decadal variability. It is thus not surprising that GCMs have great difficulty simulating the recently observed decadal swings in warming rate (including the so-called “haitus” period where they overestimate warming, and the previous decade where they typically underestimated it). By implicitly attributing a pattern to aerosol that is probably due to other factors, Forest (and especially Lewis) are underestimating climate sensitivity. Other evidence such as the continued accumulation of heat in the worlds’ oceans is also inconsistent with the hypothesis that the slow warming rate in the last decade or two is due to negative feedback in the system as argued by Lewis.
A more general problem with Lewis’ post is that he dismisses, for fairly aribtrary reasons, every study he disagrees with. The fact is that no way of estimating climate sensitivity is solid, and we have to consider all of them (except Lindzen and Choi which is based on a ridiculous argument that is contradicted by their own data). Lewis dismisses climate models because they supposedly can’t simulate clouds properly, ignoring the multiple lines of evidence for positive cloud feedbacks articulated in Chapter 7 of the 2013 WGI IPCC report as well as the myriad studies (including my Nature paper from this year) showing that the models with the greatest problems are those simulating the lower climate sensitivities that Lewis favours, not the higher ones he is trying to discount.
If we look at all the evidence in a fair and unbiased way, we find that climate sensitivity could still be either low or high, and that it is imperative to better understand the recent climate changes and the factors that drove them. My money is on the models and the paleo data, not the estimates based on the 20th century. Although I hope I turn out to be wrong.
I have two points that I’d like to put to John.
First, in his blog, John said we should move beyond global mean surface temperature (GMST) as the main metric for quantifying climate change, and drew attention to the improved estimates of ocean heat content (OHC) made possible though data from Argo buoys. John stated that OHC in the 0-2000 m deep layer had increased fairly consistently since circa 1990. He showed a graph of the Levitus et al 2012 pentadal observational estimates, updated by NOAA up to that centred on 2011. Ocean heat uptake (OHU), the rate of increase in OHC, shown by the graph was equivalent to ~0.30 W/m² over the Earth’s entire surface over 1992-2001, and 0.2 W/m² higher at ~0.50 W/m² over 2002-2011. Lyman & Johnson (2014), using a different averaging method, reached a rather lower OHU of ~0.3 W/ m² for the marginally less deep 0-1800 m ocean layer over 2002-2011. AR5 estimates total heat uptake in the ocean below 2000 m and by land, ice and the atmosphere at 0.1 W/m² over 2002-2011. Adding that to the mean of the Levitus et al and Lyman & Johnson estimates implies a total radiative imbalance at the top-of-atmosphere (TOA) of 0.5 W/m² over 2002-2011, in line with the Loeb et al (2012) estimate for 2001-2010.
John also discussed the NCAR CCSM4 model in favourable terms. The CCSM4 model shows a mean TOA radiative imbalance of 1.1 W/ m² over 2002-2011, twice the estimate calculated above from observational data. That is the greatest overestimation of any CMIP5 model. May I ask him why he does not regard that as a serious failure of the CCSM4 model, sufficient inter alia to make it almost certain that either or both its ECS and TCR values are unrealistic?
Speaking of unrealistic, it although ocean reanalysis methods may as John says have improved, it is evident that the ORAS4 reanalysis (Balmeseda et al, 2013) is unrealistic: unlike any of the observational datasets, it shows a major fall in OHC after the Mount Pinatubo eruption in 1991. Having studied the ORAS4 technical manual, I am not surprised that this reanalysis is heavily model-influenced.
Secondly, another key ocean-related metric for quantifying climate change and assessing the realism of climate models is cross-equatorial ocean heat transport, a critical component of the climate system. The general understanding is that this is in fact quite small. One source for that is Trenberth & Fasullo (2008), which concluded: ’the annual mean cross-equatorial transport is negligible (<0.1 PW), with an upper bound of 0.6 PW.' Figure 9.21 of AR5 shows that zero estimate and three others, of 0.3, 0.0 and 1.0 PW northward. The last of those (from Large & Yeager, 2009) relies heavily on very uncertain calculations: its observational estimates from a 2001 source do not really constrain the rapidly changing Indo-Pacific ocean heat transport south of 10°N. More recently, Marshall et al (2014) derive an estimate (averaging over their datasets) of 0.35 PW northward.
The above five estimates of cross-equatorial ocean heat transport average 0.2 PW northward. Figure 9.21 of AR5 shows that, by contrast, the CMIP5 models have a mean northward cross-equatorial ocean heat transport four times higher, at 0.8 PW northward. The only models with heat transports substantially below 0.8 PW are INM-CM4, IPSL-CM5A-LR, IPSL-CM5A-MR and IPSL-CM5B-LR. Does this not suggest that there are fundamental problems in virtually all the CMIP5 models, quite apart from their major overestimation of surface warming over the last 35 years and vast overestimation of tropical lower tropospheric temperature over the last 25 years? The 0.6 PW excess of the CMIP5 multimodel mean northwards ocean heat transport over the average of the observationally-based estimates is equivalent to an excess of forcing in the northern hemisphere over the southern hemisphere of 4.8 W/m². That excess is greater than estimated total anthropogenic forcing, so this is a major issue.
My concern is that ECS en TCS, as defined, can never be measured, because you cannot carry out the necessary experimentation with the earth. Therefore, they are strictly metrics describing the behaviour of climate models. Of course, a model with high sensitivity is likely to predict a larger temperature increase in response to an increase in CO2 as compared to low sensitivity model. Therefore, it is certainly of interest to compare sensitivities of different models. Discussing the climate sensitivity as a property of nature, however, is rather meaningless, in my opinion. It makes more sense to compare actual forecaste made with different models.
What I miss is a systematic discussion of the different studies comparing strong and weak points in 1. the particular definition of ECS/TCS; 2. the model used (even if you consider the model as a black box in which the temperature increase is proportional to the change of forcing this IS a model; quite poor and unrealistic in my opinion); 3. the particular observations (and or data derived otherwise) fed into the model; 4. the method used to feed data into the model.
Dear Nic, James and John,
Thanks again for your interesting posts and your willingness to answer the questions I raised.
The discussion now also went into the usability of Climate models in constraining ECS. John gave a number of arguments in favor of climate models (also in his guest blog) and why they arrive at higher ECS-values:
1. Low sensitivity models, that were amongst the oldest in the archive , can be discounted because they have difficulty in simulating even the basic features of observed variability in both clouds and radiation (Soden 2002, Mahlstein 2011, Sherwood 2014).
2. Key processes that drive ECS are better represented in many of the high sensitivity GCMs (Fasullo and Trenberth, 2012, Sherwood 2014). In fact, there is no credible GCM with an ECS of less than 2.7.
3. The decadal trends as simulated by the Community Earth System Model (CESM1-CAM5) of the National Centre for Atmospheric Research (NCAR) track quite closely with those derived from observations (see fig 2 in John’s guest blog). Yet its ECS is 4.1!
On the other hand, Nic concludes that no sensible scientist would place his faith in NCAR CESM1-CAM5, which is considered to be one of the best models in CMIP5:
1. The NCAR CCSM4 model [red: which is a subset of CESM1-CAM5] simulates over 1988-2012 four times faster warming in the tropical troposphere than the average of two satellite-observation based datasets (UAH and RSS vs CCSM4 (blue circle) and CESM1 (blue triangle) in Figure 9.9 of AR5).
2. CCSM4 simulated global surface warming over 1979-2013 more than 50% higher than the observational datasets, in particular HadCrut4.
3. Over the period 1950-2013 CCSM4′s trend in simulated global surface temperature was nearly 85% higher than per HadCRUT4.
4. The CCSM4 model shows a mean Top Of the Atmosphere (TOA) radiative imbalance of 1.1 W/m² over 2002-2011, twice the estimate calculated above from observational data. That is the greatest overestimation of any CMIP5 model.
5. NCAR CESM1-CAM5 model matches global actual warming reasonably well because the aerosol forcing was -0.7 W/m² more negative from 1850 to 2000 than the AR5’s best estimate [red: -0.9, see SPM AR5 fig 5] (Shindell et al, 2013).
6. The average of five studies shows a cross-equatorial ocean heat transport of 0.2 PW northward. Figure 9.21 of AR5 shows that the CMIP5 models have a mean that is four times higher. This is equivalent to an excess of forcing in the northern hemisphere over the southern hemisphere of 4.8 W/m², greater than total anthropogenic forcing.
With respect to Nic’s point 1, I would like to add that in our previous climate dialogue on the hot spot Mears and Christy agreed that models are showing more tropical tropospheric warming than all observations (both satellites and radiosondes); that errors in the datasets are not large enough to account for this discrepancy and that it is an important, statistically significant, and substantial difference that needs to be understood. Sherwood also agreed with respect to the satellite era (i.e. since 1979) but added that the discrepancy is not evident when looking at longer records (back to 1958).
With respect to Nic’s point 3: This seems contradictory to John’s point 3. Nic did not include a reference, John showed a figure from the CESM1-CAM5 large ensemble community project.
With respect to Nic’s point 5: what do you mean by ‘global actual warming’? If you mean ‘global surface warming’ than your point 5 seems to contradict points 2 and 3.
I am very interested in John’s reply to the points raised by Nic and vice versa.
Another important issue is on cloud feedback as already mentioned in my previous comment. John writes that recent work (he mentions 15 authors) indicate the cloud feedback is not strongly negative but rather is likely to be positive, perhaps strongly so, and a strong negative cloud feedback is needed to arrive at low ECS-values (like Nic’s). Just like Dessler, John concludes there are no valid studies supporting the strong negative cloud feedback needed to arrive at a sensitivity well below 2 C. In his public comment Steven Sherwood adds that Lewis dismisses climate models because they cannot simulate clouds properly, ignoring the multiple lines of evidence for positive cloud feedbacks articulated in Chapter 7 of AR5. Nic however, writes that ‘observational evidence for cloud feedback being positive rather than negative is lacking’. Nic, could you indicate why you say so?
@James: I am also very interested in your opinion on the issues raised above.
There were several other issues raised (also by Steven Sherwood and Gerbrand Komen), but for now I would like to first deal with the ones mentioned above.
Bart.
In response to Gerbrand’s comment (2014-05-19 11:28:18) – yes, of course any number that we calculate for a physical system is based on idealization (which is why many of the above comments describe how every such metric must be based on a model of some sort). But that does not mean the physical system itself is not fundamentally behaving in a mathematical fashion – the experience of physics in a huge variety of realms from the tiniest particle to the largest systems in the universe shows things following explainable and precise mathematical laws. So the Earth should, in principle, have that same sort of mathematical character as any other physical system. Models attempt to match that but are of course always a simplification.
If you take away some of the complications of the real Earth – day-night, seasons, changes in solar forcing, etc. and think about how such a planet would respond to changes in its own atmosphere it seems clear there should be a range of responses on different time-scales. If, say, there’s an instantaneous forcing change (say from a volcanic explosion, change happening in a day or less) then the response to that “delta-function” forcing change will be spread out over time. The immediate effect is an energy imbalance (assuming all was in balance before the change) but no temperature change at first. Wherever the energy imbalance has a direct effect, for example on the surface if the forcing change is from reflective aerosols, will start to change in temperature (cool under increased aerosol forcing). That cooling will in turn change other energy flows – radiative and convective and other, that will start to address the energy imbalance and return things to balance. It will also have other consequences such as changes in water evaporation, precipitation, ice melting, etc. that add up to feedbacks that play out over a wide range of timescales. One of the really long timescales is the response of the subsurface – and in particular the oceans with their huge heat capacity. In principle the temperature change required to reach full equilibrium needs to be not just at the surface, but across the full range of planetary systems interacting through energy flows with the surface. For a planet like Earth that leads to timescales on the order of thousands of years, necessarily.
All that is in principle describable mathematically – as a response function of the planetary temperature field as a function of time T(x, t) ~ G(x, t) delta F(t=0) – though possibly other fields may need to be included as well (ice, cloud, fresh water, etc) to handle hysteresis effects. TCR and ECS are simplified metrics describing that full response function. Necessarily there will be uncertainties in any measure through observations that tries to get a handle on those numbers, and any model of the system similarly must have uncertainties thanks to discretization and parameterizations. It’s important in comparing models with one another, and models with observations, to be very clear (and generous, even) with accounting for those uncertainties before claiming a discrepancy. Nevertheless there is an underlying mathematical reality that these are trying to get to, so it really is a useful exercise. But I do agree it might be helpful, if possible, to think about other metrics than TCR and ECS to see if we can better characterize that fundamental response with measures that might be less subject to uncertainty and easier to compare.
I have reviewed the method of estimating the climate sensitivity by comparing the Last Glacial Maximum with pre-industrial conditions, and I find the method highly speculative and not conducive to verification of the assumptions, which appear rather gross to me. In short, I don’t believe any of it. The review is ten pages long, and so is not conducive to a simple message here. It can be viewed as a pdf at:
http://www.home.earthlink.net/~drdrapp/LGM.pdf
@arthur smith
I appreciate your reacting (2014-05-19 16:23:24)to my comment.
I agree that the earth system behaves according to the laws of physics, first of all the laws of fluid dynamics, and then all the other processes that come in, but this does not provide an answer to my concern that you cannot measure ’the’ climate sensitivity. I also agree that you ‘can take away some of the complications of the real Earth – day-night, seasons, changes in solar forcing, etc. and think about how such a planet would respond to changes’ etc, but then you are really making a model. In reality it is simply impossible to do the experiment.
Maybe I am crazy, but this blocks me completely. How can one meaningfully introduce a quantity which can not be measured? I would argue, for model characterization only!
I have the same problem with the (IPCC) concept of radiative forcing. You can compute it, (‘with all tropospheric properties held fixed’) but there is no way you could actually measure it, because you can’t keep all tropospheric properties fixed if you perturb the actual system.
So, in summary, I fully agree, that there is an underlying reality, and also that climate sensitivity is useful for comparing models, but I do not see how you can measure it.
I will respond to Bart’s questions once I have studied them properly, but as I had already nearly completed a response to Steven Sherwood’s comment I have finished that first and will post it now. It may answer some of Bart’s questions in any case.
I thank Steven Sherwood for his comments. It is helpful to see some solid arguments made about my 2013 Journal of Climate study, to which I respond below. But before I do so, let me point out that the low best (median) ECS (and by implication TCR) estimates that study, and other such as Ring et al (2012) and Aldrin et al (2012) that also formed their own inverse estimates of aerosol forcing from observed spatiotemporal changes in temperature, arrived at are in line with energy balance derived estimates based on AR5’s expert best estimate of aerosol forcing.
I will quote what Steven writes in italics and put my responses in normal text.
Otto et al. 2013 showed that the estimate drops still further when the most recent data are used
That is incorrect. Otto et al 2013 reached best estimates for ECS of 1.4°C using data from the 1970s, 1.9°C using data from the 1980s, 1.9°C using data from the 1990s and 2.0°C using data from the 2000s. So using the most recent data gave the highest estimate of ECS, not a lower one. Using data for all four decades, the ECS estimate was 1.9°C.
The problem with estimating climate sensitivity from recent historical data is that the answer is very sensitive to aerosol forcing, which is poorly known, and (despite what Lewis says) such estimates also depend on models.
The suggestion that I claimed ECS estimates from recent historical data were independent of models is untrue. I wrote in my blog ” Whichever method is employed, GCMs or similar models have to be used to help estimate most radiative forcings and their effectiveness, the characteristics of internal climate variability and various other ancillary items.”
The Forest/Lewis method assumes that aerosol forcing is in the northern hemisphere (establishing the “fingerprint”), so in effect uses the interhemispheric temperature difference to constrain the aerosol forcing.
That is only partly true. The MIT 2D GCM used has a 4° latitudinal resolution, as good as many 3D GCMs of its day. Time-varying aerosol loadings are applied as a function of latitude, and will by no means be located only in the northern hemisphere. For the surface diagnostic used, the resulting surface temperature changes in four equal-area latitude zones, not just hemispheres, are compared with observed changes over each of five (Forest) or six (Lewis) decades. The upper air diagnostic uses temperature changes at eight levels and a 5° latitudinal resolution, but although giving similar results it adds little due to the larger uncertainties involved.
In the last couple of decades, northern high latitudes have warmed dramatically while the southern high latitudes have warmed very little if any. Forest’s approach will implicitly attribute this to a positive aerosol forcing over that period, in contrast to the negative forcing that would be expected given the increase in aerosol precursor emissions over that time.
Aerosol forcing estimation in the Forest/Lewis method is very stable and depends little on the periods used. Once the statistical methods in the Forest study are corrected, it produces a best estimate for aerosol forcing only 0.1 W/m² more negative using data ending in 1995 – almost two decades ago – as my study reaches using data extended to 2001. Over most of the diagnostic decades the surface temperature of the northern hemisphere was actually lower relative to that of the southern hemisphere than in the climatological (base ) period: the difference did not overtake the start of simulation period level until after 2001, and was particularly low in the decades to 1985 and 1995. So Steven’s objection is misplaced.
This leads to a very small estimate of the climate sensitivity, since if I understand correctly, the method will believe that aerosols were adding to CO2 forcing rather than opposing it as we would normally think based on independent evidence including satellite observations of aerosol forcing.
Steven does not understand correctly. Estimated aerosol forcing in my study is negative, as is usual. Moreover, the forcings used in the MIT model do not include that by Black carbon on snow and ice, which like aerosol forcing is concentrated in the northern hemisphere, and are stated in terms of average levels in the 1980s, since when as Steven says aerosol precursor emissions have risen. Those factors do not bias ECS estimation, but they do mean that my aerosol forcing best estimates need to be restated, adjusting them by about -0.2 W/m², to be comparable to aerosol forcing (ERF) estimates given in AR5. The so-adjusted aerosol forcing best estimates, of -0.5 W/m² using the Lewis diagnostics to 2001, and -0.6 W/m² using the Forest diagnostics to 1995, are well within the range of observationally-based satellite-instrument estimates cited in AR5.
The problem is that this interhemispheric warming difference since the 1980’s is almost certainly not aerosol-driven as the Forest/Lewis approach assumes. It is not fully understood but probably results from circulation changes in the deep ocean, unexpectedly strong ice and cloud feedbacks in the Arctic, meltwater effects around Antarctica, and/or the cooling effect of the ozone hole over Antarctica.
Indeed so, but as explained that has little or no impact on aerosol estimation in the Forest/Lewis studies. Incidentally, similar natural changes, in particular due to the AMO (which appears closely linked to quasi-periodic changes in ocean circulation) very probably accounted for at least part of the opposite changes in interhemispheric temperatures during the two decades up to the mid-1970s, rather than increasing aerosol forcing being responsible for the entire change in that period.
Most of these things are poorly or un-represented in climate models, especially the MIT GCM used by Forest and Lewis, and these models display too little natural decadal variability.
Agreed, but the decadal variability displayed by the MIT GCM is irrelevant. In fact averaging over an ensemble of simulations by it is used so as to reduce model variability. The estimates of decadal and other natural internal variability used in the Forest/Lewis studies come from full 3D AOGCMs. It may well be true that 3D AOGCMs also display too little internal variability, in which case my study’s uncertainty range for ECS may be too narrow. But that is not a reason to think that its ECS best estimate is biased.
It is thus not surprising that GCMs have great difficulty simulating the recently observed decadal swings in warming rate (including the so-called “haitus” period where they overestimate warming, and the previous decade where they typically underestimated it).
Indeed. However, the underlying problem is that GCMs seem to be oversensitive to forcing. GCMs only underestimated warming in the decade prior to the hiatus period if one takes that as starting in 1998, when real-world temperature were greatly boosted by the very exceptional 1997/98 El Nino, which was not included in the model simulations. If you take the hiatus period as starting any later than 1998, the average warming of the CMIP5 GCMs analysed in Forster et al (2013) JGR exceeded that in the real world. The 1991 Mount Pinatubo eruption distorts comparisons on a decadal basis; the twenty year period to the start of the hiatus offers a better comparison. Again, for all periods ending in 1999 onwards, the CMIP5 mean warms faster than the real world.
By implicitly attributing a pattern to aerosol that is probably due to other factors, Forest (and especially Lewis) are underestimating climate sensitivity. Other evidence such as the continued accumulation of heat in the worlds’ oceans is also inconsistent with the hypothesis that the slow warming rate in the last decade or two is due to negative feedback in the system as argued by Lewis.
Completely untrue. The continued accumulation of heat is not only perfectly compatible with a TCR of 1.35°C and ECS being (say) 1.75°C, it is actually implied by it. Surely Steven Sherwood must realise that? Upon substituting in Equation (1) of my guest blog using Equation (2), one obtains the relationship ΔQ = ΔT * F₂ₓ * (1/TCR – 1/ECS). This gives the increase ΔQ in ocean etc heat uptake between the base period (e.g., 1860-79) and the final period (e.g., 1998-2011) of an energy budget estimate as a function of the corresponding increase in global temperature (~0.75°C, the forcing F₂ₓ attributable to a doubling of CO₂ concentration (3.7 W/m²) and the values of TCR and ECS. Slotting in the numbers, this gives ΔQ = 0.75 * 3.7 /(1/1.35 – 1/1.75) = 0.47 W/m². Now, an estimate of ocean heat uptake over 1860-79 of about 0.25 W/m² can be obtained from Gregory et al (2013) GRL. Even discounting that by half to allow for their use of a sensitive model, the implied level of total heat uptake over 1998-2011 is 0.6 W/m², well up with observational estimates.
A more general problem with Lewis’ post is that he dismisses, for fairly aribtrary reasons, every study he disagrees with.
This is arm waving. I give specific reasons for dismissing each model. If Steven thinks any of them are wrong, I invite him to say so and to explain why.
Lewis dismisses climate models because they supposedly can’t simulate clouds properly, ignoring the multiple lines of evidence for positive cloud feedbacks articulated in Chapter 7 of the 2013 WGI IPCC report as well as the myriad studies (including my Nature paper from this year) showing that the models with the greatest problems are those simulating the lower climate sensitivities that Lewis favours, not the higher ones he is trying to discount.
Unfortunately, the “multiple lines of evidence for positive cloud feedbacks articulated in Chapter 7 of the 2013 WGI IPCC report” were not very persuasive to the AR5 scientists. As I wrote in my guest blog, “AR5 (Section 7.2.5.7) discussed attempts to constrain cloud feedback from observable aspects of present-day cloud but concluded that “there is no evidence of a robust link between any of the noted observables and the global feedback”.
Yes, some studies (including Steve Sherwood’s 2014 Nature paper) seem to show that certain specific features of the climate system are on the whole to be better/worse simulated by models that have higher/lower than average sensitivities. But it is a logical fallacy to think that implies higher sensitivity models correctly represent the climate system as a whole or that climate sensitivity is high. Moreover, the model simulations are often compared, not to observations, but to model-based reanalyses.
If we look at all the evidence in a fair and unbiased way, we find that climate sensitivity could still be either low or high, and that it is imperative to better understand the recent climate changes and the factors that drove them.
I have not claimed otherwise. I wrote that I thought it was unlikely – only 17% probability – that ECS (here effective climate sensitivity) exceeded circa 3°C. So I by no means rule out the possibility that it is higher. I entirely agree with Steven about the importance of better understanding climate changes and their causes.
Thanks Bart for again allowing me to take part in what has been an informed discourse. Unfortunately it seems this latest round of Q&A may not have lived up to the standard of previous posts as Nic’s latest post is riddled with errors in fact and framing.
To summarize, Nic’s reasoning appears to be that:
1. The mean planetary imbalance in nature is about 0.6 W/m2
2. The CCSM4 has an imbalance considerably higher than this (1.1 W/m2) and warms excessively in recent decades. This is a “failure”, and therefore it’s estimate of climate sensitivity should be dismissed.
3. The CESM1-CAM5 uses a forcing that is too strong and therefore it also should be dismissed.
4. CMIP5 models overestimate northward cross equatorial heat transport and thus effectively overestimate forcing in the northern hemisphere.
In reply:
1. I agree broadly with Nic’s estimate – the mean imbalance in nature for the ARGO period (2005-13) based on ocean heat content and other terms is about 0.65 W/m2 (global) with considerable uncertainty about that value – about 0.5 W/m2. I don’t agree with his assessment of ORAS4 and am happy to expand if desired, but I should note that the UKMO HADEN4 shows fundamentally the same basic signals as ORAS4 so perhaps Nic has a new technical manual to “study”.
2. Nic wonders why the CCSM4 simulations from the CMIP5 archive have a large imbalance and a large warming. The simple answer is that they don’t include any aerosol indirect effect and so they obviously shouldn’t be expected to replicate the observed temperature or energy imbalance records. It is an error in framing to suggest they should. This has no bearing on whether the model’s climate sensitivity is tenable.
3. Nic suggests that the CESM1-CAM5 indirect aerosol forcing is too high. In fact it is well within the AR5 estimated range. It’s not immediately clear to me where Nic gets the value of -0.7 W/m2 – is this the effective radiative forcing due to aerosol-radiation interactions he’s citing (which if so would again be within the IPCC range of –0.45 (–0.95 to +0.05) W/m2? Or is this the total effective radiative forcing due to aerosols which from AR5 is estimated at –0.9 (–1.9 to –0.1) W/m2. If the later, CESM1-CAM5 is actually about -1.5 W/m2 so, no, I don’t have concerns about the CESM1-CAM5 value and I don’t view it as a basis for discrediting the model’s sensitivity.
4. Nic raises a concern regarding CMIP5 simulated cross equatorial ocean heat transport. Notably this is a small value (a small residual of large mean hemispheric surface fluxes). Given the known problems with the ITCZ, both in the Pacific and Atlantic, in coupled models this is not at all surprising to me. Moreover the magnitude of the bias doesn’t relate in any systematic way to simulated climate sensitivity so far as I have been able to tell. Perhaps Nic has evidence to the contrary? If so, I would love to see that evidence. But perhaps the lack of any relationship is unsurprising, given that the ocean heat transport is not a forcing as Nic’s comments might lead one to believe.
Finally, regarding Nic’s assertion that “no sensible scientist would place his faith in NCAR CESM1-CAM5”, I wouldn’t presume to be the arbiter of such judgements. I can say that the NCAR CESM1-CAM5 is one of the best performing CGMs currently available (1) and the CESM family of models has been scrutinized by hundreds of studies using numerous in situ, reanalysis, and satellite datasets and various traditional and novel techniques. In my view, both the quality and sheer volume of scrutiny lies in start contrast to that given to Nic’s methods. I’ll leave it to the reader to judge which scientists are “sensible”.
References:
Knutti, R., D. Masson, and A. Gettelman (2013), Climate model genealogy: Generation CMIP5 and how we got there, Geophys. Res. Lett., 40, 1194–1199, doi:10.1002/grl.50256.
John claims that my recent post is “riddled with errors in fact and framing”. I will let readers form their own judgements on that claim after reading my below responses to John’s points.
1. I suggested a mean planetary imbalance of 0.5 W/m², in line inter alia with the Loeb et al (2012) estimate for 2001-2010, but I wouldn’t argue with 0.6 W/m². Taking the rather longer 1998-2011 period, over which the various observational datasets agree reasonably well on the change in ocean heat content (OHC), reduces the uncertainty in the annualised imbalance. Using the AR5 energy inventory data and uncertainty estimates gives a 5–95% range for the imbalance of 0.59 ± 0.19W/m² over 1998-2011.
We clearly have different views on the ORAS4 OHC reanalysis, but John has not produced any evidence that what I wrote was incorrect.
2. AR5 estimates the change in indirect aerosol forcing from the start of the CMIP5 historical simulations to 2011 at -0.35 W//m² (deducting ERF_ari per the AR5 SOD from ERF_ari+aci). A planetary imbalance of 0.6 W/m² represents 30% of mean 2002-11 forcing per AR5 (about 25% based on estimated changes in both variables since the first few decades of the instrumental period). So allowing for the omission of indirect aerosol forcing in CCSM4, using AR5 best estimates, would reduce its 1.1 W/m² imbalance by ~0.1 W/m², to 1.0 W/m², still well above the ~0.6 W/m² observational estimate. To my mind, this certainly suggests that CCSM4’s ECS is too high, although other explanations are possible.
3. I didn’t suggest that “the CESM1-CAM5 indirect aerosol forcing is too high”. What I wrote was: “The CESM1-CAM5.1 model’s aerosol forcing was diagnosed (Shindell et al, 2013) as strengthening by -0.7 W/m² more from 1850 to 2000 than per AR5′s best estimate.” This relates to total aerosol ERF, not to that from aerosol-radiation interactions (direct forcing) and the sources are as stated. Shindell et al, 2013 diagnosed total aerosol ERF as changing by -1.44 W/m² from 1850 to 2000; the change per AR5’s best estimate was 0.7 W/m² smaller at -0.74 W/m². My point was that CESM1-CAM5.1’s higher-than-AR5-best-estimate aerosol forcing enabled it to match actual warming from the 1920s to the early 2000s despite having a high ECS and TCR. If the model’s aerosol forcing had evolved in line with AR5’s best estimate, it would have simulated unrealistically fast warming.
4. The magnitude of cross-equatorial heat transport is of relevance to climate sensitivity since high sensitivity AOGCMs typically require fairly high aerosol forcing (more negative than per AR5’s best estimate) in order to reproduce the historical record of global surface warming. Since aerosol forcing is concentrated in the northern hemisphere (NH), if a model’s aerosol forcing is higher than the actual level then it would need a larger northwards cross-equatorial heat transport to maintain temperatures in the NH at a realistic level in relation to those in the southern temperature (SH). Atmospheric cross-equatorial heat transport appears to be both fairly modest (southward) and better constrained than that by the ocean, because of its effect on the position of the ITCZ, the inter-hemispheric temperature differential, etc.
It is in any event surprising how similar the ocean cross-equatorial heat transport of almost all CMIP5 models is, perhaps because (as I understand) most of them share a common ancestor ocean model.
I wasn’t suggesting that ocean heat transport is a forcing. My point was that if 0.6 PW too much is transported across the equator that is equivalent, in terms of the rate of energy input, to an excessive inter-hemispheric forcing differential of 4.8 W/m² (more accurately, 4.7 W/m²).
I wouldn’t dispute that NCAR CESM1-CAM5 is, according a quite a few metrics, one of the best performing CGMs currently available. But that does not, IMO, imply that its ECS and/or TCR are realistic. They might be accurate, but the observational evidence suggests that TCR at least – which is better constrained by observations than ECS – is unlikely to be as high as 2.3°C (e.g., Otto et al, 2103, Energy budget constraints on climate response. Nature Geoscience, 6, 415–416).
One study that has not been discussed here that belongs is that from Brian Rose (at UAlbany) and others in GRL this year. This, along with other studies, stresses the point (briefly mentioned by James in passing) that the global mean energy balance is a linear function of a time-evolving surface temperature field, rather than the global-mean temperature, and different spatial structures of warming can initiate different feedback responses, which ultimately limits the utility of inherently transient observations in constraining the equilibrium response.
In any case, I think the evidence is strong by now that limited observations do not constrain ECS as cleanly as larger and better-defined forcing periods like the LGM. Even with the issue of non-linearities, these periods can still be probed for information about the future (Gavin Schmidt’s paper with several coauthors on the marriage between models, paleo, and future, cited below) argues along these lines. The paleoclimate record is flatly incompatible with very low or very high sensitivities.
I am also sympathetic to Andrew’s argument in ballparking sensitivity on a feedback-by-feedback level, but it’s difficult to make this line of argument robust in a more quantitative fashion…especially since feedbacks influence each other.
Cited:
Rose, BEJ, K. Armour, D. Battisti, N. Feldl, D. Koll (2014), The dependence of transient climate sensitivity and radiative feedbacks on the spatial pattern of ocean heat uptake. Geophys. Res. Lett. 41, doi:10.1002/2013GL058955.
Schmidt, G.A., J.D. Annan, P.J. Bartlein, B.I. Cook, E. Guilyardi, J.C. Hargreaves, S.P. Harrison, M. Kageyama, A.N. LeGrande, B. Konecky, S. Lovejoy, M.E. Mann, V. Masson-Delmotte, C. Risi, D. Thompson, A. Timmermann, L.-B. Tremblay, and P. Yiou, 2014: Using paleo-climate comparisons to constrain future projections in CMIP5. Clim. Past, 10, 221-250, doi:10.5194/cp-10-221-2014.
Bart
Thank you for your latest, very relevant, questions. My answers are as follows.
1. You contrasted my statement, that over the period 1950-2013 CCSM4′s trend in simulated global surface temperature was nearly 85% higher than per HadCRUT4, with John’s statement that the decadal trends as simulated by the Community Earth System Model (CESM1-CAM5) of the National Centre for Atmospheric Research (NCAR) track quite closely with those derived from observations, referring to fig 2 in John’s guest blog.
These statements are not in fact contradictory. They relate to different model versions, and John’s statement relates to separate decadal trends in surface temperature, not to a single multidecadal trend.
My statement was based on a version of the CCSM4’s Historical/RCP4.5 simulation from the CMIP5 archive. It shows a linear trend in GMST of 0.197°C/decade over 1950-2013. That is 84% higher than the trend over the same period per HadCRUT4 of 0.107°C/decade.
A chart comparing surface temperature changes over 1850-2100 on the RCP8.5 scenario as simulated by CCSM4 and CESM1-CAM5 is shown in Hurrell et al (2013). Although CESM1-CAM5 has a higher ECS and TCR than CCSM4, its very highly negative aerosol forcing leads to its simulated temperature rise from 1850 not overtaking CCSM4’s until nearly the end of this century. Meehl et al (2013) give a more comprehensive comparison of projections by the two NCAR models.
2. This brings me on to point 5 of Bart’s summary, my assertion that NCAR CESM1-CAM5 model matches global actual warming reasonably well because the aerosol forcing was -0.7 W/m² more negative from 1850 to 2000 than the AR5′s best estimate (Shindell et al, 2013). Bart asks what I mean by ‘global actual warming’, and states that if I mean ‘global surface warming’ than my point 5 assertion seems to contradict points 2 and 3.
My assertion highlighted in Bart’s point 5 did refer to global surface warming. However, it related to the CESM1-CAM5 model, whereas my statements highlighted in Bart’s points 2 and 3 related to the CCSM4 model. These two model variants behave differently. CCSM4 has a lower ECS (2.9°C) and TCR (1.8°C) than CESM1-CAM5, which seems to have an ECS of 4.1°C and a TCR of 2.3°C. But CCSM4 does not simulate indirect aerosol forcing (aerosol-cloud interactions: ERF_aci). John says this means that its simulations in the CMIP5 archive shouldn’t be expected to replicate observed temperature records – or by implication future temperatures. These simulations do, however, form part of the ensemble used by the IPCC for projecting future temperatures, which is used for many purposes.
Although CCSM4 does not include indirect aerosol forcing, according to Lamarque et al (2011) its change in direct aerosol forcing from 1850-2000 was -0.81 W/m², in itself slightly higher that AR5’s best estimate of the change in total (direct + indirect) aerosol forcing over that period of -0.74 W/m².
Moreover, although Shindell et al (2013) diagnosed the 1850-2000 change in total aerosol forcing as -1.44 W/m² in CESM1-CAM5.1, Gettelman et al. (2012) note a total indirect effect of -1.3W/m² in CAM5 in 2000 compared to 1850. Although Gettelman et al. did not derive total aerosol forcing in CAM5, adding on to their -1.3W/m² indirect forcing the -0.8 W/m² direct forcing reported by Lamarque et al (2011) for CCSM4 would give a figure of -2.1W/m², much higher than Shindell diagnosed and outside the 5-95% uncertainty range given in AR5 despite that relating to changes since 1750.
3. Finally, Bart queries why I say that ‘observational evidence for cloud feedback being positive rather than negative is lacking’, pointing out that Steven Sherwood asserts that I have ignored ’the multiple lines of evidence for positive cloud feedbacks articulated in Chapter 7 of AR5′. The answer is simple. My concern is with the global level of overall cloud feedback and the observational evidence relating to it. Section 7.2.5.7 of AR5 “Observational constraints on Global Cloud Feedback’ deals with precisely this, discussing various approaches and citing many studies.
The first approach Section 7.2.5.7 discusses is to seek observable aspects of present-day cloud behaviour that reveal cloud feedback or some component thereof. Its conclusion: ‘In summary, there is no evidence of a robust link between any of the noted observables and the global feedback’; all it can point to is some apparent connections that are being studied further.
Section 7.2.5.7 then discusses attempts to derive global climate sensitivity from interannual relationships between global mean observations of TOA radiation and surface temperature, but notes studies contradicting the basic assumption of these attempts. It goes on to note all sorts of problems in finding acceptable cloud-response derived observational constraints on climate sensitivity, ending by stating ‘These sensitivities highlight the challenges facing any attempt to infer long-term cloud feedbacks from simple data analyses.’
References
Gettelman, A., H. and Coauthors, 2010: Global simulations of ice nucleation and ice supersaturation with an improved cloud scheme in the community atmosphere model. J. Geophys. Res., 115, D18216, doi:10.1029/2009JD013797.
Hurrell, J., and Coauthors, 2013: The Community Earth System Model: A Framework for Collaborative Research, Bull. Amer. Meteor. Soc., doi:
http://dx.doi.org/10.1175/BAMSWDW12W00121.1.
Lamarque, J.-F., and coauthors, 2011: Global and regional evolution of short-lived radiatively-active gases and aerosols in the representative concentration pathways. Climatic Change, 109, 191–212, doi:10.1007/s10584-011-0155-0.
Meehl GA et al (2013) Climate Change Projections in CESM1(CAM5) Compared to CCSM4. J Clim 26, 6287-6308
Shindell, D.T. et al, 2013. Radiative forcing in the ACCMIP historical and future climate simulations, Atmos. Chem. Phys., 13, 2939-2974
Dear Nic and John,
Thanks for your answers and clarifications.
Without recalling all the numbers, there seems to be a big difference in viewpoint on the crucial question whether a relatively large negative total aerosol forcing (i.e. in the lower part of the AR5 range from -1.9 to -0.1 W/m2) is necessary in CESM1-CAM5 and CCSM4 – and in GCMs in general – to replicate the observed increase in global surface warming since the beginning of the past century. John indicates that the total forcing of aerosols is about -1.5 W/m2 in CESM1-CAM5 which is in the AR5 uncertainty range and therefore he sees no reason to discredit it’s ECS value. Nic, however, points to the fact that although CCSM4 total aerosol forcing (which is equal to direct forcing in CCSM4 because it does not model the indirect component) is very close to AR5’s best estimate, this model shows too high surface temperatures, as also confirmed by John.
I invite John and Nic (and James!) to give a last reflection on this aerosol-issue.
Then a remark to Nic. You challenge Sherwoods’ claim regarding “multiple lines of evidence for positive cloud feedbacks articulated in Chapter 7 of the 2013 WGI IPCC report”. You base your judgement on a subparagraph in chapter 7 of AR5. However, the overall conclusion of the AR5 authors on cloud feedback is stated in the summary of chapter 7: “multiple lines of evidence now indicate positive feedback contributions from circulation-driven changes in both the height of high clouds and the latitudinal distribution of clouds” and further on in the summary: “The sign of the net radiative feedback due to all cloud types is less certain [than water vapor feedback] but likely positive” and is quantified as “+0.6 (−0.2 to +2.0) W/m2/°C”.
The question to Nic is whether he considers the overall cloud-feedback-conclusion of the AR5 authors to be wrong?
Bart.
I’d like to come back to something in Nic’s earlier comments, which is also relevant to this recent comment of Chris Colose. Nic, you seem to acknowledge the possibility of a nonlinearity, or perhaps equivalently, that the effective sensitivity under the moderate recent warming, is different to the equilibrium result. However, my understanding is that your calculation ignores this. Is this a fair summary of your position? Do you think the effect is small enough to ignore, or are you omitting it in principle (ie, only attempting to estimate the effective sensitivity)?
Just a final reflection on the aerosol issue per Bart’s suggestion. Nic has his values wrong. Please see Gettelman et al. 2012, Table 3 on page 8. The basic CAM5 is CAM5-LP: -1.36 W/m2 its the total effect, -1.11 W/m2 is the cloud effect and the residual (-0.25 W/m2) is the direct effect. The direct effect is NOT the -0.8 W/m2 that Nic seems to believe it is.
Gettelman, A., X. Liu, D. Barahona, U. Lohmann, and C. Chen (2012), Climate impacts of ice nucleation, J. Geophys. Res., 117, D20201, doi:10.1029/2012JD017950.
I can see the source of the discrepancy regarding CCSM4 forcing in the Lamarque et al. reference. The -0.81 figure quoted by Nic Lewis refers to clear-sky forcing. This is not the same as the all-sky forcing being discussed by others because it masks out cloudy areas from the analysis, and therefore doesn’t represent a global average. For example, Bellouin et al. 2008 found clear-sky aerosol forcing to be -1.3 W/m2, and used a simple translation to obtain an all-sky forcing of -0.65 W/m2, 50% of the clear-sky figure.
I thank Bart for raising the points about aerosol forcing and cloud feedbacks.
Regarding aerosol forcing in CCSM4 and CESM1-CAM5, I took my figures from Meehl et al (2012): Climate change projections in CESM1(CAM5) compared to CCSM4. That paper states about aerosol forcing:
“Gettelman et al. (2012b) note a total indirect effect of -1.3 W/m² in CESM1(CAM5) in 2000 compared to the preindustrial climate in 1850.”
Looking again at Gettelman et al (2012), it is not clear what figure Meehl et al. have taken, but it doesn’t actually seem to be the total aerosol indirect effect. John may be right that is the total aerosol forcing; alternatively it may be the shortwave indirect aerosol forcing with the ice offset applied again – Gettelman’s ice offset wording seems confusing. On the other hand, Meehl et al states that their Table 1 shows a reduction in the total aerosol indirect effect varying from +0.8 to +1.2 W/m² from 2005-2100, which is consistent with it being at least -1.3 W/m² in 2000. I have sought clarification.
Meehl et al (2012) also states, discussing global aerosol forcing from the direct effect reducing over the 21st century, that “Lamarque et al. (2011) indicate that this corresponds to a similar value of about 0.5 W/m² of additional forcing in CCSM4 that comes from a reduction of 60% in the direct anthropogenic cooling effects of aerosols”. That implies CCSM4’s direct aerosol forcing in 2000 was -0.8 W/m², which is why I gave that figure.
However, although Meehl et al evidently treated Lamarque’s total direct clear sky aerosol forcing of 0.81 W/m² – which Lamarque et al refer to as a global annual average – as being a global forcing value, Paul S suggests that this is in fact a figure for the clear sky area only. Whilst the figure that Gettelman gives for clear-sky shortwave radiation does appear to be a global radiative forcing figure, not a forcing over the clear-sky proportion of the total, I think Paul is right that Lamarque et al instead use the term to refer to forcing averaged over the clear sky, not over the whole globe. So, as John says, my -0.8 W/m² was mistaken.
My original argument, that the reason the CESM1-CAM5 model matches global actual warming reasonably well because the aerosol forcing was -0.7 W/m² more negative from 1850 to 2000 than the AR5′s best estimate, remains valid. That argument was based on CESM1-CAM5’s aerosol forcing as diagnosed by Shindell et al 2013, not on figures given by Meehl et al 2012.
More generally, higher negative aerosol forcing in CMIP5 models (relative to their base date) compared to AR5’s best estimates seems to be the most important reason why many CMIP5 models have, until the last decade or so, broadly matched the observed global warming over the instrumental period. The CMIP5 models’ median TCR of 1.8 °C is considerably above the TCR implied by comparing the observed rise in GMST with the change in AR5’s best estimate of total forcing and scaling their ratio by F₂ₓ (the forcing from a doubling of CO₂ concentration). Therefore, if total forcing in the CMIP5 models matched that estimated by AR5 up to now one would expect to have seen them considerably over-warming.
Regarding cloud feedbacks, as Bart says I base my claim on the lack of good observational evidence for overall cloud feedback being positive, as concluded in Section 7.2.5.7 of AR5 “Observational constraints on Global Cloud Feedback’. The multiple lines of evidence Steven Sherwood refers to relate just to individual types of cloud feedback: “feedback contributions”. There may well be other types of cloud feedback that are negative. The only consistent evidence for positive overall cloud feedbacks comes from GCM simulations. Although GCMs consistently show positive cloud feedback, as shown by Figure 3 in my guest blog CMIP5 GCMs have major errors even in something as basic as cloud fraction by latitude. Moreover, over almost all latitude bands there is a huge variation (including as to sign) in cloud feedbacks between different models, especially shortwave (see Fig. 3.d and 4.b of Zelinka & Hartmann, 2012)
I appreciate that Section 7.2.6 of AR5 quantifies overall cloud feedback as +0.6 with a 90% range of −0.2 to +2.0 W/m²/°C. That is based on the mean from GCMs and a widened version of the distribution of cloud feedback in GCMs. I do consider this conclusion to be wrong. In my view, it is not good scientific practice to assign a range for overall cloud feedback based on models when there is no solid observational evidence as to its value and models are known to be very far from perfect. The range given is, incidentally, at odds with the overall conclusion as to ECS in AR5, which assigns a 17% probability to ECS being less than 1.5°C. An ECS of under 1.5°C seem to require cloud feedback to be more negative than -0.2 W/m²/°C, which is only assigned a probability of 5%.
I will respond separately to James’ query.
References
Gettelman, A., X. Liu, D. Barahona, U. Lohmann, and C. Chen (2012), Climate impacts of ice nucleation, J. Geophys. Res., 117, D20201, doi:10.1029/2012JD017950.
Lamarque, J.-F., and coauthors, 2011: Global and regional evolution of short-lived radiatively-active gases and aerosols in the representative concentration pathways. Climatic Change, 109, 191–212, doi:10.1007/s10584-011-0155-0.
Meehl GA et al (2013) Climate Change Projections in CESM1(CAM5) Compared to CCSM4. J Clim 26, 6287-6308
Shindell, D.T. et al, 2013. Radiative forcing in the ACCMIP historical and future climate simulations, Atmos. Chem. Phys., 13, 2939-2974
Zelinka, M. D. and D. L. Hartmann, 2012: Climate Feedbacks and Their Implications for Poleward Energy Flux changes in a warming climate. Journal of Climate, 25, 608–624.
Dear Nic,
Before you posted your last comment I asked John for some clarification on his last comment. In this mail he wrote the following, which is relevant, especially regarding your claim high negative aerosol forcing in CMIP5 models is necessary to match the observed global warming over the instrumental period. John wrote:
Given the uncertainties in observations (e.g. ocean heat content) and arising from aerosol interactions, it is an open question as to what the “right value” [of the total aerosol forcing] is. There is considerable uncertainty. The notion that the cooling needs to be excessively “high” to match observations is not reality. The simple fact is that simulations using aerosol forcing and indirect effects within our observational range, when compared with the observational record of surface temperature and ocean heat content, do not constrain climate sensitivity to Nic’s values. Despite Nic’s protests, the CESM1-CAM5 is a very tenable simulation. I agree that this basic fact is problematic for reconciling his values with a vast body of work.
I invite participants to read more about it at:
Meehl, Gerald A., and Coauthors, 2013: Climate Change Projections in CESM1(CAM5) Compared to CCSM4. J. Climate, 26, 6287–6308.
doi: http://dx.doi.org/10.1175/JCLI-D-12-00572.1
Hurrell, James W., et al. “THE COMMUNITY EARTH SYSTEM MODEL.”Bulletin of the American Meteorological Society 94.9 (2013).
Bart.
Unfortunately CESM1 does not seem to have been included in the multi-model assessments such as Gillet et al and Stott et al, I’m assuming because its outputs were not available at the time. So it’s difficult to make any detailed statements regarding its performance in simulating recent climate change. However, eyeballing the output graph in Hurrell et al, it seems to indicate a current warming rate of almost 0.3C per decade. Is John really prepared to stand behind such an estimate? If that’s right, the real world already has about half a degree of catching up to do.
I am responding now to James’ query about effective climate sensitivity vs equilibrium climate sensitivity, and to the related part of Chris Colose’s comment.
My Journal of Climate objective Bayesian study did in principle estimate equilibrium sensitivity, since the settings of the parameter used to control sensitivity in the MIT 2D GCM were calibrated to equilibrium sensitivity. But my energy budget estimates based on AR5 forcing and heat uptake data do, as James says, estimate effective sensitivity and ignore the difference between that and equilibrium sensitivity.
The relationship between effective and equilibrium sensitivity varies between AOGCMs. The true equilibrium climate sensitivity is not known for most coupled CMIP5 models, and is usually taken from a ‘Gregory’ plot – the regression, typically for 140 years, of TOA radiative imbalance against GMST change following an abrupt 2x, or usually 4x, step increase in CO₂ concentration. One way of comparing effective and equilibrium sensitivity is to look at a model’s Gregory plot. If the regression line passes close to the initial point – the response is linear – then there is no indication of a material difference between effective and equilibrium sensitivity. Of the fifteen CMIP5 models for which Gregory plots are given in Andrews et al (2012), seven show almost perfectly linear behaviour, four show strongly non-linear behaviour, and four show fairly mild non-linearity. Virtually all the non-linearity is in the first few years; there is little evidence of sensitivity changing with the magnitude of forcing, at least up to a 4x increase in CO₂ concentration.
From a practical point of view, it more useful to know whether TCR, if correctly estimated from warming over the instrumental period (most of which has been in response to forcing over the last ~60 years), is likely to be a good guide to warming from now until the final decades of this century on scenarios with various changes in forcing. Allowance needs to be made for emerging warming-in-the-pipeline from past forcing when making such a TCR-based projection. What CMIP5 models suggest about the reasonableness of this method can be judged from how much warming during the second half of a 140 year model simulation in which CO₂ rises by 1% p.a. exceeds that in the first half. The below plot, Figure 1 from Tomassini et al (2013) shows the results of such an experiment for twelve CMIP5 models.
The amount of warming-in-the-pipeline after 70 years that will emerge over the second 70 years varies between models, but is probably ~0.4°C on average. About half the models show evidence of some increase in warming between the first and second 70 years in excess of 0.4°C. However, on average CMIP5 models show only a small degree of nonlinearity. Interestingly, CESM1-CAM5, although it warms faster than CCSM4, shows less evidence of non-linearity.
Who knows whether the real world will behave like any of the models? I think the IPCC scientists had it about right when they wrote in AR5 that the climate sensitivity measuring the climate feedbacks of the Earth system today “may be slightly different from the sensitivity of the Earth in a much warmer state on timescales of millennia”. Certainly, based on the results shown in Tomassini et al (2013) and the Gregory plots in Andrews et al (2012) it seems reasonable to ignore the difference between effective and equilibrium climate sensitivity when making projections over the rest of this century, at least.
Chris Colose referred to the Rose, Armour et al (2014) paper. Whilst interesting, it is based on artificial aqua-planet simulations with a mixed layer ocean. It is easier to relate time-varying effective sensitivity to the behaviour illustrated in the predecessor paper, Armour et al (2013), as that involves simulation by a CMIP5 model – actually CCSM4 – of the actual Earth, with a realistic ocean. In CCSM4, effective sensitivity increases over time, taking hundreds of years to approach equilibrium sensitivity. In simplified terms, the reason appears to be that ocean heat uptake (OHU) is stronger and more persistent – delaying the surface temperature rise – at latitudes where local sensitivity is higher. As the ocean in these regions eventually warms up then, because of the higher regional sensitivity, the surface temperature there has to rise further than the average elsewhere to compensate for the fall off in OHU. (This ignores important factors such as heat transport, and any variations in local feedbacks that occur as the pattern of OHU evolves.)
The prime region where this mechanism applies appears to be the Southern ocean, which at circa 50 degrees latitude absorbs heat particularly strongly and deeply. CCSM4 has a very high local sensitivity (very low climate feedback) at that latitude, as shown by the thick grey line in Figure 4 in Armour et al (2013):
However, the latitudinal pattern of feedbacks in CCSM4 is very different from most other CMIP5 models, as shown by Figure 3.f of Zelinka & Hartmann (2012), the thick black line being the multimodel mean:
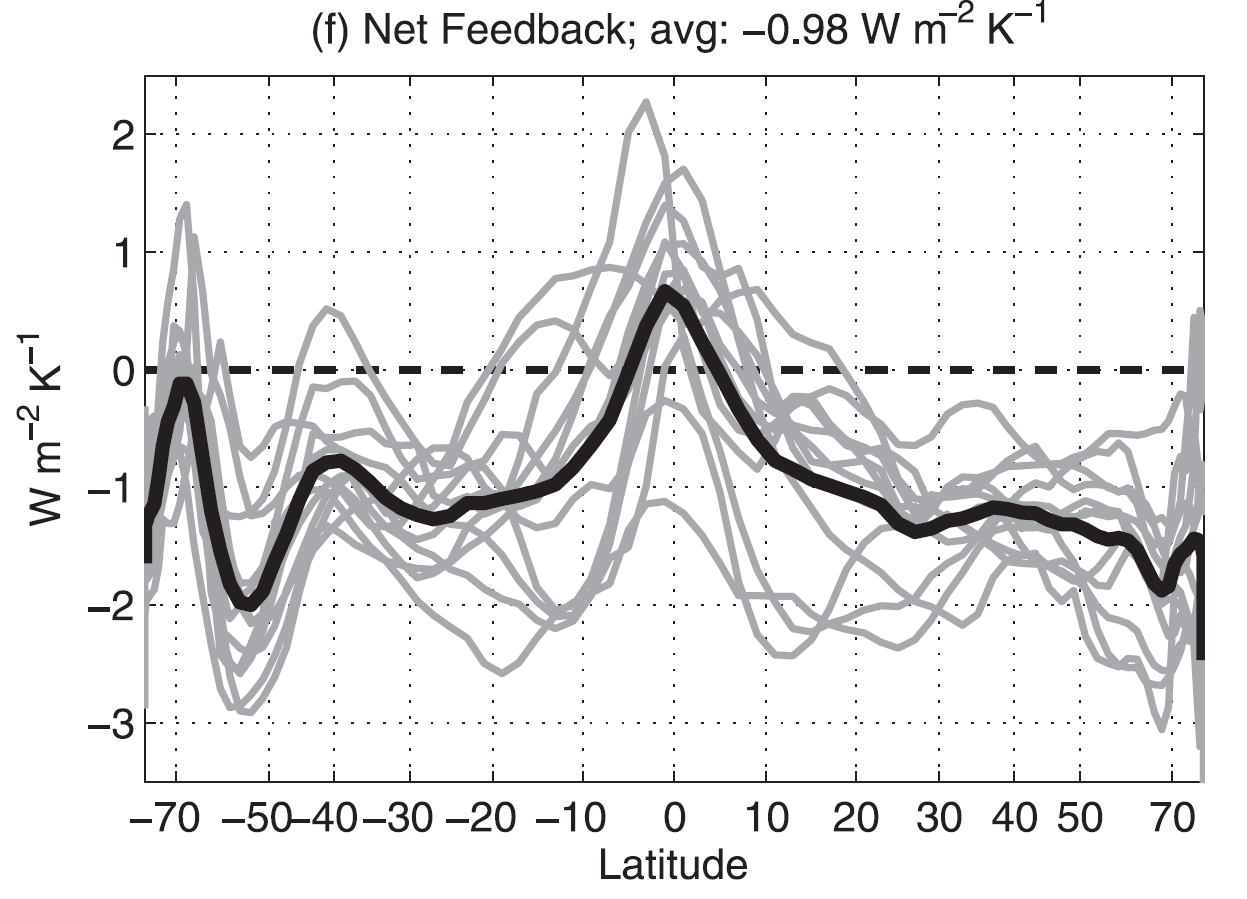
The Zelinka & Hartmann graph is based on global rather than local temperature changes, so feedback should be scaled down towards the poles (north in particular). Nevertheless, the latitudinal pattern of feedback strength in CCSM4 per Armour et al is pretty much the opposite of that of most other CMIP5 models, which inter alia appear to have a low local sensitivity around 50 degrees south. That does not imply CCSM4’s feedback pattern is less realistic than in other models. All models may have the feedback pattern materially wrong. But it does seem that Armour’s reasoning for why equilibrium climate sensitivity can be expected significantly to exceed effective sensitivity is very much model-specific.
References
Andrews, T., J. M. Gregory, M. J. Webb, and K. E. Taylor, 2012. Forcing, feedbacks and climate sensitivity in CMIP5 coupled atmosphere-ocean climate models, Geophys. Res. Lett., 39, doi:10.1029/2012GL051607.
Armour, K. C., C. M. Bitz, and G. H. Roe (2013), Time-varying climate sensitivity from regional feedbacks, J. Clim., 26, 4518–4534.
Rose, B. E. J., K. C. Armour, D. S. Battisti, N. Feldl, and D. D. B. Koll (2014), The dependence of transient climate sensitivity and radiative feedbacks on the spatial pattern of ocean heat uptake, Geophys. Res. Lett.,
Tomassini, L et al, 2013: The respective roles of surface temperature driven feedbacks and tropospheric adjustment to CO2 in CMIP5 transient climate simulations. Clim Dyn, DOI 10.1007/s00382-013-1682-3.
Zelinka, M. D. and D. L. Hartmann, 2012: Climate Feedbacks and Their Implications for Poleward Energy Flux changes in a warming climate. Journal of Climate, 25, 608–624.
Nic Lewis- Just FYI to your recent post.
John Marshall at MIT and several co-authors (including Kyle Armour) have some new work showing that delayed Antarctic warming relative to e.g., the Arctic, is moreso a consequence of advective process (owing to the nature of the local ocean circulation), rather than anomalous ocean heat uptake and storage.
http://oceans.mit.edu/JohnMarshall/papers/papers-in-progress/
A key point of the Rose paper is that the results cannot be understood in terms of a fixed feedback parameter vs. latitude, as shown in your plots, but that the local feedbacks themselves evolve in a rather robust fashion as the pattern of surface warming evolves in time.
Can someone please explain the concept of “back radiation”, i.e. electromagnetic radiation (power transfer) in a direction of more intense electromagnetic field strength, at any frequency? Such concept is in opposition to all of Jimmy Maxwell’s equations. Such concept is also in defiance of Gus Kirchhoff’s laws of thermal radiation.
In addition such flux, (power transfer) has never been observed, detected, or measured. Where does such fantasy originate and why?
With help of colleagues, I’ve been able to dig up the aerosol direct effects in CCSM4 for Nic. Meehl et al 2012 cite -0.45 W/m2 for sulfate and +0.14 W/m2 for the black carbon direct effect. They also have numbers for tropospheric ozone and organic carbon. For details, see Meehl et al 2012 linked below.
http://journals.ametsoc.org/doi/abs/10.1175/JCLI-D-11-00240.1
I also recall there was the suggestion in earlier posts that the CESM1-CAM5 is a closely related derivative of the CCSM4. In fact, the two models are very different, as described in the papers I sent along previously and also in the Gettelman et al. paper we published last year (Gettelman, A., J. E. Kay, J. T. Fasullo, 2013: Spatial Decomposition of Climate Feedbacks in the Community Earth System Model. J. Climate, 26, 3544-3561. doi: http://dx.doi.org/10.1175/JCLI-D-12-00497.1). Most of the cloud/convective schemes were rebuilt from the ground up and so aerosol forcing from one cannot be assumed to be the same as the other. The contribution from clouds in the midlatitudes to the increase in climate sensitivity from CCSM4 to CESM1-CAM5 was one of the surprising aspects of that study.
Dear Nic, John and James,
Let’s try to round off the discussion on aerosols and models.
For me, the crucial claim made by Nic in one of is his last posts is:
‘More generally, higher negative aerosol forcing in CMIP5 models compared to AR5′s best estimates seems to be the most important reason why many CMIP5 models have, until the last decade or so, broadly matched the observed global warming over the instrumental period.’
John’s reply to that was:
‘The notion that the cooling needs to be excessively “high” to match observations is not reality. The simple fact is that simulations using aerosol forcing and indirect effects within our observational range, when compared with the observational record of surface temperature and ocean heat content, do not constrain climate sensitivity to Nic’s values.’
@Nic: It is not clear to me how you draw this general conclusion. I went through several studies you referred to and there is one study – Shindell (2013) – that explicitly compares 7 models (including CESM1-CAM5.1) from CMIP 5 with respect to total aerosol forcing, summarized in figure 22 as follows:
This figure seems to confirm your claim that some (not many!) CMIP-5 models have higher negative aerosol forcing compared to AR5’s best estimate of -0.9 W/m2. However, the figure also shows that: “…there is an anti-correlation between historical aerosol RF and equilibrium climate sensitivity.”, which, in my eyes, seem to contradict the claim you make and seem to indicate there are (also) other reasons why these 7 CMIP5 models match observed global warming.
@John: it would be very helpful to me if you could elaborate a bit more on the interesting claim you make.
@James: I would like to ask to give your view on this matter.
Bart.
Reference
Shindell, D. T. et al, 2013. Radiative forcing in the ACCMIP historical and future climate simulations. Atmos. Chem. Phys. 13, 2939–2974
Dear Bart,
Thank you for your question about aerosols and models. You query my conclusion that “higher negative aerosol forcing in CMIP5 models compared to AR5′s best estimates seems to be the most important reason why many CMIP5 models have, until the last decade or so, broadly matched the observed global warming over the instrumental period.”
In that connection, you say that the figure in your comment ‘also shows that: “…there is an anti-correlation between historical aerosol RF and equilibrium climate sensitivity.”’
The figure in your comment actually relates Aerosol ERF (effective radiative forcing) to ECS. The phrase you quote from Shindell et al (2013) relates to a different panel of their figure 22 that shows, as per the quoted phrase, aerosol RF (radiative forcing), not aerosol ERF as in your figure. Aerosol RF (radiative forcing) gives a very incomplete picture of aerosol forcing; it is Aerosol ERF that is relevant to the surface temperature record.
My statement related simulated Historical warming, rather than ECS, in CMIP5 models to their Aerosol ERF. It is in any event TCR rather than ECS to which Historical warming ought to be related. However, for the CMIP5 models analysed in Forster et al 2013 the correlation between historical warming (to 2001-05, per Table 3) and TCR is only ~0.25.
I have computed the correlation between Historical warming and Aerosol ERF across all the CMIP5 models analysed in Forster et al 2013 for which Shindell et al 2013 gives Aerosol ERF estimates (including the additional ERF values in Table G2; I have assumed bcc-csm1-1-m has the same ERF as bcc-csm1-1).
The Historical warming vs Aerosol ERF correlation is very high – almost 0.9 – as shown in this figure:
The marker for CESM1(CAM5), which was not analysed in Forster et al 2013, would be almost on top of that for CSIRO-Mk3.6.0.
The observed warming from 1860-79 (which I believe to be the reference period used in Forster et al 2013) to 2001-05 is ~0.75°. Taking the average over the longer 1999-2007 period gives very similar warming. The Aerosol ERF for the best fit line through the points in the figure that corresponds to Historical warming of 0.75°C is about -1.1 W/m². By contrast, the AR5 best estimate for the increase in Aerosol ERF over the same period as that diagnosed in Shindell et al 2013 (1850 to 2000) is -0.75 W/m², some 0.35 W/m² less negative.
My earlier conclusion is fully supported by the foregoing analysis. What I wrote was aimed principally at the reasons why many CMIP5 models with TCRs close to or above the median level of 1.8°C simulated Historical warming no higher than that observed. Where a model has a TCR at or close to the lower level of 1.3–1.4°C implied by comparing observed historical warming with the change in forcing per AR5’s best estimates, one would not expect it to need stronger Aerosol ERF than per AR5’s best estimate in order approximately to match Historical warming.
As well as Aerosol ERF and TCR, the level of greenhouse gas and other forcings in CMIP5 models can also be an important factor in determining the historical warming it simulates. Although the ratio of greenhouse gas forcing in 2001-05 to the forcing from a doubling of CO₂ concentration for the CMIP5 models diagnosed in Forster et al 2013 is on average close to the AR5 best estimate level of 0.69, it varies from 0.52 to 0.97.
CMIP5 models also may simulate a lower level of Historical warming than would be expected from their TCRs and Aerosol ERF levels because the analysis of a (small) sample of CMIP5 models in Shindell et al (2014) indicates that they exhibit a substantially higher transient sensitivity to Aerosol (and Ozone) ERF than to greenhouse gas ERF. That is not because the efficacies of those forcings (which relates to the equilibrium response) exceeds – whether due to inhomogeneous distribution or otherwise – one, as claimed in Kummer & Dessler (2014). See, e.g., Hansen et al, 2005. Rather, it is because more of the Aerosol and Ozone ERF is concentrated in the northern hemisphere middle-to-high latitudes, where the temperature response is not only stronger, but more rapid, than average. Shindell’s analysis is valid in principle in the real world as well as in model-simulated worlds. However, the difference between estimated middle-to-high latitude total forcing in the northern and southern hemispheres is quite small. Based on an observational estimate of the ratio of transient climate sensitivity for the northern hemisphere middle-to-high latitudes relative to that globally (Crowley et al, 2014), this points to the effect being minor. Observational estimates of TCR using a global approach are probably just a few percent too low, at least if TCR and ECS are moderate.
As my figure shows, there is a large spread in simulated Historical warming to 2001-05, but by then the models analysed were on average simulating a significantly greater rise in surface temperature than observed. Although my analysis only relates to the subset of the CMIP5 models analysed in Forster et al 2013 for which Aerosol ERF data was available to me, their average Historical warming is in line with that for all the Forster et al 2013 models.
John’s statement that ‘simulations using aerosol forcing and indirect effects within our observational range, when compared with the observational record of surface temperature and ocean heat content, do not constrain climate sensitivity to Nic’s values’ is consistent with my conclusion. The key phrase is ‘ aerosol forcing and indirect effects within our observational range’. The observational range for Aerosol ERF is very wide. John made this point himself, writing:
‘Given the uncertainties in observations (e.g. ocean heat content) and arising from aerosol interactions, it is an open question as to what the “right value” [of the total aerosol forcing] is.’
Uncertainty in [total] aerosol ERF is the main problem preventing observational ECS and TCR estimates from being better constrained. There is also uncertainty in observed ocean heat content, but that is considerably smaller, and of direct relevance principally to observational estimates of ECS.
I will end by reiterating the final conclusion of Schwartz et al (2010), which remains true:
‘The principal limitation to empirical determination of climate sensitivity or to the evaluation of the performance of climate models over the period of instrumental measurements is the present uncertainty in forcing by anthropogenic aerosols. This situation calls for greatly enhanced efforts to reduce this uncertainty.’
References
Crowley TJ, SP Obrochta and L Liu, 2014. Recent Global Temperature ‘Plateau’ in Context of a New Proxy Reconstruction.Earth’s Future DOI: 10.1002/2013EF000216
Forster, P. M., T. Andrews, P. Good, J. M. Gregory, L. S. Jackson, and M. Zelinka, 2013. Evaluating adjusted forcing and model spread for historical and future scenarios in the CMIP5 generation of climate models. Journal of Geophysical Research, 118, 1139–1150.
Hansen J et al, 2005. Efficacy of climate forcings. Journal of Geophysical Research, 110, D18104
Kummer JR and AE Dessler, 2014. The impact of forcing efficacy on the equilibrium climate sensitivity. Geophysical Research Letters.
Schwartz, SE et al, 2010. Why Hasn’t Earth Warmed as Much as Expected? Journal of Climate, 23, 2453-2464
Shindell, D. T. et al, 2013. Radiative forcing in the ACCMIP historical and future climate simulations. Atmos. Chem. Phys. 13, 2939–2974
Shindell D.T., 2014. Inhomogeneous forcing and transient climate sensitivity, Nature Climate Change, vol. 4, pp. 274-277.
Dear Nic,
Just a short questions for clarification:
On may 15 you write: “If aerosol was known to have a current ERF of -0.9 W/m², in line with AR5′s best estimate…”
On may 21: “AR5′s best estimate of the change in total (direct + indirect) aerosol forcing over that period of -0.74 W/m².”
On may 27: “the AR5 best estimate for the increase in Aerosol ERF over …1850 to 2000 is -0.75 W/m²”
What explains the difference in numbers? Different periods?
Bart.
Nic,
It is nice to see that we have found common ground regarding the major challenge posed by uncertainty in aerosol radiative effects in trying to constrain climate sensitivity using methods aimed at fitting either the global surface temperature or ocean heat content records. I find your reference to Schwartz et al. 2010 to be spot on, though I must acknowledge that, as Steve is a good friend, I may be somewhat biased. Steve has built upon this work in Schwartz et al. 2012.
Still your citation of Steve’s work, and seeming embrace of it, leaves me wondering why you are so comfortable rejecting what I see as its major finding. In his 2012 paper he concludes that: “Equilibrium sensitivities determined by two methods that account for the rate of planetary heat uptake range from 0.31 ± 0.02 to 1.32 ± 0.31 K (W m-2)-1 (CO2 doubling temperature 1.16 ± 0.09 to 4.9 ± 1.2 K), more than spanning the IPCC estimated “likely” uncertainty range”? This was a fundamental point I made in my original post (Schwartz et al 2012 was citation #1).
Do you have a basis for rejecting this key finding of Schwartz et al. 2012?
From my point of view, its conclusion underscores the need to fully explore complementary approaches to the problem, such as the “first principles” approach of feedback analysis in GCMs, among others.
John
Schwartz, Stephen E. “Determination of Earth’s transient and equilibrium climate sensitivities from observations over the twentieth century: strong dependence on assumed forcing.” Surveys in geophysics 33.3-4 (2012): 745-777.
A few general thoughts on aerosol forcing:
1) Backing up what I believe is Nic Lewis’ general theme, aerosol forcing in some models, and in AR5 chapter7/8, is a substantial contributor to total net anthropogenic forcing. There is also a large spread of net aerosol forcing across the CMIP5 model ensemble (something like -0.3 to -1.6 W/m2, about 10 – 60% of net non-aerosol forcing). All else being equal a greater negative net aerosol forcing will reduce the amount of warming produced by the model, so aerosol forcing can be considered a major contributor to the spread of simulated historical warming amounts in the CMIP5 ensemble. Another major contributor is sensitivity. If we can get a decent idea of the correct aerosol forcing that should lead to narrowing of uncertainty on sensitivity.
2) How seriously should we take the best estimate for aerosol forcing given in AR5? I think it’s worth stating that the confidence level for forcing estimates of aerosol-cloud interactions RFaci and ERFaci are given as ‘low’ and ‘very low’. DirectRF or RFari was elevated to ‘high’ confidence though the uncertainty range expanded compared to AR4, and ERFari was given as ‘low’.
There is of course evidence which points to a total net aerosol forcing of about -0.9W/m2, but given these confidence levels the word “about” should be a major part of interpreting such a statement. I don’t think it’s realistic to regard -1.0, -1.1 or perhaps even -1.2W/m2, for example, as much less likely than -0.9, or to definitively discount larger (more negative) aerosol forcing as too high. That’s at least my reading of the relevant AR5 chapters taken as a whole.
3) Model (and observational, for that matter) aerosol forcing estimates can be a minefield, as earlier portions of this discussion can attest. One issue relevant to recent discussion here is timescale.
Modelling groups involved with the ACCMIP project submitted a set of time slice simulations representing anthropogenic aerosol emissions at various moments. They used the difference between the 2000 and 1850 time slice simulations to represent the aerosol forcing of the models. Since the IPCC AR5 forcing estimate uses 1750 as base year an adjustment is required for a like-for-like comparison.
Numbers for CMIP5 models not involved in ACCMIP (which is the majority) tend to be calculated by comparison between sstClimAerosol and sstClim simulations. sstClimAerosol is equivalent to the ACCMIP 2000 experiment, but sstClim is not equivalent to ACCMIP 1850 because it doesn’t include any anthropogenic aerosol emissions at all. That means the result represents the absolute anthropogenic aerosol forcing, as opposed to being relative to a particular moment.
Relevant to Bart’s recent question the different values given by Nic Lewis are indeed referencing different periods, -0.75 for 1850-present and -0.9 for 1750-present, according to the forcing profile presented in AR5 Chapter 8. As described above it is correct to use the 1850 figure to compare with ACCMIP numbers, but it is not correct for comparing with numbers for all other models. If anything the AR5 estimate requires a small adjustment the other way for comparison. Unfortunately AR5 chapter 7 makes the same error/confusion by listing ACCMIP and sstClimAerosol-sstClim results in the same table under the banner of 1850-2000 forcing.
—————————————-
One minor point of pedantry:
There are actually 3 versions of the GISS-E2-R model included in CMIP5 and aerosol setup/forcing is the key difference between the versions. It’s not entirely clear which, if any, of these was used in the ACCMIP submissions to produce the stated -1.1W/m2 forcing but if I had to guess it would be version 2, which produces about 0.7ºC warming in the historical run.
I’d like to just follow up/respond on a few points before responding to Bart’s and John’s latest.
First, Andrew Gettelman (to whom Jerry Meehl referred my question) has confirmed that the 1.3 W/m² total indirect effect aerosol forcing in CESM1(CAM5) statement in Meehl et al (2013) was erroneous, and it should be closer to 1.1 W/m², with total aerosol effects (direct + indirect) about 1.4 to 1.5 W/m². That is in line with the figure of 1.44 W/m² per Shindell et al (2012) that I used originally.
Secondly, I have a couple of observations on Chris Colose’s comments that “In any case, I think the evidence is strong by now that limited observations do not constrain ECS as cleanly as larger and better-defined forcing periods like the LGM.” and “The paleoclimate record is flatly incompatible with very low or very high sensitivities.”
Donald Rapp, in his comment takes the opposite view about how well the LGM constrains ECS, and gives a link to his detailed analysis that comes up with a range of ECS values from the LGM – preindustrial transition varying from 1.3°C to 2.8°C.
Moreover, whilst the 1°C to 6°C ECS range that the AR5 authors decided paleoclimate evidence in its entirety supported suggests ECS is most unlikely to be under 1°C, it provides little evidence against ECS being between 1.5°C and 2°C. (I have checked this by deriving an appropriate likelihood function for the paleo evidence and undertaking an objective statistical analysis based on combining that likelihood function with one erived from warming over the instrumental period.)
Chris also states:
“A key point of the Rose paper is that the results cannot be understood in terms of a fixed feedback parameter vs. latitude, as shown in your plots, but that the local feedbacks themselves evolve in a rather robust fashion as the pattern of surface warming evolves in time.”
I have re-read Rose et al (2014) but do not see where Chris gets his assertion from. The paper states that doubling CO₂ and imposing ocean heat uptake either tropically or at high latitudes all excite different feedback patterns. But I can see no claim that those patterns evolve over time. So far as I am aware, the two feedback plots I compared both involved a forcing increase, primarily from greenhouse gases, and the ocean heat uptake resulting therefrom. So I would have thought they were broadly comparable (apart from one measuring feedback relative to local, and the other to global, surface temperature).
Finally, I thank Chris Colose for the pointer to the new paper by John Marshall et al. Chris writes
“delayed Antarctic warming relative to e.g., the Arctic, is moreso a consequence of advective process (owing to the nature of the local ocean circulation), rather than anomalous ocean heat uptake and storage.”
I assume that is intended to contrast with my statement that:
“the Southern ocean, which at circa 50 degrees latitude absorbs heat particularly strongly and deeply”.
Figures 4.a and 6 of Marshall et al show strong heat absorption in the Southern Ocean, peaking between 50°S and 60°S. I agree that only part of this is stored locally, with much of it getting advected away. But for the argument I was making I don’t think it matters what proportion of the heat is advected away rather than stored locally at depth.
References
Marshall J et al, 2014. The ocean’s role in polar climate change- asymmetric Arctic and Antarctic responses to greenhouse gas and ozone forcing. Phil. Trans. R. Soc. A 372: 20130040. http://dx.doi.org/10.1098/rsta.2013.0040
Meehl G A et al, 2013. Climate Change Projections in CESM1(CAM5) Compared to CCSM4. J Clim 26, 6287-6308
Rose B E J et al, 2014. The dependence of transient climate sensitivity and radiative feedbacks on the spatial pattern of ocean heat uptake. Geophys. Res. Lett., 41, doi:10.1002/2013GL058955.
Shindell, D T et al, 2013. Radiative forcing in the ACCMIP historical and future climate simulations. Atmos. Chem. Phys. 13, 2939–2974
Dear Bart,
You query my different figures for the AR5 best estimate of aerosol forcing. As you suspect, the reason is mainly different periods. The current ERF of -0.9 W/m² is for 2011, the most recent year for which AR5 gives forcing data, relative to 1750, representing preindustrial conditions, where all the AR5 forcing data are set at zero.
The period I referred to on 21 May was 1850 to 2000, the same as for the figure I was comparing it with. Between those years AR5’s best estimate of aerosol ERF changed by -0.744 W/m², which I rounded to -0.74 W/m². Early today, I instead rounded the same 1850 to 2000 figure to -0.75 W/m², which looks a little less unrealistically precise than -0.74 W/m².
Nic
John,
Thanks for your query. I do indeed in general embrace Steve Schwartz’s work, including almost all of his 2012 paper. In my guest blog, I accepted the range and median from its five TCR estimates, but I rejected one of its five ECS estimates, writing:
“Schwartz (2012) – The upper, 3.0–6.1°C, part of its ECS range derives from a poor quality regression using one of six alternative forcings datasets; the study concluded that dataset was inconsistent with the underlying energy balance model.”
For those not familiar with the study, Steve takes six forcing data sets and for each regresses, with zero intercept, surface temperature anomalies on forcing, both with and without the planetary heating rate deducted. The ECS estimates in its abstract are derived from the regressions of temperature change on forcing alone, together with estimated heat uptake coefficients.
One of Steve’s forcing data sets (Myhre) gives results for both regressions that are inconsistent with linear proportionality between temperature change and forcing, and is not used to estimate TCR and ECS. Another of the data sets (MIROC) does so for the regression with the planetary heating rate deducted. Its regression of temperature change on forcing has an R² of only 0.29, far lower than for the remaining four datasets (R² from 0.54 to 0.78). Its ECS estimate of 1.32 K/(W/ m²) is well out of line with the range of 0.31–0.74 K/(W/ m²) for the four datasets with good quality regressions.
I consider it justifiable in the circumstances to reject the realism of the ECS estimate using the MIROC data set. Doing so reduces the ECS range, at 5-95% uncertainty about the outer estimates, to 1.1–3.1 K. However, that range does not sample all the uncertainty in forcing, temperature change and heat uptake rates.
I agree that the analysis of feedbacks in GCMs is worthwhile. But there is a fundamental limitation, in that feedback or adjustment mechanisms not included in any model physics will not show up. Since cloud behaviour is parameterized rather than being represented at a basic physics level, this is in my view by no means unlikely. Moreover, the idea that the range of feedback values exhibited by existing GCMs represents a statistically valid uncertainty range seems strange to me. It is possible that for some processes represented in parameterized form, some combination of parameter settings outside those used in any GCM would produce quite different, and more realistic, feedbacks. The high dimensionality of parameter space makes searching it for good parameter combinations very difficult. Whilst a good GCM is very useful and may reproduce well many aspects of the real climate, in a final analysis only observations of the real world climate system can show how it actually behaves.
This enlightening dialogue has prompted me to offer first a specific and then a general comment.
First, I’m impressed with the call by Nic Lewis and John Fasullo for more effort focused on reducing the broad uncertainties surrounding aerosol forcing. Better accuracy should be most critical for TCR estimates based on regressing temperature change on forcing change. Aerosol forcing is of course also relevant to equilibrium sensitivity estimates, but these depend on many additional variables that are also uncertain. TCR is of particular relevance to changes expected over the remainder of this century (but see below).
Estimating the equilibrium temperature change resulting from a CO2 doubling most appropriately incorporates all relevant feedbacks. These include not only short term feedbacks such as changes in water vapor, lapse rate, clouds, albedo, and the modifying effects of varying atmospheric and ocean circulation patterns, but also longer term changes in ice sheets, dust/vegetation, and the carbon cycle. A strong dichotomy is sometimes assumed between the short and long term responses, but it is likely that every feedback follows its own time course, with no absolute dividing line. Curiously, these estimates have been termed “Earth System Sensitivity” rather than “equilibrium climate sensitivity” (ECS), with the term ECS misapplied, in my view, to three other types of sensitivity estimation that exclude the longer term responses from the feedback calculations. These three types may be of more immediate practical importance, but they are not true equilibrium estimates. I’ll refer to them as EFS, PCS, and FCS. The term “EFS” may already be familiar, but PCS and FCS are terms of convenience I’ve conjured up for this discussion, and may not have been used before in this context.
“Effective climate sensitivity” (EFS) attempts to derive a value for equilibrium temperature change from observations made under non-equilibrium conditions, based on an energy balance model relating changes in planetary energy imbalance N to those in forcing F and radiative restoring (the increase in heat loss to space from a surface temperature rise): N = F – λ ΔT, where the feedback parameter λ quantities the rate of increased heat loss per K warming. At equilibrium (N = 0), the temperature change is given as F/ λ, which for doubled CO2 is about 3.7/ λ. The equation is well known, but my point here is that it asks a specific question – If λ is constant, so that λ calculated from non-equilibrium data is the same as λ at equilibrium, what would the equilibrium temperature change be? In other words, EFS is a hypothesis about the consequences of a constant λ. Typical values have been in the range of 2 C. I should add that the range is broad due to uncertainties about the forcings, and broad uncertainty is also true for the values of PCS and FCS discussed below, but I prefer to leave that challenge to a different discussion.
PFS (my abbreviation for paleoclimate sensitivity) asks a different question. If the forcings from an earlier era (typically the LGM) can be used as surrogates for forcing due to doubled CO2, what temperature change would that allow us to predict for doubled CO2? PFS is a hypothesis about the relevance of changes under a different climate involving different forcings to our current climate forced by CO2. Typical values are closer to 3 C, again with substantial uncertainty.
FCS is my convenience term for “Feedback-based Climate Sensitivity” derived from “bottom up” models incorporating the short term feedbacks I cited above. It hypothesizes that these accurately capture the entirely of climate behavior (minus the long term feedbacks) in response to CO2 forcing, despite the known weaknesses of current GCMs. Typical values also tend to center around 3 C.
If I were to attempt a single assertion to illustrate the essence of what I’m describing, it would be that each one of these estimates, despite differing among themselves, might in theory be largely correct, because they each estimate something different – i.e., they each test a different hypothesis, which in none of the cases actually involves the true equilibrium response that Earth System Sensitivity aims for.
The most apparent disparity is between EFS and the other two metrics. Inaccurate forcing estimates may play a role in the disparity, but there is also reason to conclude that EFS, in hypothesizing a constant λ, is not accurately representing the real world evolution of climate responses to an imposed forcing. A number of groups have reported model-based evidence for the variation of λ with time and temperature in a downward direction, signifying an increasing value calculated for equilibrium temperature change. The very recent article by Rose et al that Chris Colose mentioned above suggests that it may be impossible even to evaluate the relationship between EFS and the other metrics on the basis of current evidence – transient sensitivity and ocean heat uptake. I don’t presume to judge the weight of evidence. Rather, I would suggest that since neither EFS nor PCS nor FCS is a true ECS and since none of them is necessarily addressing the same hypothesis as the others, their differences should be acknowledged. Specifically, a logical default position for entities that may not be identical would be, I suggest, that we not call them all by the same name, since that prejudges the issue.
This seems particularly relevant to recent literature, which increasingly has looked at EFS. The values are lower than values estimated for PCS and FCS, and this has led some to suggest that the latter two estimates are wrong. That may or may not be the case, but if it’s a case to be made, it should be done explicitly based on evidence, and not implicitly through the use of identical names for the different estimates.
Thanks Nic for addressing the question I raised regarding Schwartz 2012. While I can appreciate your desire to establish a basis for excluding some of the forcing datasets used by Steve (to narrow the resultant range of ECS), I find the argument you present for doing so to contain the same lack of questioning of basic assumptions that I’ve identified in your other work. It is based on what you “believe” is the right value of R2 for the relationship between forcing and temperature, and that it should be high. In fact, we know very well from both GCMs and observations that surface temperature and forcing need not correlate strongly at all, and that their degree of correlation over any finite and therefore transient record can be strongly positive, weak, or even negative as a consequence of internal variability. In fact, your assumed “constraint” is therefore inappropriate and needs to be thought through more carefully in my view. Perhaps you could use a GCM ensemble for doing so?
It also concerns me that your reasoning is circular. That is, you evaluate the forcing datasets with inappropriate expectations based on the temperature record (discussed above) and then use that screened subset of forcing to make conclusions regarding the same temperature record that what was used for the screening. In the end, all you’re left with is confirmations related to your initial assumptions and biases. In my view, any valid screening of forcing datasets should be divorced entirely from assumptions regarding how it should relate to the temperature record. Screening criteria need instead to be based on the suitability of the data and methods used in creating the forcing data itself. As such, there is no basis for narrowing the range of uncertainty presented by Schwartz et al. 2012 and as I see it, that uncertainty range stands.
On GCMs, I wonder what large negative feedback you might envision that is “not included in any model physics”? To me, it seems more like wishful thinking than informed conjecture. I too wish it were so – but I see no evidence that it is. On clouds, you seem to ignore the vast body of work aimed at bridging the scale separation between GCM and microphysical scales. There is a considerable body of work focusing on cloud resolving models and large eddy simulation that addresses the very gap you cite as cause for concern. Yet this work fails to reveal the potential for strong negative cloud feedbacks that you cite. Collectively I think this work pours cold water on any hopes for a low climate sensitivity. I think as well that documentation of the latest generation of GCMs (see again Hurrell et al. 2013 BAMS) shows just how remarkably GCMs have evolved. In the process they have pointed towards the higher end of the sensitivity range. (Along these lines, please see the paper by Su et al. 2014 that just came out further supporting the conclusions of Fasullo and Trenberth 2012).
Lastly, (per Bart’s prodding to provide greater clarity to readers) I’d like to elaborate a bit on what I referred to earlier as a viable means for testing the basic assumptions of your (and related) statistical methods. Using the same input fields that you currently use (from instrumental records) but based on model output, we can examine the degree to which this method solves for the known climate model’s sensitivity. With a centuries-long control run where the only forcing considered is CO2, I suspect your method will work quite well. But what is the sensitivity to multiple forcings and internal variability? In fact, for the most part, we don’t know. But using a multi-member model ensemble, such sensitivities should be able to be clearly quantified. Will such methods provide an accurate and precise estimate of the underlying model’s climate sensitivity? Or will large errors result due to the inherent limitations of simple statistical models in assessing a complex dynamical system? These are the questions we are currently exploring and we expect concrete answers in the not-so-distant future.
Su, H., J. H. Jiang, C. Zhai, T. J. Shen, J. D. Neelin, G. L. Stephens, and Y. L. Yung (2014),Weakening and strengthening structures in the Hadley Circulation change under global warming and implications for cloud response and climate sensitivity, J. Geophys. Res. Atmos., 119, doi:10.1002/2014JD021642.
Thank you, John, for responding to my explanation regarding Schwartz (2012). I will comment on that before responding to your other comments.
Schwartz (2012)
You exaggerate by claiming that I established a basis for excluding “some” of the forcing datasets used by Steve Schwartz. I only did so for a single forcing dataset (MIROC), and for ECS estimation by one method only. Steve himself had already excluded another forcing dataset (Myhre) for both ECS and TCR estimation, and had excluded the same forcing dataset as I did for ECS estimation by the other method.
You claim that forcing and surface temperature change need not correlate strongly at all. But Steve’s study is based on a simple model in which these variables do exhibit linear proportionality. So you are in effect rejecting the basis of Steve’s paper. Indeed, I wonder if you are actually rejecting the entire basis of estimating TCR and/or (effective) climate sensitivity from the warming observed over the instrumental period. That would be an extreme position and not, I hope, one that many climate scientists would support.
Contrary to what you suggest, Steve does find that “a rather robust linear proportionality is exhibited for most of the forcing data sets between surface temperature and forcing, but with different slopes”, although he finds that not to be so for the Myhre forcing data set. For the MIROC dataset he finds a reasonably strong relationship between surface temperature and forcing (R² of 0.47) when the regression is not constrained to pass through the origin, but (unlike for the other datasets, excluding Myhre) a much weaker relationship (R² of 0.29) when it is so constrained.
Steve wrote “The fraction of the variance in the temperature data accounted for by the regression forced through the origin is over 50% for four of the six forcing data sets. For most of the data sets, the intercept is near zero; constraining the regression line to pass through the origin results in little decrease in the fraction of the variance in the data accounted for by the regression”. He goes on to say that “A high correlation with zero intercept, that is, temperature anomaly proportional to forcing, is consistent with a planetary heating rate N that is likewise proportional to the temperature increase.”
Since for the MIROC dataset the correlation with zero intercept is low, in its case the relationship of temperature with forcing does not support the planetary heating rate being proportional to the temperature increase. Yet the assumption that the heating rate is so proportional is used to estimate ECS (although not TCR) from the MIROC dataset. The combination of the low correlation with zero intercept using the MIROC forcing dataset and the indication that that dataset is not consistent with the method used to derive ECS from it seems to me valid grounds for excluding that estimate. I note that in, relation to the MIROC forcing dataset, Steve wrote that the departure from linear proportionality, together with the observations of increase in temperature and planetary heating rate, are inconsistent with an energy balance model for which the change in net emitted irradiance at the top of the atmosphere is proportional to the increase in surface temperature – the model that underlies his study.
Contrary to your claim, my reasoning – which is close to Steve’s – is not circular. And it has nothing to do with bias – the basis for rejecting a forcing dataset is not related to whether the ECS estimate it produces is high or low. You might contend – although I would not – that the linear proportionality assumption made by Steve between changes in forcing, surface temperature and planetary heat uptake is unjustified even as an approximation over periods of under a century. But, having made that assumption, I think it is right to follow it through. Nevertheless, I don’t think a multiple-forcing-dataset regression approach is the best way to estimate TCR and ECS from observed warming over the instrumental period. As I wrote, the 1.1–3.1 K range for ECS given by Steve’s study if the MIROC dataset is excluded does not sample all the uncertainty in forcing, temperature change and heat uptake rates. I disregarded that range in my guest blog (and in the Lewis/Crok report). But if, as you seem to do, you reject the simple proportionality model underlying Steve’s study then you should certainly not conclude – as you do – that his uncertainty range stands.
As you say, internal variability affects the relationship between changes in surface temperature and forcing. There are also substantial uncertainties in forcing, especially aerosol forcing. Long AOGCM control runs are very useful for providing estimates of internal variability, in ocean heat uptake as well as in surface and atmospheric temperatures. However, even if they simulate multidecadal internal variability well (including, implicitly, in forcing as well as in ocean–atmosphere heat interchange), they cannot be expected to match its actual phasing. Therefore, estimates of ECS and/or TCR using temperature observations over the instrumental period are likely to be biased up or down if they span a period over which multidecadal internal variability (in particular, the AMO) has a significant positive or negative influence.
GCM ensembles can certainly be used as a surrogate for uncertainty in forcing, as was done in Otto et al (2013). But I think using the AR5 uncertainty distributions for forcings, now that they are available, is much preferable.
Cloud feedback
You query what negative feedback I envision that might not be included in any model physics. I do not go along with attempts to reverse the burden of proof. When the results of a model that is known to be imperfect disagree with observational evidence, the scientific default position is that the model needs to be modified. If the modellers claim that their model is correct and observational evidence is at fault then it is incumbent on them to prove so. It is not up to someone who accepts the observational evidence that the model is not a good representation of the real world to show where and how it misrepresents the real world.
You say that “On clouds, you seem to ignore the vast body of work aimed at bridging the scale separation between GCM and microphysical scales.” Such work is indeed valuable. However, the multi-authored Zhang et al (2013) paper giving results for low cloud feedbacks from the first phase of the international CGILS project, whilst interesting, inter alia concluded that “the relevance of CGILS results to cloud feedbacks in GCMs and in real-world climate changes is not clear yet. In a preliminary comparison to cloud feedbacks in four GCMs at the three locations, SCMs [single column models] results were uncorrelated to those simulated by the parent GCM”. If ultimately it proves to be the case that cloud feedback is positive rather than negative, then so be it. But there is a long way to go before cloud feedbacks are fully understood and correctly represented in GCMs. Additionally, as Figure 3 of my guest blog showed, GCMs have severe biases as to cloud extent.
It is remarkable how much GCM modelling of water vapour and cloud water content varies, particularly in the upper troposphere (UT). Per Jiang et al (2012), the modelled mean CWCs [cloud water contents] over tropical oceans range from ~0.03x to ~15x the observations in the UT and from 0.4x to 2x the observations in the lower/mid-troposphere (L/MT). Modelled water vapour over tropical oceans was within 10% of the observations in the L/MT, but mean values ranged from ~0.01x to 2x the observations in the UT. Moreover, Figure 6 of Jiang et al (2012) shows that all CMIP5 models analysed have specific humidity levels above the observational uncertainty range at pressure levels between ~150 hPa and ~250 hPa in mid (30°-60°) and high latitudes, as do all but HadGEM2, CNRM-CM5 and one other model in the tropics. For global cloud water content, many models simulate a level outside the observational uncertainty range above ~200 hPa, whilst NCAR-CAM5 is at or below the observational lower bound almost everywhere save near the surface – well below it between ~350 hPa and ~600 hPa.
Su et al (2014)
You mention the recent Su study. I read this when it came out, and thought it quite interesting. However, it seems odd that its metrics based on relative humidity profile in the tropics and sub-tropics rank the sensitive UK Met Office’s HadGEM2-ES model poorly. In the Jiang et al (2012) study, that model’s overall performance ranking was second only to the low sensitivity NCC NorESM model. A contact of mine at the UK Met Office who is knowledgeable about this area was unconvinced by the Su paper.
In Figure 10 of Su et al (2014), six high sensitivity models were placed within or adjacent to the boxes favoured by observational evidence in both performance metrics. For one of these models (NCAR CAM5) I do not have historical/RCP4.5 global temperature time series. The other five models (CSIRO Mk3.6, CanESM2, GFDL-CM3, MIROC-ESM and MPI-ESM-LR) simulated global warming over 1979-2013 with trends in the range 0.19–0.39°C/decade, averaging 0.295°C/decade. That is nearly double the observed HadCRUT4 trend of 0.155°C/decade. Moreover, 1979-2013 was a period over which the AMO exhibited a considerable warming influence on global temperature (Zhou & Tung, 2013), and uncertainty in the change in aerosol forcing was relatively small. However well they score on Su’s performance metrics, these high sensitivity models have badly failed the acid test of simulating global warming over a 35 year period.
References
Jiang, J. H., et al., 2012. Evaluation of cloud and water vapor simulations in CMIP5 climate models using NASA “A-Train” satellite observations, J. Geophys. Res., 117, D14105, doi:10.1029/2011JD017237.
Otto, A., F. E. L. Otto, O. Boucher, J. Church, G. Hegerl, P. M. Forster, N. P. Gillett, J. Gregory, G. C. Johnson, R. Knutti, N. Lewis, U. Lohmann, J. Marotzke, G. Myhre, D. Shindell, B Stevens and M. R. Allen, 2013: Energy budget constraints on climate response. Nature Geoscience, 6, 415–416.
Schwartz, Stephen E., 2012. Determination of Earth’s transient and equilibrium climate sensitivities from observations over the twentieth century: strong dependence on assumed forcing. Surveys in geophysics 33.3-4: 745-777.
Su, H., J. H. Jiang, C. Zhai, T. J. Shen, J. D. Neelin, G. L. Stephens, and Y. L. Yung (2014),Weakening and strengthening structures in the Hadley Circulation change under global warming and implications for cloud response and climate sensitivity, J. Geophys. Res. Atmos., 119, doi:10.1002/2014JD021642.
Zhang, M., et al. “CGILS: Results from the first phase of an international project to understand the physical mechanisms of low cloud feedbacks in single column models.” Journal of Advances in Modeling Earth Systems 5.4 (2013): 826-842.
Zhou, Jiansong, and Ka-Kit Tung. “Deducing Multidecadal Anthropogenic Global Warming Trends Using Multiple Regression Analysis.” Journal of the Atmospheric Sciences 70.1 (2013).
Just a quick post to address Nic’s query as to whether I see a need to reject the approach of Schwartz 2012. It is my view that the degree of coherence one should expect between forcing and temperature depends both on the nature of the forcing and the timescale over which the two are compared. Clearly on very long timescales (a century and longer) one would expect fairly good coherence. On shorter timescales, the expectation is that the coherence would degrade considerably due to internal variability. On decadal timescales, we find in GCM simulations that variability in global mean temperature arising from forcing can easily be swamped by internal variability. Variance on this and shorter timescales is likely to be key to the criterion you use for rejecting the MIROC forcing dataset used in Steve’s paper. So my answer is no, I see no need to reject the approach of Schwartz (which is centered primarily on lower frequency variability) while having misgivings regarding your approach for rejecting Steve’s uncertainty range. I also agree wholeheartedly with your statement that ” There are also substantial uncertainties in forcing, especially aerosol forcing”. I believe this is point is fundamental to Steve’s uncertainty range.
I’d like to respond to the most recent public comments, by Paul S and Fred Moolten, which raise some good points.
Dealing first with Paul’s comments, I agree that AR5 does not imply aerosol forcing levels moderately different from 0.9 W/m² are much less likely than that level. I view the probability density function (PDF) given for aerosol forcing as intended to represent the uncertainty, with ‘low confidence’ being reflected in the very wide PDF, in accordance with the Bayesian probabilistic approach used. Figure 8.16 of AR5 shows that the aerosol forcing PDF level remains above 70% of its peak value from 1.2 W/m² to 0.3 W/m².
On the basis of the AR5 uncertainty distribution, one can’t rule out any of the CMIP5 models’ aerosol forcings as definitely too high – nor as too low even for models that only include direct aerosol forcing. However, it should be borne in mind that the AR5 aerosol estimate, particularly its long negative tail, is influenced by aerosol forcing in CMIP5 and other models. The means for the model and satellite-based estimates used in formulating AR5’s aerosol uncertainty range were respectively 1.28 and 0.78 W/m².
The ‘likely’ (17-83%) ranges of circa 1.2–3.0°C for effective climate sensitivity and 1.0–2.0°C for TCR that I gave in my guest blog reflect, inter alia, the full AR5 uncertainty distributions for aerosol and other forcings.
Regarding Paul’s point about the aerosol forcing reference point for non-ACCMIP CMIP5 models, I have been unable to locate aerosol forcing estimates for most such models. Perhaps Paul can point to where estimates using the method he describes are to be found for the relevant models? For all the CMIP5 models included in my chart showing Historical warming vs Aerosol ERF, I believe the ACCMIP protocol with 1850 as the reference was used.
Paul’s point about GISS-E2-R is a good one, and rather worrying. I would have expected GISS to use the same version (1?) for ACCMIP as was used for the primary CMIP5 results, but he may well be right about it being version 2. Certainly, warming of 0.7°C in the Historical run would bring that model much closer to a best-fit line in my chart showing Historical warming vs Aerosol ERF.
Turning to Fred’s comments, I agree that the acronym ECS is, confusingly, used (in AR5 as well as by myself and others) to cover a range of meanings. IMO, the ECS concept is of most relevance for how global warming will develop over the next century or two. Projecting such warming requires incorporation of the ocean’s behaviour over that period, but not that of ice sheets and other slow components of the climate system. Apart from not being concerned with multicentennial behaviour, that corresponds to the IPCC definition of equilibrium climate sensitivity, which does not represent full equilibrium. As Fred says, there are a range of different timescales depending on the feedback. But atmospheric feedbacks are fast and the ocean can be modelled.
Although a few studies have emphasised evolution in the climate feedback parameter λ over multidecadal or even centennial timescales, the Gregory plots in Andrews et al (2012) show that, where CMIP5 models exhibit a nonlinear relationship between surface temperature change and radiative imbalance after a step quadrupling of CO₂, they mainly do so only over the first few years of the 150 year simulation. That suggests to me that what is involved is more likely to do with non-surface-temperature-dependent atmospheric etc. adjustments to CO₂ forcing, as discussed in Williams, Ingram and Gregory (2008): Time variation of Effective Climate Sensitivity in GCMs and/or to other relatively fast processes.
Whilst effective climate sensitivity is an imperfect measure, I don’t think there is reliable evidence that using it will lead to estimates for global warming over the rest of this century, and probably next century, that are materially inaccurate. Inaccuracy in estimating carbon cycle feedbacks is of much greater significance in projecting warming from different emission pathways. In the RCP8.5 scenario, carbon cycle feedbacks add about 60% to the increase in atmospheric CO2 concentration over 2012-2100. But it is unclear that there is much observational evidence for any carbon cycle feedback at all: Gloor et al 2010. What can be learned about carbon cycle climate feedbacks from the CO2 airborne fraction.
Dear Nic, John and James,
My summary of some key aspects in the discussion so far is that you (or at least Nic and John) seem to agree that the uncertainty in direct and indirect negative aerosol forcing is of crucial importance in the determination of ECS and its uncertainty range from either instrumental observations or GCMs. For Nic it is obvious that observational studies should be valued higher than studies based on climate-models, since there are major problems in models, in particular related to clouds and the simulated warming in past 35 years.
John argues that both approaches have their strength and weaknesses and that there is no strong indication to value one approach above the other. In fact, John is currently working on a study to test Nic’s method using model output from a GCM with a known sensitivity. Also, there is clear scientific understanding why models simulated too high warming rates, especially in the past 15 to 20 years. With respect to clouds, there is a vast body of work pointing at a positive cloud feedback and there is no evidence it might be (strongly) negative.
The study of Schwartz (2012) is cited often, both in your guest blogs and in the discussion so far. A crucial aspect is whether the MIROC database should be part of the analysis since it has a strong influence on the upper part of the derived ECS uncertainty-range. I understand the line of reasoning of Nic to reject MIROC, but it immediately raises the question why Schwartz decided otherwise (and I could not find it in his paper). John writes in his last response that “Variance on this and shorter timescales is likely to be key to the criterion you use for rejecting the MIROC forcing dataset used in Steve’s paper.” John, could you say something more why you think so, because (looking at figure 8) the MIROC database spans the period from 1900 to 1998, shorter than the other databases used in Schwartz (2012) but still almost one century.
After that, I would like to switch to another line of evidence: Paleo Climate.
Bart.
I thank Bart for his summary and will just offer a few clarifications.
On the observations vs models point, I certainly think it desirable to minimise dependence on complex numerical models so far as possible, although it is often necessary to rely on them to some extent. In science, observations determine to what extent models are valid, not vice versa. But there is a problem in that observations are very incomplete, span a limited time and are affected by internal variability. So up to now it has been difficult to rule out many of the possible models of climate behaviour
John’s and my view as to the ‘clear scientific understanding’ of why (CMIP5) models simulated too high warming rates, especially in the last 15 to 20 years are quite different. IMO, the correct scientific understanding is that the models have too high a transient climate sensitivity – and, since the Earth’s energy imbalance seems to be relatively modest – too high an ECS (here meaning effective climate sensitivity). The idea that the ‘vast body of work pointing at a positive cloud feedback’ is based on solid scientific observational evidence is contradicted by AR5’s conclusion about Observational Constraints on Global Cloud Feedback, that “there is no evidence of a robust link between any of the noted observables and the global feedback”.
There is also now a suggestion from experiments with fixed sea surface temperatures that part of what had previously been interpreted in models as positive cloud feedback to surface temperature changes is in fact a rapid atmospheric and land surface adjustment, probably better seen as part of effective radiative forcing. For instance, according to Vial et al (2013) the NCAR CCSM4 model actually shows negative overall cloud feedback, whilst on average across the models analysed cloud feedback, whilst positive, is fairly small – similar to albedo feedback. On the other hand, CCSM4 shows a large positive cloud adjustment, as (to a lesser extent on average) do most models analysed.
Turning to the Schwartz (2012) paper, I don’t think one should place too much emphasis on its precise results and whether or not a particular forcing dataset tells us much about uncertainty in climate sensitivity. To my mind, the take-home message from the paper is this. In general the relationship between forcing estimates and the global instrumental temperature record, along with estimates of heat uptake over the last fifty years, points to TCR and ECS being relatively low. However, there is substantial uncertainty in forcing estimates, related mainly to anthropogenic aerosols. As it concludes: “Confident determination of Earth’s climate sensitivities thus remains hostage to accurate determination of these forcings.” I agree. The observational evidence from warming over the instrumental period certainly points to sensitivity being more likely to be low than high, but one cannot at present conclude for definite that it is low.
Reference
Vial J, J-L Dufresne and S Bony, 2013. On the interpretation of inter-model spread in CMIP5 climate sensitivity estimates. Climate Dynamics, DOI 10.1007/s00382-013-1725-9.
My previous comment relates to Schwartz 2012’s Fig 10 and I’ve attached it below. Here he explores the linear proportionality between observed temperature change since the late 19th century and forcing. The various R^2 noted by Nic are based on two different least squared fits to the data. The colors of the data plotted (large circles) pertain to the year of the data while the colors of the text within the graph relates to the two different fitting procedures (whether it is constrained to cross 0,0 or not). You can see that the degree of correlation relates strongly to the degree to which temperature and forcing follow each other from year to year. The point I’m making is that it is unclear how tightly F and T should track each other on this timescale in nature due to internal variability. It is clear that a priori no strong constraint exists. I am therefore reluctant to exclude a given forcing dataset based on the criterion suggested by Nic. It is a point that Steve himself seems to agree with. He has offered the following quote below for this discussion.
From Steve Schwartz: “The exclusion of the data sets from my further analysis was based on the fact that they did not fit the model relating forcing and observation, but I would not use even that to exclude such forcing histories from the realm of possibility; we need to evaluate forcing independently from its implications on response. Try to maintain a firewall. Otherwise it becomes circular reasoning.”
I agree with John that it is unclear how well F and T in Figure 10 of Schwartz (2012) should track each other on a timescale of one – or even a few – years, given internal variability. However, a regression best-fit line is determined more by the average values of points towards its ends than by values for individual years, particularly those whose points fall towards the middle of the data. Carrying out the regression on points representing averages of several years’ data is unlikely to change the best fit much. The worrying thing about the regressions for the MIROC data set is that the unconstrained best fit based on 1965-1998 data does not pass close to the origin, which it should do under the energy balance model underlying the paper.
I agree with Steve Schwartz that excluding forcing data sets on the basis that they do not fit the model used is not ideal. But I think that using just a selection of mainly GCM-derived data sets is more of a problem. I understand why Steve did so: at the time there were only a limited number of forcing data sets available. Even using forcing time series diagnosed from a much larger ensemble of CMIP5 models does not provide as wide, or as scientifically justified, a spread of possible forcing histories as the climate scientists involved in AR5 decided on, based on uncertainties for each individual forcing. Hence my preference for using the AR5 forcing data set, now that it is available, and its associated uncertainty distributions. For most climate variables, the idea that the CMIP5 ensemble provides a realistic uncertainty distribution is highly questionable.
FWIW, I believe Steve Schwartz’s views on the most likely levels of ECS and TCR are much closer to mine than to John’s.
Dear Nic and John,
Thanks for your clarifications concerning Schwartz (2012). I think it is clear now why according to John one cannot simply reject the overall conclusion of this paper and why there is a risk for circular reasoning. Nic emphasizes that irrespective of Schwartz the relationship between forcing estimates and the global instrumental temperature record in general, along with estimates of heat uptake over the last fifty years, points to TCR and ECS being relatively low.
This last remark of Nic brings us back to the overall quality-issue of the instrumental approach as promoted by Nic. In his report ‘A sensitive matter’, Nic writes:
“As energy budget estimates of ECS are directly grounded in basic physics and involve limited additional assumptions, unlike those from all other methods (including AOGCMs), they are particularly robust. The method does, however, rely on the use of reliable and reasonably well-constrained estimates of:
• changes in global mean total forcing
• TOA radiative imbalance (or its counterpart, climate system – very largely ocean – heat uptake)
• global mean temperature.
But providing that this is done, there seems little doubt that this approach should provide the most robust ECS estimates. Energy budget estimates in effect represent a gold standard.”
So Nic assumes that the estimates needed for his energy budget approach are ‘reliable and reasonably well-constrained’. Reading the discussions on other blog-sites (such as on climate-lab-book last March or on ‘and then there is physics‘) there is quite some discussion to what extent this is actually true. For example, in a recent extensive review study on observations of ocean temperature and heat content (Abraham et. al, 2013) it is concluded that:
“…estimates of Ocean Heat Content (OHC) trends above 700m from 2005 to 2012 range from 0.2 to 0.4W/m2, with large, overlapping uncertainties, highlighting the remaining issues of adequately dealing with missing data in space and time and how OHC is mapped, in addition to remediating instrumental biases, quality control, and other sensitivities.”
To me, this does not sound like ‘reasonably well-constrained’ and should result in much larger uncertainty ranges in ECS and TCR than suggested by Nic.
It would be interesting to hear your thoughts on this, also from James.
Bart,
It’s important to note that your quote refers to 2005-2012, a rather short period of limited relevance to determining ECS/TCR. I’m sure there will always be some debate over how confident we can be of the observed values, but on the other hand it is also clear that the observations (or to be more precise, these particular sets of observations when analysed though energy-balance modelling) do point towards the lowish end of the commonly quoted range.
Bart,
Observational uncertainty in changes in Ocean Heat Content, whilst considerable, contributes far less to the total uncertainty in energy budget estimates of ECS than does uncertainty in forcing, in particular aerosol forcing. And it does not affect such estimates of TCR at all, as they only involve changes in global surface temperature and forcing.
I have made careful estimates of the uncertainties in changes in global surface temperature, forcing and total heat uptake implied by the uncertainty ranges given in AR5, with allowance for internal variability added. Energy budget estimates for ECS and TCR derived from them actually give smaller uncertainty ranges, with lower upper bounds, than those I put forward in my guest blog here and in the ‘A Sensitive Matter’ report, not larger ones.
The idea that observational uncertainties are so large as to preclude useful estimation of ECS and/or TCR is a myth. Some of those in the climate modelling community may wish it were true, as observations increasingly show that high sensitivity models are simulating unrealistically fast warming. 🙂
Apologies but I have been on travel and busy with end of term, so did not follow the blog after posting nearly a month ago. I would like to respond briefly to Nic’s main replies to my previous comment. Nic’s comments are very thorough and show that he has lots of time to devote to this. I am not so lucky and probably won’t have time to continue the dialogue beyond this post.
Nic claims that I am only “half right” in asserting that his method relies on the inter hemispheric difference in warming to tease out the aerosol and GHG/feedback signals. But how else can it work? If it uses some other fingerprint, please explain. In any case the method only works if the fingerprint is known correctly. The assumption that aerosol forcing is concentrated in the northern hemisphere, for example, could prove to be quite untrue (see recent paper in Science by Ilan Koren et al., which implies that cloud-mediated effects could actually have been stronger in the southern hemisphere because there is so much less background aerosol). If the method relies on some other, more complicated fingerprint then it is even more uncertain. I think Nic needs to be more forthright about what fingerprints are actually being used and what the results would be if others were used.
He also notes that if natural variability on decadal time scales (as seems to be the case) greater in reality than in most AOGCMs, this broadens the PDF but does not shift the best estimate. This is true if you have no information on what natural variations actually happened in recent decades. But we do have information on them, and as I explained, they have characteristics that will interact with the implicit aerosol assumptions to bias the result towards lower ECS and TCR. One commenter pointed out the recent paper by Matt England et al, which is also highly relevant here although not about the inter hemispheric warming difference. Nic’s statement that his results aren’t so sensitive to time period misses the point – recent asymmetric warming trends are strong enough to stand out no matter what time period is used, so his insensitivity to time period is just what I’d expect. Moreover, broadening of the pdf is not inconsequential as it reveals that the instrumental record is not a very good constraint on ECS.
He has also twisted the conclusions of AR5 Chapter 7 (of which I was a co-author). The multiple lines of evidence were not only based on GCMs, please read the chapter. We explicitly required observational evidence or back-up from detailed cloud simulations. The two feedback mechanisms we identified as having such support, are both positive (relating to the rise of the tropopause and the poleward shifting of cloud bands) have support both from observations and explicit models of the relevant processes. And as Andy Dessler points out in a comment, to get ECS < 2C you need very strong negative cloud feedbacks to come from somewhere in order to cancel out the known positive ones. We have no evidence for such a thing after decades of searching. The quote from our chapter given by Nic was taken out of context and does not imply there is no evidence for positive feedback. It applied only to one particular strategy that has been used. And in my view the statement would not even be true, due to advances made since AR5 went to press, which further support a climate sensitivity consistent with a stronger positive cloud feedback.
Finally, Nic challenges me to defend the studies he wishes to dismiss. All I can say is that one could dismiss every single study, including his, by cherry-picking some random imperfection in the methods or models used. These studies all passed peer review, which does not prove they are valid, but means that if Nic wishes to dismiss them the burden is on him to identify the key flaw and explain why it would have led to an overestimate of ECS rather than an underestimate.
As a round off on the subject of aerosols, I would like to add the following to my previous summary (also based on personal communication with Nic en John):
The uncertainty in aerosol forcing is very large (-0.1 to -1.9 W/m2 according to AR5) and this is the prime determinant of the uncertainty in ECS and TCR, as deduced from the instrumental period using an energy balance model. All participants seem to agree on this.
In Lewis(2013), Nic uses an aerosol forcing which is on the smaller (less negative) side of the abovementioned range of about -0.7 W/m2.
GCM’s which reproduce the observed warming have an aerosol forcing on the larger (more negative) side of the abovementioned range, but also well within this range. For Nic this is one of the reasons to doubt the models ECS and TCR value, whereas John argues that values being well within the uncertainty range should not lead to such a conclusion.
The aerosol forcing isn’t known for all GCM’s (please confirm or reject whether this is the case).
In order to further constrain ECS and TCR from the instrumental period, constraining the aerosol forcing is a necessary condition.
Bart,
I pretty much agree with your additional comments on aerosols, but would like to add a few clarifications.
Your comment on aerosol forcing in Lewis (2013) (adjusted to 1750-2011, as per AR5) does not make clear that the study formed its own observationally-based inverse estimate of aerosol forcing rather than using an external estimate. Surface temperature changes in four equal-area latitude zones were compared with observed changes over each of six decades, rather than using an external forcing estimate. Steven Sherwood’s comment that I need to be more forthright about what fingerprints are actually being used and what the results would be if others were used is wide of the mark. The fingerprint (the spatiotemporal pattern of aerosol forcing) was determined by the Forest, Stone and Sokolov team at MIT, so he should look in the relevant publications by those authors to see what pattern was used. I simply used their 499 sets of 2D climate model simulations; there is no question of trying other fingerprints because no simulations run with different fingerprints are available.
I have only been able to locate aerosol forcing estimates for a dozen or so CMIP5 models. Excluding a few models that do not include aerosol indirect effects (e.g., CCSM4, bcc-csm1-1), the change in total aerosol forcing is typically above the AR5 best estimate, averaging -1.17 W/m² over 1850-2000 for the models analysed in Shindell et al (2013), significantly higher than the -0.74 W/m² best estimate per AR5 over that period.
I wouldn’t say that I doubt model ECS and TCR values directly because of their large aerosol forcing. Rather, I regard their large aerosol forcing as explaining why until the last few decades AOGCMs did not simulate excessive surface warming or ocean heat uptake despite having high ECS and TCR values, but have done so since then. However, their large aerosol forcing may have indirectly led to AOGCMs having high sensitivities, as the model developers chose model variants and tuned them with an eye on matching the historical temperature record.
Regarding constraining ECS and TCR from the instrumental period, it is in theory possible that a period over which there was confidence that aerosol forcing had changed little might enable ECS and TCR to be better constrained even if the change in aerosol forcing since, e.g., 1850 remained poorly constrained. In that connection, there is a general view that aerosol forcing has changed little over the last ~35 years. Unfortunately, that period has probably been strongly (positively) influenced by multidecadal internal variability in the form of the AMO and also has an asymmetrical volcanic forcing profile.
Reference
Shindell, D. T. et al, 2013. Radiative forcing in the ACCMIP historical and future climate simulations. Atmos. Chem. Phys. 13, 2939–2974
This is a response to various points, italicised, in Steven Sherwood’s comment on 23 June.
Nic claims that I am only “half right” in asserting that his method relies on the inter hemispheric difference in warming to tease out the aerosol and GHG/feedback signals. But how else can it work? If it uses some other fingerprint, please explain.
My statement in my 19 May comment that what Steven wrote in his 16 May was “only partly true” (not half right) was made in response to his false claim that “The Forest/Lewis method assumes that aerosol forcing is in the northern hemisphere (establishing the “fingerprint”), so in effect uses the interhemispheric temperature difference to constrain the aerosol forcing.” I gave a detailed explanation on 19 May of why this claim was wrong.
The assumption that aerosol forcing is concentrated in the northern hemisphere, for example, could prove to be quite untrue
In the fairly unlikely event that were the case, all the CMIP5 AOGCMs would also have got the latitudinal distribution of aerosol forcing changes very wrong as well, implying even more serious deficiencies in the behaviour of those models than currently looks likely to be the case.
I think Nic needs to be more forthright about what fingerprints are actually being used and what the results would be if others were used.
See my 25 June comment in response to Bart.
One commenter pointed out the recent paper by Matt England et al, which is also highly relevant here.
Maybe so, but not for the reason Steven thinks. The England paper claims that increased ocean heat uptake, associated with a strengthening of Pacific trade winds since the beginning of this century, accounts for much of the hiatus in global surface temperature since then. The paper shows that all the claimed resulting increase in ocean heat content (OHC) is in the top 300 m. Steven fails to point out that observational estimates of the rate of 0-300 m OHC increase are actually lower after 2000 than before then. According to Lyman & Johnson (2014) the linear trend in total 0-300 m OHC equated to a global rate of only 0.04 W/m² over 2002-11, an order of magnitude less than the 0.39 W/m² over 1992-2001!
But we do have information on them, and as I explained, they [natural variations] have characteristics that will interact with the implicit aerosol assumptions to bias the result towards lower ECS and TCR. … Nic’s statement that his results aren’t so sensitive to time period misses the point – recent asymmetric warming trends are strong enough to stand out no matter what time period is used, so his insensitivity to time period is just what I’d expect.
See my 19 May comment for a detailed explanation of why these claims are quite wrong. The observational temperature trend by latitude over the 1861-1995 period used in the Forest (2006) diagnostics shows no such asymmetry – in fact the northern hemisphere warmed slightly less than the southern. The main index for the AMO, the main source of internal variability that affects the interhemispheric temperature differential, didn’t cross the zero baseline until 1995.
He has also twisted the conclusions of AR5 Chapter 7 (of which I was a co-author). The multiple lines of evidence were not only based on GCMs, please read the chapter.
I answered this in point 3 of my 21 May response to Bart, writing “My concern is with the global level of overall cloud feedback and the observational evidence relating to it. Section 7.2.5.7 of AR5 “Observational constraints on Global Cloud Feedback’ deals with precisely this, discussing various approaches and citing many studies.” I have not twisted the conclusions of Ch.7 of AR5, I have simply given precedence to what it says about observational evidence. Of course almost all GCMs show overall positive cloud feedback – that is why they have high climate sensitivity! I never claimed that Ch.7 does not cite observationally-based evidence for some specific positive cloud feedbacks, just that it concludes – as it does – that robust observational evidence for positive OVERALL cloud feedback is lacking.
And as Andy Dessler points out in a comment, to get ECS < 2C you need very strong negative cloud feedbacks to come from somewhere in order to cancel out the known positive ones. We have no evidence for such a thing after decades of searching.
I would dispute that. Lindzen & Choi (2011) show such evidence, and Spencer & Braswell (2011) show the difficulty in estimating cloud feedbacks. Counterarguments were made in Dessler (2011) but have been challenged. Clearly, the separation of internal cloud fluctuations from feedbacks is difficult and represents an ongoing research problem.
Finally, Nic challenges me to defend the studies he wishes to dismiss. All I can say is that one could dismiss every single study, including his, by cherry-picking some random imperfection in the methods or models used. These studies all passed peer review, which does not prove they are valid, but means that if Nic wishes to dismiss them the burden is on him to identify the key flaw and explain why it would have led to an overestimate of ECS rather than an underestimate.
As I wrote on 19 May: “This is arm waving. I give specific reasons for dismissing each model. If Steven thinks any of them are wrong, I invite him to say so and to explain why.” Steven has failed to do so. Passing peer review means little. I have identified the key flaw in each study and shown why it leads to an overestimate of ECS – it doesn’t look to me as if Steven has even read my critiques of the studies.
A thought for Steven Sherwood
Steven was quoted in the PlanetOz Environmental blog hosted at the Guardian newspaper’s website on 10 March 2014 as saying, in respect of the report A Sensitive Matter by Marcel Crok and myself published on 6 March:
It relies heavily on the estimate by Forster and Gregory, which was an interesting effort but whose methodology has been shown not to work; this study did not cause the IPCC to conclude that sensitivity had to be low, even though both Forster and Gregory were IPCC lead authors and were obviously aware of their own paper.
Steven’s claim is entirely untrue. The report does not rely on an estimate of ECS or TCR by Forster and Gregory. Its best estimate and range for ECS are based on the Ring 2012, Aldrin 2012, Lewis 2013 and Otto 2013 studies and for TCR are based on the Gillett 2013, Otto 2013 and Schwartz 2012 studies. The ECS and TCR estimates are backed up by an energy budget analysis based on AR5 forcing and heat uptake estimates.
In the context of this constructive “dialogue” it would be great if Steven re-evaluated the claim he made at the time.
Another important subject we did not discuss yet is priors.
Nic wrote in his blog:
“Most of the observational instrumental-period warming based ECS estimates cited in AR5 use a ‘Subjective Bayesian’ statistical approach. The starting position of many of them – their prior – is that all climate sensitivities are, over a very wide range, equally likely. In Bayesian terminology, they start from a ‘uniform prior’ in ECS. All [instrumental based] climate sensitivity estimates in the AR4 report were stated to be on a uniform-in-ECS prior basis. So are many cited in AR5.[…] Use of uniform-in-ECS priors biases estimates upwards, usually substantially. When, as is the case for ECS, the parameter involved has a substantially non-linear relationship with the observational data from which it is being estimated, a uniform prior generally prevents the estimate fairly reflecting the data. The largest effect of uniform priors is on the upper uncertainty bounds for ECS, which are greatly inflated.
Instead of uniform-in-ECS priors, some climate sensitivity estimates use ‘expert priors’. These are mainly representations of pre-AR5 ‘consensus’ views of climate sensitivity, which largely reflect estimates of ECS derived from GCMs. Studies using expert priors typically produce ECS estimates that primarily reflect the prior, with the observational data having limited influence.“
According to Nic’s study (Lewis, 2013), a non-informative prior should be used because:
“The non-informative prior prevents more probability than data uncertainty distributions warrant
being assigned to regions where data responds little to parameter changes, producing better constrained PDFs”
In his guest blog James wrote:
“Further issues arise with his methods, though in my opinion these are mostly issues of semantics and interpretation that do not substantially affect the numerical results. (For those who are interested in the details, his use of automatic approach based on Jeffreys prior [which is a non-informative prior] has substantial problems at least in principle, though any reasonable subjective approach will generate similar answers in this case.)”
My interpretation of James’ remark is that the use of a non-informative prior can also cause substantial problems, but do not seriously affect the results as derived by Nic.
The question I would like to discuss is:
What are the pros and cons of informative, non-informative (or Jeffrey’s) and expert priors in the different types of studies (i.e. instrumental based or paleo based)?
I thank Bart for raising the thorny subject of choice of prior for estimating climate sensitivity when a Bayesian statistical approach is used.
Let me start by making two general points.
First, in general, standard frequentist statistical methods such as ordinary least squares (OLS) regression can be interpreted from a Bayesian viewpoint and, when doing so, involve use of a prior that is implicit in the method and data error distributions. That prior is necessarily objective – it emerges from the statistical model involved, not from any subjective choice by the investigator. For instance, when OLS regression is used and Gaussian data error distributions are assumed, uncertainty in the regressor (x) variable being negligible relative to that in the regressee (y) variable, the implicit prior for the regression coefficient (slope) is uniform. That prior is completely noninformative in this case.
Secondly, in many studies climate sensitivity is not the only unknown parameter being estimated. In such cases, where a Bayesian approach is used, a joint likelihood function is derived and multiplied by a joint prior distribution to give a joint estimated posterior PDF, from which marginal PDFs for each parameter of interest are obtained by integrating out the other parameters. The joint prior that gives rise to a marginal PDF for climate sensitivity (or another parameter of interest) that properly reflects the information provided by the data will not necessarily be the product of individual priors for each parameter – it may well be a non-separable function of all the parameters.
James is correct to say that use of Jeffreys’ prior can give rise to substantial problems, although I am satisfied that it has not done so in the cases where I have used it for estimating climate sensitivity. Problems generally do not arise unless there are multiple parameters and marginal posterior parameter PDFs are required, not just a joint PDF for all parameters. It is well known that Jeffreys’ prior often needs modifying when a parameter’s uncertainty is being estimated as well as its central value. An example is simultaneous estimation from a sample of the underlying population mean and standard deviation. But in most studies uncertainty is not estimated simultaneously with climate sensitivity, and this problem tends not to arise. When Jeffreys’ prior is not suitable, the so-called “reference prior” method, developed by Bernardo and Berger, often provides a satisfactory noninformative prior.
An expert prior is a particular type of informative prior – one might say it is an intentionally informative prior that is derived from subjective opinions rather than only from data. Investigators often use uniform priors for climate sensitivity (and other parameters). Uniform priors are typically informative, biasing estimation towards higher sensitivity values and greatly increasing the apparent probability of sensitivity being very high, relative to what the data values and data error assumptions implied. But I do not imagine that reflects a genuine prior belief on the investigators part that sensitivity is high and an intention to reflect that belief in the prior. Rather, I think in reflects ignorance about Bayesian inference and, in some cases, inappropriate advice in the widely-cited Frame et al (2005) paper to use a uniform prior in the parameter that was the target of the estimate, which advice was adopted in AR4 in relation to climate sensitivity.
There are two problems with using expert priors, even assuming that genuine prior information exists as to parameter values and that it is desired to reflect that information rather than (as is usual in scientific studies) for the results given to reflect only the data obtained and used in the experiment involved.
The first problem is that where the data only weakly constrains the parameter, as is the case for climate sensitivity, the results will be strongly influenced, and may even be dominated, by the expert prior used. That appears to be the case for several of the climate sensitivity estimates presented in AR5: Tomassini et al (2007), Olson et al (2012) and Libardoni and Forest (2011/13).
The second problem is more subtle: the posterior PDF resulting from use of an expert prior may not correctly reflect the combined information embodied in that prior and the data used in the study. That is because, if the expert prior distribution is thought of as arising from multiplying a data-likelihood function by a prior that is noninformative for inference from the statistical model involved, that prior is unlikely also to be noninformative for inference from the product of that notional likelihood function and the likelihood function for the study’s actual data.
I would therefore not recommend using any sort of informative prior, expert or otherwise, for climate sensitivity when estimating that parameter. A noninformative (joint) prior should always be used IMO; Jeffreys’ prior is a good one to start with and is likely to be satisfactory for the purpose.
It may well be appropriate to use data-based informative prior, and sometimes expert priors, for parameters that are not of interest and/or that the study does not constrain well. Indeed, in some studies many variables that would often be treated as uncertain data (e.g., the strengths of various forcings) are estimated as unknown parameters, using priors that reflect the uncertainty distributions of current estimates for those variables.
By and large the same considerations apply to paleoclimate as to instrumental period studies. However, as paleo studies generally involving higher uncertainty the importance of using a noninformative prior is greater. If climate sensitivity is the only parameter being estimated in a paleo study and, as with instrumental period warming based studies, fractional (%) uncertainty in forcing changes dominates that in temperature changes, a uniform prior in the climate feedback parameter, the reciprocal of climate sensitivity, will generally be noninformative for estimating that parameter. It follows mathematically that a prior of the form 1/Sensitivity^2 will be noninformative for estimating climate sensitivity.
Whatever prior is used, I recommend comparing the resulting best estimate (the median should be used) and uncertainty ranges with those derived from using a frequentist profile likelihood method. The signed root likelihood ratio (SRLR) method is simplest to apply. Although the confidence intervals the SRLR method gives are generally only approximate and may well be a bit narrow, they provide an excellent check on whether the credible intervals derived from a Bayesian marginal posterior PDF are realistic. And the median estimate from that PDF should, if it realistic, be very close to the maximum of the profile likelihood.
WHY I DO NOT AGREE WITH LEWIS’ CHOICE OF PRIOR DISTRIBUTION (AND A DIALOGUE BETWEEN TWO ALIENS)
Most experts in climate sensitivity think that the “non-informative prior distribution” of climate sensitivity is what Nic Lewis uses, and that it results into a low climate sensitivity. I do not agree. Some time before Nic published his paper, I also published a paper on the non-informative prior distribution of climate sensitivity (Pueyo, S. 2012. Climatic Change 113: 163-179), and my conclusions were very different:
http://www.springerlink.com/content/3p8486p83141k7m8/
Unfortunately, estimates of climate sensitivity are very sensitive to methodological choices. When adopting a given methodology, climatologists are implicitly positioning themselves about issues in which there is no unanimity among the own experts in probability theory. This means that, if we want our estimates to be realistic, we have a difficult challenge ahead, which we cannot address in the usual ways, e.g. by increasing computing power. However, I hope the climatological community ends up addressing this challenge fully, and does it as soon as possible. To help climatologists bypass some hard texts, I once wrote a comic version of my paper on non-informative priors, featuring a dialogue between two aliens named Koku and Toku.
Also, some time ago, motivated by a conversation with Dr. Forest, I “transcribed” another dialogue between Koku and Toku, which sheds light on the difference between Nic’s and my own view of non-informative priors (I strongly recommend reading the comic above before reading this second dialogue; the comic is short):
There is one thing in which Nic, myself and many others agree: in that the uniform prior vastly overestimates climate sensitivity S. However, this does not mean that many estimates in the literature should be overestimates. The overestimation resulting from this prior is so obvious that, in practice, the uniform is assumed only between S=0 and some Smax, and a zero probability is assumed above Smax, with no explicit criterion to choose Smax (discussed in Annan & Hargreaves 2011, Climatic Change 104:423–436). With this correction, it is not so obvious that this method should overestimate sensitivity, but it is obvious that it is inappropriate. The conclusion of my paper was that the non-informative prior of climate sensitivity is proportional to 1/S. In contrast, Nic sustains that the non-informative prior depends on the dataset but that it will often be roughly proportional to 1/S^2 (see his comment 1048). My prior, S^(-1), is midway between the uniform S^0 and Nic’s S^(-2). If using my prior results into a probability distribution f(S), Nic’s will often give a distribution f'(S) proportional to f(S)/S. My conclusions are that Nic’s is not the correct non-informative prior and that, at least for some datasets, it results into a vast underestimation of climate sensitivity.
Let me add that, in fact, my proposal in Pueyo (2012) was not a direct use of 1/S. I proposed a middle way between the non-informative prior (proportional to 1/S) and subjective priors. My proposal was to start from the non-informative prior, and, then, to introduce explicit and well-justifed modifications (e.g. based on physics) before feeding the data. I hope someone tries this.
I thank Salvador Pueyo for commenting about non-informative priors. I am fully aware of Salvador’s 2012 Climatic Change paper. In it he argues that the problems of estimating S and its reciprocal, the climate feedback parameter, are equivalent, and hence their priors should have the same form – implying a uniform-in-log(S) prior, which has the form 1/S. I disagree with this argument: the two problems do not have the same characteristics. Noninformative priors depend on the experiment involved. Therefore, there is no one correct noninformative prior for estimating S, as Salvador implies: it all depends what is measured and on the error/uncertainty distributions involved.
Salvador writes in his second comic: “One of the main differences is that my method follows Edwin T. Jaynes’ criterion (Jaynes is best known for having introduced the maximum entropy principle), while Lewis (like Jewson et al.) follows Harold Jeffreys’ criterion.”
I would certainly follow Jeffrey’s criterion (setting the prior equal to the square root of determinant of the Fisher information matrix) in the simple 0ne-dimensional case considered in Pueyo (2012), where climate sensitivity is the only parameter being estimated. I think it is quite well established that doing so is appropriate when inference about S is to be made purely on the basis of the data being analysed, without assuming any prior knowledge about it. The authoritative textbook Bayesian Theory Bernardo and Smith (1994/2000), in summarising the quest for noninformative priors, states baldly that:
“In one-dimensional continuous regular problems, Jeffreys’ prior is appropriate”
Jeffreys’ prior has the very desirable property (for a physicist or anyone else seeking objective estimation, if not for a Subjective Bayesian) that if the data variables and/or the parameters undergo some smooth monotonic transformation(s) (e.g., by replacing a data variable by its square), the Jeffreys’ prior will change in such a way that the inferred posterior PDF for the (original) parameter remains as it was before the transformation.
I am a fan of Jaynes, but his maximum entropy principle was developed for the finite case. Unfortunately, Jaynes’ attempts to extend it to the continuous parameter case failed save in certain cases (notably where a transformation group exists).
I thank Nic for his answer to my comment. The points he made will be helpful for some basic clarifications.
Nic refers to Bernardo and Smith’s authority to support the methods that he uses to obtain the “non-informative prior” for each dataset. However, Bernardo was careful enough to coin a new expression for what he (and now, Nic) was using: “reference prior”. Even though there is some confusion between both concepts in the statistical literature, they are quite different. The most important difference does not lie in how you calculate each of these “priors”, but in the meaning that you give to them. In the context of climate sensitivity, we might be able to progress more quickly in our discussions if, in his papers and posts, Nic says that he has been using the “reference prior” and that I sought (or that I found, but he does not seem to agree with this) the “non-informative prior”.
A non-informative prior distribution “sensu stricto” plays the original role of any prior distribution in Bayesian theory: it intends to tell how likely different options are (e.g. different values of climate sensitivity) without considering some given data (in the “non-informative” case, without considering any data at all). When you introduce the data, the prior probability distribution is updated and gives rise to the posterior distribution.
The reference distribution does not tell you the same. The reference distribution is a function that you can use in the place of the prior distribution “sensu stricto” when you cannot decide the later. It is intended just as a convention, as something that everybody is supposed to use when they don’t know what to use, so that everybody’s results are comparable (and, since the reference prior has several good statistical properties, you avoid some types of “accident”). This is a practical option when the posterior distribution is strongly constrained by the data. However, this is not the case of climate sensitivity. In the case of sensitivity, small differences in the prior can have a visible impact on the posterior. Since the reference prior cannot be given the strict meaning of a prior probability distribution, what you obtain by updating it cannot either be given the meaning of a posterior probability distribution. In fact, it is meaningless.
That the reference prior is not, strictly speaking, a prior probability distribution, is apparent from the fact that, as Nic emphasizes, it depends on the experiment. The probability that climate sensitivity is large cannot depend on some experiment that I am planning to do to measure it. Otherwise, climate policy would be much easier: rather than reducing emissions, just plan the right experiment to be carried out in a distant future: once you have it in mind, it should be unlikely that global warming will be severe. Well, at least this is what we would think if we interpreted the reference prior as a prior probability distribution “sensu stricto”, but this is not the right interpretation.
The confusion between reference prior and non-informative prior causes two serious problems. We have already seen one: that the final result (the posterior distribution) is given an unwarranted meaning. The second problem is that, as reference priors are different for different experiments, by using them you cannot combine different types of data. This is especially unfortunate in our case, because, without combining different data types (as Annan and Hargreaves 2006 began to do), it will be difficult for the data to constrain the posterior distribution enough to forget our discussions about the prior of choice (also, we will be more vulnerable to possible biases inherent to specific types of data).
In Pueyo (2012) I had already given an alternative: seek the actual non-informative prior based on Jaynes’ logic, and enrich it with well-justified pieces of prior information. Nic says that Jaynes’ approach “failed save in certain cases”, but I don’t know how he decides that it “failed”. However, even if we accepted that neither Jaynes’ nor any other method allow us to determine a true non-informative prior, there would still be something that we could do: to go ahead by putting together increasing amounts and heterogeneity of data up to the point in which the posterior is robust enough to our choice of prior. However, we cannot do this in the framework of reference priors.
Taking all of this into account, I invite Nic to rethink his current approach and his conclusion that climate sensitivity should be so low, and to consider exploring these other approaches.
A general comment regarding “objective probability”.
Nic and Salvador have both discussed so-called “objective” approaches to Bayesian probability. It is important to clearly understand what this means, and its limitations. These “objective” probabilities do not represent some truth about the state of reality. They are merely an (at best) automatic way of converting uncertain information into a probability distribution which has some intuitively appealing mathematical properties. Intuition can be misleading, however, and despite these properties, there is no guarantee that the results will be useful, sensible, or even remotely plausible.
Conveniently, Nic provides a good example of a catastrophic failure of his approach in the example that he explains in some detail on this climate audit blog post. The topic in that case is carbon dating, but the point is a general one. In his example, his “objective” algorithm returns a probability distribution that assigns essentially zero probability to the interval 1200-1300 AD. That is, it asserts with great confidence that the object being dated does not date from that interval even in the case that the object does in fact date from that interval, and despite the observation indicating high likelihood (in the Bayesian sense) over that interval. That is, this result is entirely due to the so-called “objective” prior (“automatic” might be less susceptible to misinterpretation) irrespective of the data obtained.
Now, Nic asserts that any real physicist will agree with his method. If he can show me a scientist from any field who is happy to assert that a false statement concerning physical reality is true, then I’ll show him a poor scientist.
It is clear that, despite many decades of trying, no-one has come up with a universal automatic method that actually generates sensible probabilities in all applications. Moreover, there is nothing in Nic’s approach that provides for any testing of the method, i.e. to identify in which cases it might give useful results, and when it fails abysmally. Indeed, Nic appears to still think that his method presented in the climateaudit post is appropriate, despite it automatically assigning zero probability to the truth in the case that the item under study actually does date from the interval 1200-1300 AD. But I would hope that most readers – and most scientists aiming to understand reality – would agree that assigning zero probability to true events is not a good way to start, irrespective of the appealing mathematical properties of the method used to perform the calculations. Therefore, there seems little purpose is served by debating over which particular mathematical properties are most ideal in abstract situations. The purpose of scientific research is to understand the world as it really is, and the methods can only be evaluated in terms of how they might help or hinder in that endeavour.
James Annan, as I understood you, you focused on CS on the ground that it was “more relevant [than TCR] to stabilisation scenarios and long-term change over perhaps 100-200 years (and beyond)”. However you didn’t challenge the claim made in the Introduction that TCR is the parameter more relevant to policy. Furthermore I don’t believe those who did spend more time on TCR (mainly Nic Lewis among the experts) challenged it either.
So if I may I would like to challenge it here.
On the face of it, it seems quite reasonable to assume that CO2 will be compounding annually at a CAGR of 1% by 2050. Taking that as a lumped value applicable to the century as a whole, this would make estimation of TCR invaluable for forecasting global mean surface temperature in 2100.
But what is the basis for estimates of TCR? Nic rightly focuses on Box 12.2 of AR5, which is where the current report examines this question most closely, along with estimating both equilibrium climate sensitivity and effective climate sensitivity defined as varying inversely with the climate feedback parameter.
I had a very hard time following how the behavior of 20th C global surface temperature could be used to estimate any of those three measures of climate response to CO2. Problem 1 is that CO2 was rising last year at only 0.5%, at 0.25% in 1960, and even less before then. Problem 2 is that CO2 has risen only 43% since the onset of industrial CO2. And Problem 3 is that ocean delay, long recognized as a source of uncertainty, may be an even bigger source of uncertainty than assumed in interpreting historical climate data.
I do not mean to imply that these are inconsequential numbers, quite the contrary in fact, but rather that they invalidate overly naïve extrapolation from the previous century to this one.
A pathologically extreme example of how badly things can go when you neglect changing CAGR of CO2 can be seen in the 2011 paper of Loehle and Scafetta on “Climate Change Attribution”. They analyze climate as a sum of two cycles, a linear “natural warming” trend, and a steeper linear anthropogenic trend. Setting aside the cycles, the trends purport to model rising temperature before and after 1942, rising (in their Model 2) at respectively 0.016 C and 0.082 C per decade, obtained by linear regression against the respective halves of HadCRUT3.
The following argument justifies their attribution of pre-1942 warming to natural causes.
“A key to the analysis is the assumption that anthropogenic forcings become dominant only during the second half of the 20th century with a net forcing of about 1.6 W/m2 since 1950 (e.g., Hegerl et al. [23]; Thompson et al. [24]). This assumption is based on figure 1A in Hansen et al. [25] which shows that before 1970 the effective positive forcing due to a natural plus anthropogenic increase of greenhouse gases is mostly compensated by the aerosol indirect and tropospheric cooling effects. Before about 1950 (although we estimate a more precise date) the climate effect of elevated greenhouse gases was no doubt small (IPCC [2]).”
For reasons I will give below it is not clear to me that the influence of pre-1942 CO2 was so minor, but set that aside for the moment. Their justification for their linear model of post-1942 warming is as follows.
“Note that given a roughly exponential rate of CO2 increase (Loehle [31]) and a logarithmic saturation effect of GHG concentration on forcing, a quasi-linear climatic effect of rising GHG could be expected.”
The relevant passage from [31] is,
“An important question relative to climate change forecasts is the future trajectory of CO2. The Intergovernmental Panel on Climate Change (IPCC, 2007) has used scenarios for extrapolating CO2 levels, with low and high scenarios by 2100 of 730 and 1020 ppmv (or 1051 ppmv from certain earlier scenarios: Govindan et al., 2002), and a central “best estimate” of 836 ppmv. Saying that growth increases at a constant percent per year, which is often how the IPCC discusses CO2 increases and how certain scenarios for GCMs are generated (see Govindan et al., 2002), is equivalent to assuming an exponential model.”
In effect L&S have based their model of 20th century climate on TCR.
So how bad can this get? Well, ln(1 + x) is close to x for x much less than 1, but becomes ln(x) for x much larger than 1. The knee of the transition is at x = 1. Taking preindustrial CO2 to be 1, today we have a CO2 level for which x = (400 – 280)/280 = 0.43, and with business as usual should reach 1 (double preindustrial) around 2050.
So for the 19th and much of the 20th century ln(1 + x) can be taken to be essentially x. Since x is the product of population and per-capita energy consumption we can assume with Hofmann, Butler and Tans, 2009, that up to now anthropogenic CO2 and hence forcing has been growing exponentially. (Actually the CDIAC data show that the CAGR of CO2 emissions for much of the 19th century held steady at 15%, declining to its modern-day value of around 4-6%, but the impact of anthropogenic CO2 was so small in the 19th C that approximating it with modern-day CAGR of CO2 emissions may not make an appreciable difference. When I spoke to Pieter Tans in 2012 about extrapolating their formula to 2100 he thought a lower estimate might be more appropriate, which is consistent with the declining CAGR of emissions between 1850 and now, but estimating peak coal/oil/NG is far from easy, a big uncertainty.)
It follows that CO2 forcing to date has been growing essentially exponentially, not linearly, but that it will gradually switch to linear (or even sublinear) during the present century. Hence extrapolating 20th century global warming to the 21st century and beyond cannot be done on the basis of either a linear or logarithmic response to growing CO2, but must respect the fact that over the current century forcing will be making the transition from one to the other.
The sharp transition at 1942 in Loehle and Scafetta’s model is in this light better understood as the flattening out (as you go from 1950 to 1930) of an exponential curve. Even if aerosol forcing happened to approximately cancel the left half of the exponential, it would be preferable to put an estimate of the aerosol contribution independently of the CO2 forcing. Moreover if the feedbacks are capable of doubling or tripling the no-feedback response then this would entail aerosol forcing driving CO2, raising the possibility of estimating aerosols around 1900 by comparing the difference between the Law Dome estimates of CO2 with the CDIAC’s estimates of CO2 emissions, provided the difference is sufficiently significant.
There is also the matter of any delay in the impact of radiative forcing on surface temperature while the oceans take their time responding to the former (Hansen et al 1985). If forcing grows as exp(t) with time t, any delay d means that temperature actually grows as exp(t – d) = exp(t)exp(-d), introducing a constant factor of exp(-d) into observation-based estimates of climate response. In particular if exp(-d) = ½, as it might well, then failure to take this delay into account will result in underestimating the prevailing climate response by a factor of two. This on its own would entirely account for misreading a sensitivity of 3.6 as 1.8. That’s a huge contribution to uncertainty. If furthermore the delay varies with time (as it may well given the complexities of ocean heat transport) then so does the factor exp(-d), making the uncertainty itself a factor of time. One might hope that d varied if at all very slowly with time, and preferably monotonically, say linearly to a first approximation.
For such reasons I feel that if climate projections are to be based on climate observations, a third notion of climate response is needed, one that differs from TCR along the above lines, taking into account both the manner in which CO2 grows and the extent to which the ocean delays the response of global mean surface temperature (in degrees) to forcing (in W/m2).
[hope this ends up in the off-topic comments…]
wiljan, the concept of back pressure exists any time there is resistance to a flow from high pressure to low pressure, whether the pressure be radiation pressure, air pressure, voltage, whatever. It is the high pressure end that experiences the back pressure, reducing the flow by reducing the pressure gradient at that end. The notion of back pressure entails no contradiction to the relevant laws, whether applied to the flow of photons, air molecules, electrons, cars driving into a bottleneck, or people walking into a store.
James claims that my Climate Audit blog post about radiocarbon dating provides a “catastrophic failure” of the use of noninformative prior. That is because of the stress he places on probability density and the way he interprets low values of a (posterior) probability density function (PDF). I find it more useful to see how realistic the uncertainty ranges produced by a PDF are than what its values are in particular regions. Moreover, I think one should look at the likelihood function (and/or the prior) as well as the PDF, in order to understand where the likelihood is very low, implying the data is inconsistent with the parameter value, and where only the prior is low (implying, for a noninformative prior, simply that the data is uninformative about the parameter value).
Primary scientific results are normally stated in terms of a best estimate and an uncertainty range, with any PDF underlying the best estimate and uncertainty range being secondary. When a frequentist statistical method is used – as it is in a large proportion of cases – the uncertainty range is usually designed to be a confidence interval whose boundaries the true value of the (fixed but uncertain) parameter involved would fall below in the specified proportions of cases (e.g., 5% and 95%) upon repeating the experiment many times using random drawings from the data etc. uncertainty distributions involved. The method may or may not accurately achieve that aim (known as probability matching), but in most cases there is little disagreement of its desirability. When the IPCC AR5 scientific report states that it is 95% certain that more than half the global warming over 1951– 2010 was anthropogenic in origin, that is based on a frequentist confidence interval, not derived from a Bayesian PDF. If most scientists were told that a archaeological artefact had been shown by radiocarbon dating to be at least 3000 years old with 95% certainty, I think they also would expect the statement to reflect a confidence interval bound, with at least approximately probability matching properties, not a subjective Bayesian PDF.
As I showed in my radiocarbon dating blog post, the use in the case considered of the noninformative Jeffreys’ prior provided uncertainty ranges that in all cases gave exact probability matching no matter what percentage boundaries were specified or from what probability distribution and within what range the sample being dated was picked, unlike whatever method James prefers. Not my idea of failure!
James considers that a near zero probability density over 1200-1300 AD – a calendar period over which the radiocarbon age hardly changes, so that the data is very uninformative about the calendar age – is unrealistic. I suggest that view can only come from prior knowledge of the probability characteristics of calendar ages of samples. The method I was criticising was put forward in a paper that explicitly assumed that no prior knowledge about the probability characteristics of calendar ages of samples existed. But even if some such knowledge does exist, it does not follow that incorporating such knowledge into calendar age estimation (by multiplying an estimated PDF reflecting it, used as an informative prior, by the data likelihood function in an application of Bayes’ theorem) will improve results, even if the PDFs look more believable. As my Climate Audit post showed, doing so and then drawing samples from a segment of the known true calendar age probability distribution often produced estimated uncertainty ranges with probability matching characteristics that were not just worse than when using Jeffreys’ prior (inevitably, as that gave perfect matching), but substantially worse. It should be noted that although the Jeffreys’ prior will assign low PDF values in a range where likelihood is substantial but the data variable is insensitive to the parameter value, the uncertainty ranges the resulting PDF gives rise to will normally include that range.
It is important to understand the meaning of the very low (not zero) value of the prior, and hence of the posterior PDF, over 1200-1300 AD, or over any other period where the radiocarbon age, whilst consistent with the data in terms of having a significant likelihood, varies little with calendar age. It simply reflects that over the interval concerned the data is very uninformative about the parameter of interest, because the interval corresponds to a small fraction of the data error distribution. If some non-radiocarbon data that is sensitive to calendar ages between 1200 and 1300 AD is obtained, then the noninformative prior for inference from the combined data would cease to be low in that region, and the posterior PDF would become substantial in the calendar region consistent with the new data, resulting in a much tighter uncertainty range.
James’ statement that “It is clear that, despite many decades of trying, no-one has come up with a universal automatic method that actually generates sensible probabilities in all applications.” is true. But it masks the fact that in very many cases – probably the vast bulk of practical parameter inference problems – Berger and Bernardo’s reference prior approach does, in many peoples’ view, do so. In the one-dimensional case, Jeffreys’ prior is the reference prior.
James refers to probabilities produced using an objective Bayesian approach as having some “intuitively appealing mathematical properties”. I will single one of these out as a property that the vast bulk of physicists would support. Jeffreys’ prior, and some of the more sophisticated priors that remain noninformative for marginal inference about one parameter out of many in circumstances when Jeffreys’ prior does not do so, are invariant under one-to-one transformations of data and parameter variables. That means, for instance, that if a PDF is estimated for the reciprocal of climate sensitivity, 1/ECS, rather than for ECS itself, and the resulting posterior PDF for 1/ECS is then converted into a PDF for ECS by using the standard transformation-of-variables formula, the PDF thus obtained will be identical to that resulting from estimating ECS directly (from the same data). The construction of the noninformative prior (which will differ greatly in shape between the two cases, when both expressed in terms of ECS) guarantees that this invariance property obtains. A subjective Bayesian approach does not respect it, at least when data variables are transformed.
James’ claims that there is nothing in “Nic’s approach that provides for any testing of the method, i.e. to identify in which cases it might give useful results, and when it fails abysmally.” I beg to differ. I think most statisticians (and scientists) would regard the accuracy of probability matching as a very useful – and widely used – way of identifying when a statistical method gives useful results. There is a large literature on probability-matching priors, and the performance of noninformative priors is often judged by their probability-matching (Kass and Wassermann, 1996). Indeed, Berger and Bernardo (1992) refer to the commonly used safeguard of frequentist evaluation of the performance of noninformative priors in repeated use, as being historically the most effective approach to discriminating among possible noninformative priors.
References
Kass RE, Wasserman L (1996): The Selection of Prior Distributions by Formal Rules. J Amer Stat Ass 91 435:1343-1370
Berger J O and J. M. Bernardo, 1992: On the development of reference priors (with discussion). In: Bernardo J. M., Berger J. O., Dawid A. P., Smith A. F. M. (eds) Bayesian Statistics 4. Oxford University Press, pp 35–60
Dear all, I’ll temporarily take over Bart Strengers’ task of facilitating this discussion.
Thank you Nic, James and Salvador Pueyo for your comments about the influence of the prior distribution on the estimate of ECS.
James and Salvador both mention that what is commonly referred to as an “objective” prior isn’t really objective in the common usage of the word. That is corroborated by the fact that Salvador and Nic come to different conclusions based on what both regard as being an objective prior.
In the exchange between Nic and Salvador (in public comments) both seem to disagree about what’s the most appropriate non-uniform (aka “objective”) prior to use. Salvador claims that what Nic uses is not a truly non-uniform prior but rather the reference prior and is not the optimal choice to make. Salvador uses Jaynes’ non-uniform prior; Nic on the other hand claims that this is not appropriate.
According to Nic, the choice of prior “depends what is measured”. That is criticized by Salvador: If the prior depends on the experiment, it’s not strictly speaking a prior, but rather a reference distribution, which, in the absence of strong constraints by data (as is the case for ECS) causes a meaningless posterior distribution. Question to Nic: Could you reply to this specific criticism? Nic claims that the Jaynes prior is not suited to the “continuous parameter case”. Question to Salvador: Could you reply to this specific criticism?
Both Nic and Salvador come to their choice of prior to prevent the problem that’s common with uniform priors (which both Nic, Salvador and James have criticized, as e.g. quoted in Ch 10 of AR5), namely that the shape of the uniform prior distribution is very different in ECS versus in 1/ECS (the so called sensitivity parameter, lambda) and that a uniform prior in ECS could lead to an overestimate of ECS (though Salvador argues that in practice the uniform prior is only assumed for a certain range of ECS, so not all uniform priors necessarily result in overestimations). Question to John: In light of these criticisms, is the use of uniform priors suitable for the estimation of ECS?
James claims that what Nic uses as a prior can cause erroneous results: In the example as explained in http://julesandjames.blogspot.fi/2014/04/objective-probability-or-automatic.html (a reply to Nic’s post at http://climateaudit.org/2014/04/17/radiocarbon-calibration-and-bayesian-inference/) the posterior pdf shows zero probability density at locations where the data show substantial likelihood but the prior pdf is zero, i.e. the prior pdf prevents the data from being properly reflected in the posterior pdf (“[it] automatically assign[s] zero probability to the truth”). Question to Nic: Could you reply to this specific criticism?
Thank you Bart (Verheggen) for your synthesis and questions. Let me just clarify that most of your mentions of “non-uniform prior” refer, more precisely, to “non-informative prior”.
I am grateful that Bart clearly separates my approach from Nic’s. Quite confusingly, they are often lumped together, e.g. in the recent post by James (perhaps because he posted it while my previous post, in which I emphasize the differences, was awaiting approval). As I said in my previous post, Nic’s would be more properly called “reference prior” than “non-informative prior”, while I did seek a “non-informative prior”. From my point of view, the concepts of “non-informative prior” and “reference prior” differ as much from each other as each of them differs from the concept of “expert prior”.
James and Nic have engaged in a discussion on whether or not it is a “catastrophic failure” that, in a given example (unrelated to climate sensitivity), Nic’s method assigns an almost null probability to the interval in which the only measurement lies, and larger probabilities to neighboring intervals. Behaviors like this occur because “reference priors” like Nic’s can be very complex, which makes clear that they are not “non-informative”. In fact, they contain a lot of information, but it is information on the measurement technique, which is completely unrelated to the original concept of “prior probability” (i.e. the probability to be assigned to the property to be measured before the measurement takes place).
James uses this example to criticize so-called “objective priors” in general, an expression that encompasses both reference priors and non-informative priors, and that he suggests to replace by “automatic priors”. I think my comment above makes clear that his point is valid only for reference priors, and cannot be extrapolated to non-informative priors. Furthermore, I will argue that, in some sense, non-informative priors are indeed “objective”, but are not “automatic” (unlike reference priors).
I will introduce my argument with the help of a thought experiment. Consider a set of objects whose positions have been decided by algorithms that are completely different and unrelated to each other. My intuition tells me that the frequency distribution of the coordinates of these objects will be uniform. Besides intuition, the rational argument to expect a uniform distribution is that it is the only one that preserves the symmetries of the problem. Therefore, the uniform should be the non-informative probability distribution in this case (according to Jaynes’ invariant groups logic), and it is so “objective” that it results into an observable frequency distribution.
I have suggested that there are several frequency distributions in nature that are almost non-informative because they result from putting together values with completely different, “idiosyncratic” origins (see a synthesis of previous papers in http://arxiv.org/abs/1312.3583). Often, the variable of interest is not a position, so the symmetries to be conserved are not the same, and give rise to a distribution that is not uniform. To predict this objective distribution, one needs to identify the symmetries of the problem, which is an objective but not automatic process.
We do not have a sample of “idiosyncratic” climate sensitivities allowing us to observe a frequency distribution, but we can still treat the non-informative distribution similarly to a frequency distribution, as we would treat the result of tossing a coin only once. As soon as we have this prior distribution (log-uniform, according to my results) and some measurements defining a likelihood function, Bayes theorem is very clear in that we have to combine the first with the second to know how likely different values of sensitivity are. The way measurements are taken affects only the likelihood function, not the prior probability distribution.
Nic’s technique does not deal with actual non-informative priors. Whether or not one agrees with my claim that non-informative priors do exist, one has to concede that using a different type of prior (reference prior) as if it were non-informative can cause serious trouble unless the amount of data makes the result quite insensitive to the prior, which is rarely the case with climate sensitivity. As I said, underestimation of climate sensitivity is especially likely when using Nic’s method.
Bart asked me to reply Nic’s claim that Jaynes’ prior is not suited to the “continuous parameter case”. In my previous post, I stated: “Nic says that Jaynes’ approach ‘failed save in certain cases’, but I don’t know how he decides that it ‘failed’”. Unless he clarifies this, I cannot fully answer his criticism. However, I have already given some reasons to think that Jaynes’ logic is valid.
I am responding to Bart (Verheggen)’s 27 July comment and questions.
Bart states that the fact that Salvador Pueyo and I come to different conclusions based on what we both regard as being an objective prior provides corroborative evidence that an objective prior is not objective in the common use of that word. Although in most cases a completely noninformative prior may not exist, so that conclusions will depend to at least a modest extent on a subjective choice of prior, the main reason that Salvador and I come to different conclusions is that we have completely different views on what makes a prior uninformative, resulting in us selecting different priors. One of us must be wrong! (Of course, even where there is a unique fully noninformative prior, parameter estimation involves other subjective choices.)
Bart asks me to respond to Salvador’s criticism that if the prior depends on the experiment, it’s not strictly speaking a prior, but rather a reference distribution, which, in the absence of strong constraints by data (as is the case for ECS) causes a meaningless posterior distribution.
Where a prior is intended to be noninformative, not reflecting any prior knowledge of the parameter(s) being estimated, then it should depend on the experiment and nothing else. It is best regarded as a mathematical tool or weighting function, designed to produce a posterior PDF that reflects the data not the prior. Such a prior has no direct probabilistic interpretation: it should not be regarded as a probability density. A prior that is noninformative in this sense may or may not be a “reference prior” in the Berger and Bernardo’s sense. I think Salvador arguing that such a prior is not actually a prior distribution in the strict sense of representing a genuine probability distribution for the parameter(s). I wouldn’t disagree. But so what? It doesn’t follow that in the absence of strong constraints by data that means the resulting posterior distribution is meaningless. Quite the contrary. And the distinction that Salvador is making between what he calls respectively “reference priors” and “noninformative priors” makes no sense to me.
The whole point about a noninformative prior is that it is constructed so that only weak constraints by the data are required in order for the resulting posterior PDF for the parameter(s) to be dominated by (correctly-reflected) information from the data rather than information from the prior. Indeed, Berger and Bernardo show that reference priors have a minimal influence on inference, in the sense of maximising the missing information about the parameters.
Salvador’s arguments about a uniform distribution being noninformative for positions, and relating to symmetries, may be valid when parameters only have a finite number of possible values, but they fail in the continuous case because the relevant invariant measure is unspecified. Jaynes recognised this point (Section 12.3 of Probability Theory: The Logic of Science), and was only able to resolve the measure problem in special cases, in particular when a transformation group existed.
Bart also asks me to respond to James’ criticism that (in a radiocarbon dating example) the posterior PDF shows zero probability density at locations where the data show substantial likelihood but the prior pdf is zero. My 23 July comment already deals with most of what James said. When Jeffreys’ prior (the original noninformative prior) is used, the prior, and hence the posterior, is very low (not zero) in regions where the data are very uninformative about – change little with – the parameter(s). If no existing knowledge about the parameters is to be incorporated, the resulting PDF is correct, however odd it may look.
Suppose the data measures a variable (here radiocarbon age of an artefact), with known-variance Gaussian-distributed random errors. In the absence of prior knowledge about the artefact’s radiocarbon age or calendar age, use of a uniform prior for inferring the radiocarbon age of the artefact is both natural and noninformative. Use of a uniform prior results in a Gaussian posterior PDF, credible intervals from which exactly match frequentist confidence intervals for the same measurement. What’s not to like about that? But if one accepts that posterior PDF for radiocarbon age, one necessarily accepts the sort of odd-shaped posterior for the artefact’s calendar age that James rejects, since it follows from applying the standard transformation of variables formula.
James prefers what he views as realistic-looking posterior PDFs for parameters, even if the uncertainty ranges they produce disagree substantially with relative frequencies in the long run – and the posterior PDFs they imply for radiocarbon ages are most unrealistic looking.
I on the other hand prefer – , certainly for reporting scientific results – posterior PDFs that produce uncertainty ranges which are at least approximately valid in frequentist coverage (probability matching) terms upon (hypothetically) repeated independent measurements, so that they represent approximate confidence intervals. (Exact matching of confidence intervals is not generally possible using a Bayesian approach.) Of course, if there is genuine prior knowledge about the distribution of an artefact’s calendar age, then the position is different. But I don’t think a wide uniform distribution would convey such knowledge in any case.
Nic states “the main reason that Salvador and I come to different conclusions is that we have completely different views on what makes a prior uninformative, resulting in us selecting different priors. One of us must be wrong! ”. I had previously stated that the “reference priors” used by Nic aren’t “non-informative priors”. However, Nic says that “the distinction that Salvador is making between what he calls respectively ‘reference priors’ and ‘noninformative priors’ makes no sense to me ”. Let’s see the point of view of Bernardo, who introduced reference priors (building on Jeffreys), and is repeatedly cited by Nic as an authority. In his seminal paper on reference priors, this author stated that “a reference prior does not describe a situation of ‘non-information’ about the parameters of a model” (Bernardo 1979a, p. 126). The opinion that the introducer of reference priors has on non-informative priors is best expressed in the title of Bernardo et al. (1997): “Non-informative priors do not exist”. In the ensuing comments, Ghosh (1997) summarizes the position behind this title as a “concern with useful non-subjective posteriors instead of noninformative priors”. Indeed there has been much confusion between reference and non-informative priors in the literature (and even Bernardo has contributed to it at some points), but it is clear to me that this distinction does “make sense”.
I not only made the distinction between reference priors and non-informative priors, but went beyond and stated that “the reference prior cannot be given the strict meaning of a prior probability distribution ”. Nic writes “I think Salvador arguing that such a prior is not actually a prior distribution in the strict sense of representing a genuine probability distribution for the parameter(s). I wouldn’t disagree. But so what? ”. His interpretation is correct, but let me answer to the “so what?”. Bayes theorem establishes a mathematical relationship between probabilities. Therefore, if you pretend to apply Bayes theorem but your input is not a probability distribution, then you cannot use Bayes theorem to state that your output is a probability distribution. You are free to equate this output to a probability distribution, but this results from an extra step: a subjective decision. It is not an objective result.
Then, what is the usefulness of the “reference posterior” that you obtain using a “reference prior”? I had already stated two points: as a conventional way to express the information in your sample, and as a way to avoid some technical problems that some other priors pose in some cases. Bernardo mentions these uses, but actually, he emphasizes another one: it “is just a part – an important part, I believe – of a healthy sensitivity analysis to the prior choice” (Bernardo et al. 1997, p. 163). He means that the result of applying a reference prior is useful because it can be compared with the result of applying your subjective prior of choice, to check if the posterior distribution is sensitive to the prior.
We are having this lively discussion because of the consequences that different choices of prior may have for decisions in climate policy, including Nic’s choice, i.e. a reference prior. If the usefulness of reference priors is limited to the points that I described above, what are the implications of taking the resulting posterior distribution at face value for policy decisions? Bernardo (1979b, p. 140) was admirably honest: “it would certainly be foolish to use it in lieu of the personal posterior which describes the decision-maker opinions”. Of course, this assumes that we can reach well-founded opinions in other ways, probably with a sound expert prior, which is no less problematic in the case of climate sensitivity. So, which other alternatives do we have?
I mentioned two alternatives. For those who, unlike Bernardo and many others, think that non-informative priors do exist, the alternative is clear: using them (corrected with pieces of well-founded knowledge, Pueyo 2012). This is my opinion and I posit that such priors can be found by applying Jaynes’ logic. The problem that Nic sees in this option is that Jaynes admitted being only “able to resolve the measure problem in special cases, in particular when a transformation group existed.” In the case of climate sensitivity, we can consider the transformation group whose elements are changes in measurement units. These changes do not have to affect a non-informative prior. This leads to the result in Pueyo (2012).
The second alternative is accepting a posterior distribution only when it proves mostly insensitive to the prior (so we do not need to decide which prior is the correct one). Probably, we will need to combine different types of data to reach this point. Such combinations are forbidden when using reference priors, but are perfectly correct when assuming that we have a prior probability distribution sensu stricto, either non-informative or informative, whether or not we specify it.
References
Bernardo, J.M. 1979a. Reference posterior distribuitons for Bayesian inference. Journal of the Royal Statistical Society B 41: 113-128.
Bernardo, J.M. 1979b. Author’s reply. Journal of the Royal Statistical Society B 41: 139-147.
Bernardo, J.M., Irony, T.Z. & Singpurwalla, N.D. 1997. Non-informative priors do not exist. A dialogue with José M. Bernardo. Journal of Statistical Planning and Inference 65: 159-177.
Ghosh, J.K. 1997. Non-informative priors do not exist – discussion of a discussion. Journal of Statistical Planning and Inference 65: 180-181.
Pueyo, S. 2012. Solution to the paradox of climate sensitivity. Climatic Change 113: 163-179
Dear John, Nic and James,
I would like to discuss now another important line of evidence: paleo climate. Below I try to summarize the arguments that have been brought up in the guest blogs and in the discussion so far.
James argues that when averaged over a sufficiently long period of time, the earth must be in radiative balance or else it would warm or cool massively. This enables us to use paleoclimatic evidence to estimate ECS. Non-linearities in the temperature response complicate the comparison of paleo-climate to the current changes in climate, but James argues that nevertheless paleoclimate evidence can offer useful constraints to ECS, due to the relatively large changes in temperature and forcing. The evidence rules out both very high and very low sensitivities and provides a figure around the IPCC range which could be used as a prior for Bayesian analyses.
Nic basically rejects paleo climatic approaches based on what is written in the last sentence of paragraph 10.8.2.4 in AR5 where it is concluded that paleo studies support a wide 10–90% range for ECS of 1.0–6°C. Nic points to the fact that in general, AR5 states that the uncertainties in paleo-studies are underestimated because of 1) the difficulty in estimating changes in forcing and temperature and 2) past climate states are very different, that is, may differ from the ECS measuring the climate feed-backs of the Earth system today and therefore widening the uncertainty range (i.e. flattening the PDF) seems reasonable. Nic thinks the uncertainties are simply too great to support the narrower 2–4.5°C range mentioned by James.
John argues that paleo studies benefit from the large climate signals that can occur over millennia and that the paleo record provides a vital perspective for evaluating the slowest climate feedbacks. He emphasizes that sensitivity to nonlinearities, major uncertainty in proxy records (Rohling 2012), data problems, and uncertainty in forcing undermine any strong constraint on ECS and it is unclear whether progress on these fronts presents an immediate opportunity for reducing uncertainty in ECS in the near future.
A general question for all would be to discuss the pros and cons of paleo-estimates of ECS, in light of the arguments brought forward by the others.
Specifically:
James: Could you respond to the issues raised by Nic and to indicate why you think the uncertainties aren’t too great to support the 2 – 4.5 °C range.
John: What range of ECS estimates do you think can be derived from paleo-studies
Nic: AR5 is full of caveats (incl e.g. about Bayesian priors), so why should the caveated language about paleo-estimates of ECS be translated into them being rejected?
Bart V asks me to justify my treatment of paleo-estimates of ECS. (I wouldn’t put any weight on what AR5 said about Bayesian priors, BTW.)
Bart has over-interpreted my position. I don’t exactly reject paleo-estimates of ECS. Rather, I agree with AR5’s caveats, and broadly accept their 1–6°C range, although I would be inclined to treat it as a 17–83% likely range rather than a 10–90% range.
However, AR5 gives little indication of the shape of the uncertainty distribution involved in paleo estimates. My view is that for paleo estimates the fractional uncertainty as to forcing changes and as to the relationship of climate feedbacks in different climate states (which AR5 does highlight) is likely in reality to be considerably greater than fractional uncertainty as to temperature changes. Assuming so, the overall PDF for ECS from paleoclimate studies should have a rather similar skew to that derived from instrumental period warming based studies, implying a median estimate far below the midpoint of the 1–6°C (or whatever) range.
That being so, even if the paleoclimate studies provide a completely independent overall estimate of ECS to that from warming over the instrumental period, the paleo estimate should not greatly affect the overall median and likely range derived from warming over the instrumental period. I’ve done some calculations based on the 1.7°C median estimate and 1.2–3°C likely range I put forward, which corresponds to uncertainty in the climate feedback parameter (the scaled reciprocal of ECS) having a normal distribution. If the overall 1–6°C paleo estimate shares that characteristic, then incorporating it would do little other than narrow my 1.2–3°C likely range at both ends. This is perhaps an extreme case, but it does illustrate my point.
In the past week, Nic, James and John indicated they want to finalize this dialogue on Climate Sensitivity. Although I would be interested in the viewpoints of James and John on the topics raised by Bart Verheggen in his last post, I respect their decision, also because the discussion has been going on for quite a while now.
As a very last question I invited the experts – by e-mail – to give their personal opnion on the relevance of the debate on Climate Sensitivity to climate policy and policy makers.Their answers are given below.
On the subject of the relevance of the scientific debate on climate sensitivity to the political discourse, it is my opinion that the two are largely disconnected, at least here in the US. It is true that those advocating for the status quo often insist that sensitivity is low (or zero), but this is done largely out of convenience rather than being based on specific tidbits of convincing evidence. In fact, the latest shift in political tactic here is to disavow any knowledge of the science altogether (e.g. Marco Rubio’s and Rick Scott’s “I’m not a scientist” remarks). In truth, despite the differences voiced by Nic, James and I during our discussion, our viewpoints represent a relatively narrow portion of the US political spectrum, where half of Congress essentially deems the issue unworthy of further study – an opinion clearly voiced by members with vested interests that would change little if the scientific consensus were to become firmer. Given this reluctance to embrace even the broadly accepted facts on the issue (e.g. that humans have contributed substantially to Earth’s warming since the mid-20th C), any strong connection between the scientific debate and policy seems thus far to be elusive. It is both my hope and expectation that this will change.
The equilibrium climate sensitivity has long been a central question for scientific research in climate change, and is a key (although not sole) determinant for the importance and urgency of CO2 emissions mitigation.
Thus, it is not surprising that it has become a touchstone in the political debate. If the equilibrium sensitivity was around 1C or below, then anthropogenically-forced climate change would be a rather slow process and we would have little committed change. If sensitivity is close to 6C or higher, then we would already have committed the climate system to huge changes and rapid, massive emissions cuts would be an extremely urgent priority. However, a broad range of evidence points to a sensitivity well inside these values (albeit somewhat towards the lower end) and the remaining debate concerning the precision of our estimates is not, or at least rationally should not be, so directly pertinent for policy decisions. We already know with great confidence that human activity is significantly changing the global climate, and will continue to do so as long as emissions continue to be substantial.
I’m not an expert on the politics involved, but I give my personal opinion below:
The political situation in Europe (certainly in the UK) is different from that in the USA. The political centre ground is considerably to the left of that in the US. All the main parties affirm belief both in the science of climate change and the need to take action to reduce carbon emissions. In reality, very few politicians have any real understanding of the science, or of the merits of the costly climate policies that they legislate for. The government politicians in the UK listen to a fairly narrow group of advisers on climate change, and take no notice of the observational evidence that sensitivity may very well be lower than represented in the CMIP5 models. The highbrow media (BBC, Guardian newspaper) are committed believers in dangerous anthropogenic climate change and present only that viewpoint.
There are also various pressure groups warning of dangerous climate change and pushing for strong actions to reduce emissions. These include renewable energy groups and other subsidy farmers with vested interests, environmental NGOs and radical politico-environmental campaigning/protesting groups. So, at present, the scientific debate on climate sensitivity has a very limited impact in the UK political context. However, the few writers and bloggers who put forward the case for climate sensitivity being low, climate scientists and advisers being biased and/or policy being wrong-headed do get some attention, as does the Global Warming Policy Foundation think tank. The longer the hiatus continues, and the more energy prices are pushed up (and consumer choice reduced) by emission reduction policies, the less IMO the public is likely to believe climate scientists and to support policies to combat AGW. The politicians might then listen to a wider range of views within the scientific debate on sensitivity. Or a party with very different views on AGW might get to wield power, as in Australia.
@James: I interpreted Bart’s question to mean what relevance the debate on climate sensitivity actually had in practice in the political context, as I believe John also did, whereas you seem to have taken it to mean more what relevance it should have.
IMO, the debate on climate sensitivity and TCR should still be very pertinent in the political context, even though it currently is not. When allied to the parallel debate on the level of carbon cycle feedbacks, which has barely started, lowish sensitivity/TCR estimates (in line with what AR5 forcing and heat uptake best estimates imply) point to global warming from now to 2081-2100 of little more than 1 K on a business-as-usual scenario.
The CMIP5 mean projected rise is about three times as great. Which is correct has huge implications for what the optimal policy response is.
I don’t think that any politicians in the UK, or for that matter Japan (where I lived and worked until recently) is paying much attention to the arcane debate in the literature about climate sensitivity. There are of course pressure groups who tend to distort the science as an alternative to debating honestly about policy choices, but thankfully they don’t seem to have much influence.